Hyperspectral Image Denoising Via Texture-Preserved Total Variation Regularizer.
IEEE Trans. Geosci. Remote. Sens.(2023)
摘要
The total variation (TV) regularizer is a widely used technique in image-processing tasks to model an image's local smoothness property. Intrinsically, the TV regularizer imposes sparsity constraints on the gradient maps of the image, which inevitably weakens the image texture structure and thus affects the quality of image restoration. To alleviate this issue, we propose a novel texture-preserved TV (TPTV) regularizer for hyperspectral images (HSIs) by introducing a weighting scheme. Specifically, the weights are assigned to the gradient maps of HSIs, which help slack the sparsity constraint for the pixels with large variations, thus preserving the texture structure. Additionally, we elaborate an empirical method to learn the weights adaptively from observed HSIs. Then, we propose an HSI denoising method based on the TPTV regularizer. Experimental results on synthetic and real HSIs illustrate the superiority of our proposed method over other state-of-the-art methods. In addition, the proposed weighting scheme can be finely embedded into other TV regularizers and protect the image texture. The experimental results also demonstrate that the denoising performance of the original method is significantly improved after embedding the weighting scheme.
更多查看译文
关键词
Hyperspectral image (HSI) denoising, texture-preserved total variation (TPTV), total variation (TV), weighting scheme
AI 理解论文
溯源树
样例
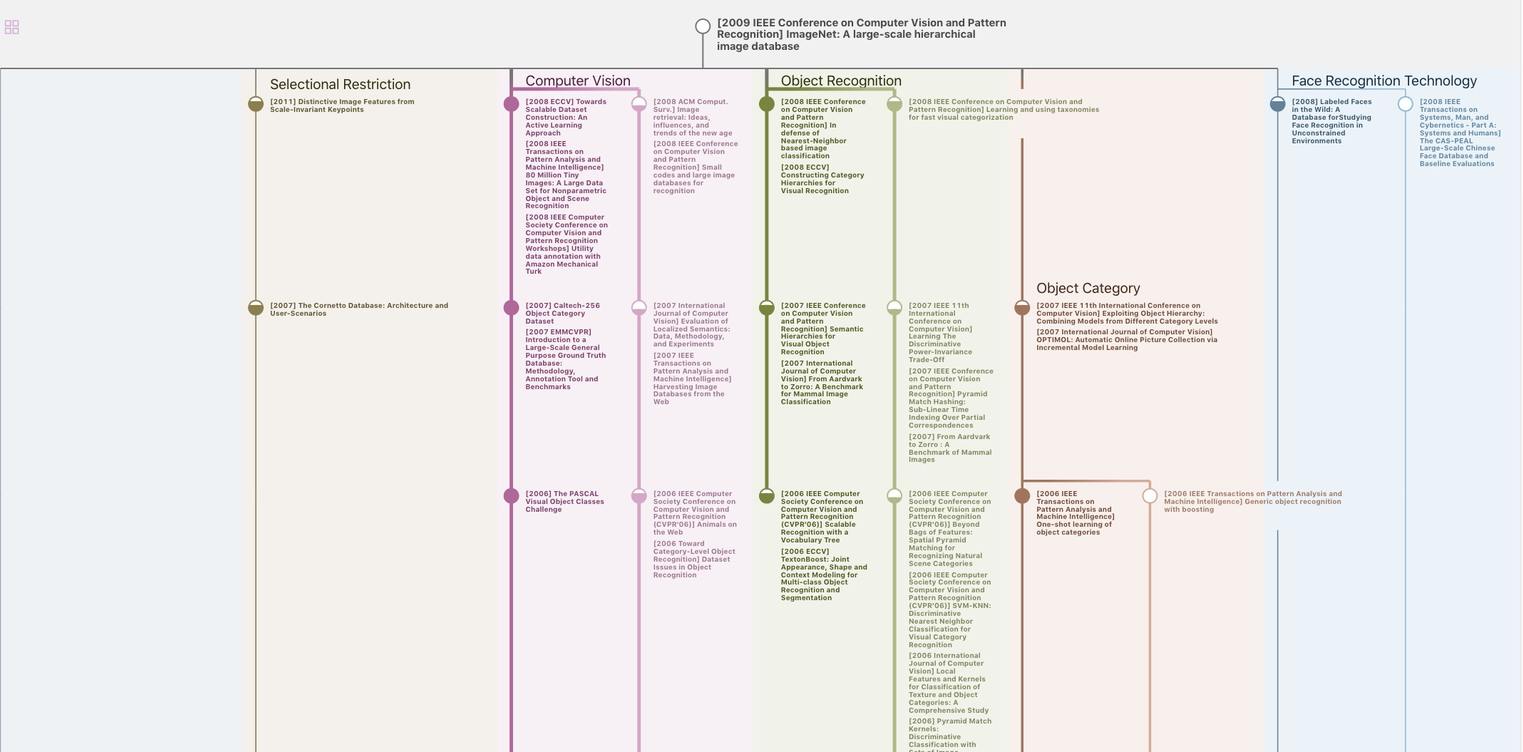
生成溯源树,研究论文发展脉络
Chat Paper
正在生成论文摘要