Dropout Autoencoder Fingerprint Augmentation for Enhanced Wi-Fi FTM-RSS Indoor Localization.
IEEE Commun. Lett.(2023)
摘要
In this letter, we propose a dropout autoencoder fingerprint augmentation approach for enhanced Wi-Fi fine time measurement and received signal strength signals-based indoor localization. Due to complex indoor environment, fingerprinting techniques suffers from unrecorded measurements at some reference points, leading to incomplete fingerprint datasets. The dropout autoencoder was employed to reconstruct clean signal features for the unrecorded fingerprint measurement which can significantly affect the localization accuracy of fingerprinting systems. The localization is accomplished by utilizing deep neural networks (DNN)-based regression. We collected two datasets from experiments conducted in two indoor offices using commercial off-the-shelf devices. The performance of our proposed method was compared to existing methods, and on the respective datasets, our proposal method showed better performance with a localization accuracy of
$\mathrm {0.3~ \text {m}}$
and
$\mathrm {0.6~ \text {m}}$
for the 1-
$\sigma $
percentile errors and
$\mathrm {0.66~ \text {m}}$
and
$\mathrm {1.5~ \text {m}}$
for the 2-
$\sigma $
percentile errors.
更多查看译文
关键词
dropout autoencoder fingerprint augmentation,localization,ftm-rss
AI 理解论文
溯源树
样例
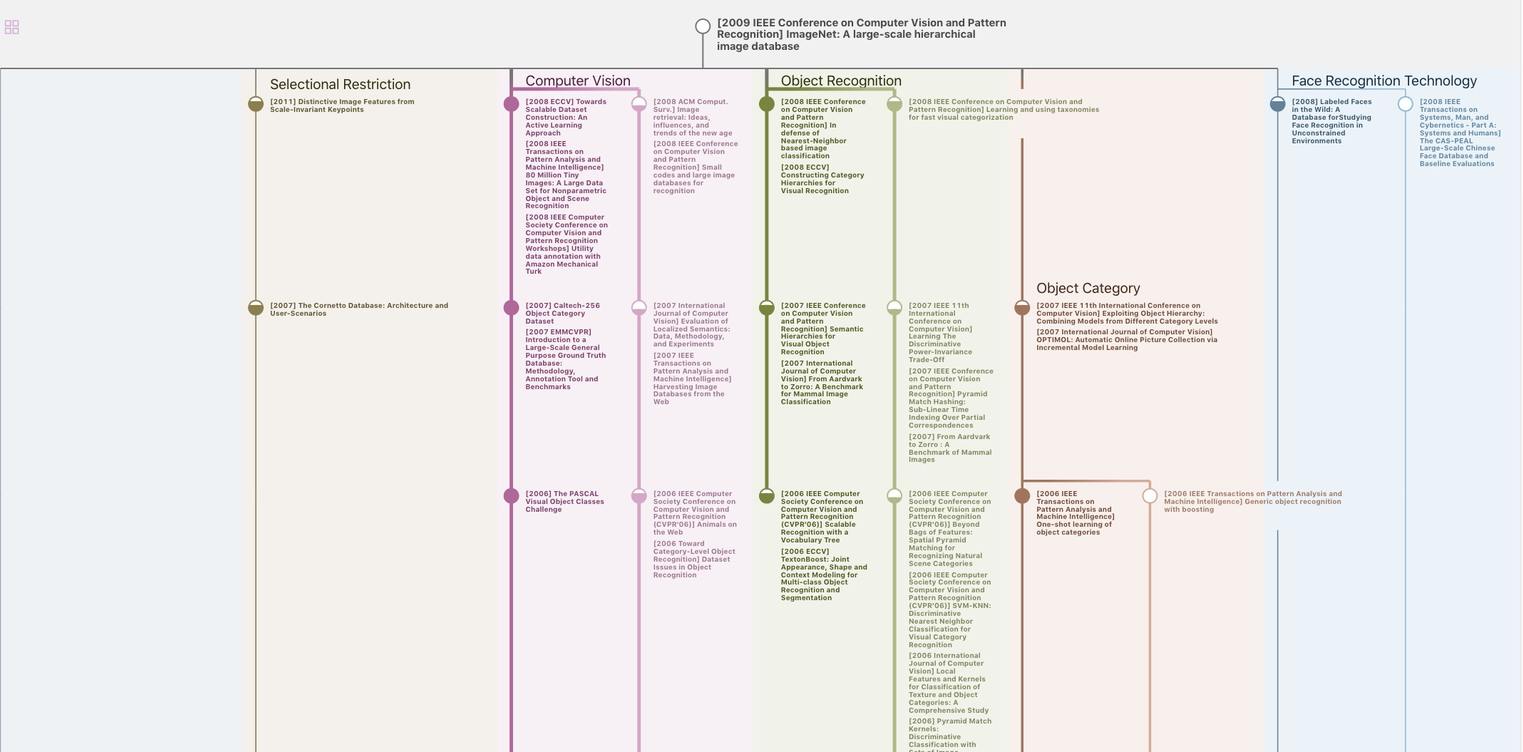
生成溯源树,研究论文发展脉络
Chat Paper
正在生成论文摘要