A Semi-Soft Label-Guided Network With Self-Distillation for SAR Inshore Ship Detection.
IEEE Trans. Geosci. Remote. Sens.(2023)
摘要
With the soaring development of deep learning (DL) mechanisms in recent years, convolution neural network (CNN)-based methods have been extensively investigated to achieve high accuracy of ship detection in synthetic aperture radar (SAR) images. However, existing CNN-based SAR ship detection methods still suffer from challenges in complex inshore scenarios due to the strong interference therein. To tackle this issue, a novel semi-soft label-guided network based on self-distillation (SD) for SAR ship detection (S2LSDNet) is proposed in this article. First, different from the existing CNN-based detectors to extract features from the image domain only under the guidance of a one-hot label, an efficient SD training strategy is devised to extract semi-soft label information to boost the inshore ship detection accuracy. Second, an angle-related and balanced intersection-over-union (ArBIoU) loss is developed to enhance the inshore ship positioning performance by using the adaptive weights of center point bias and the aspect ratio difference. Experiments on the open SAR ship detection datasets demonstrate the effectiveness and superiority of the proposed method compared with the existing state-of-the-art approaches, especially in inshore scenes.
更多查看译文
关键词
semi-soft,label-guided,self-distillation
AI 理解论文
溯源树
样例
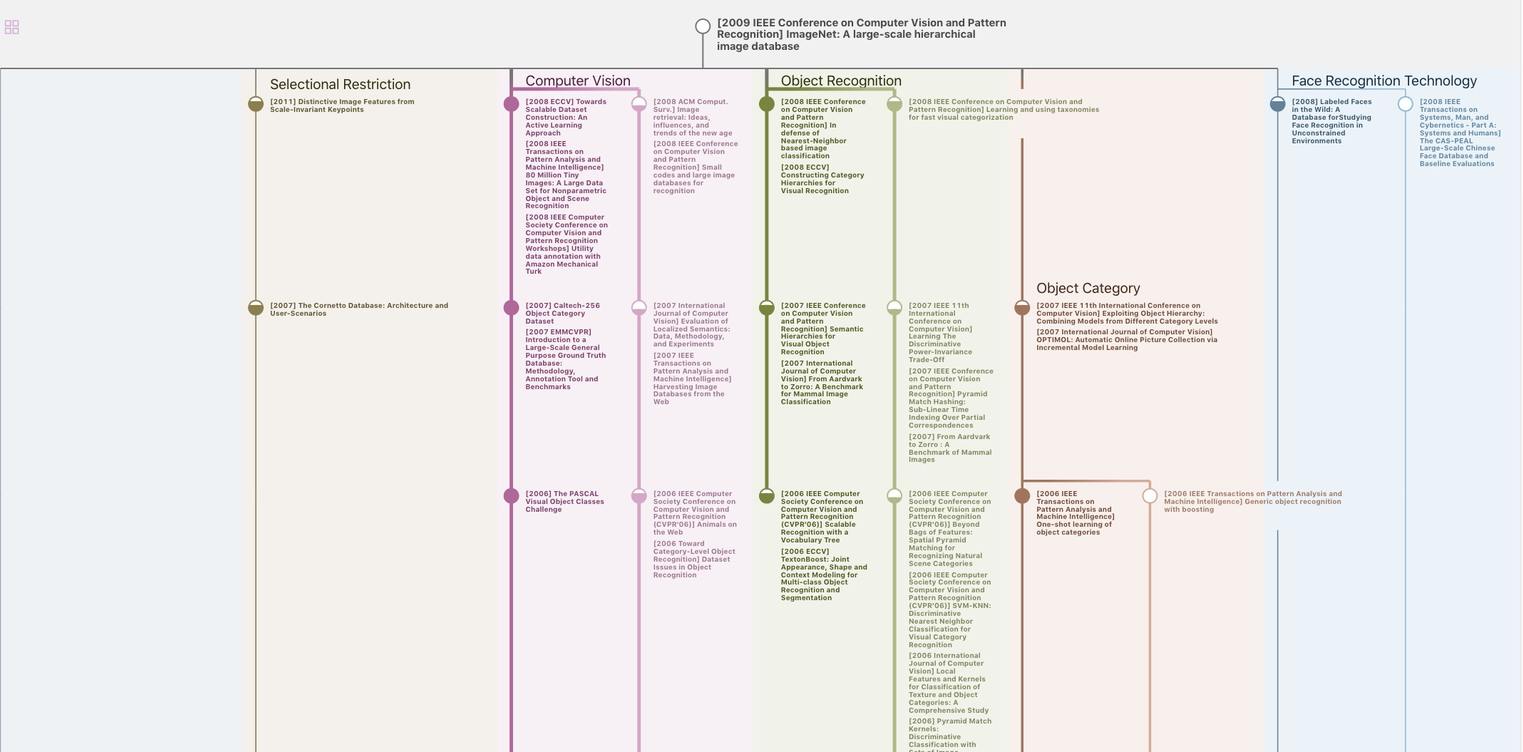
生成溯源树,研究论文发展脉络
Chat Paper
正在生成论文摘要