Imbalanced multi-label data classification as a bi-level optimization problem: application to miRNA-related diseases diagnosis
Neural Comput. Appl.(2023)
摘要
In multi-label classification, each instance could be assigned multiple labels at the same time. In such a situation, the relationships between labels and the class imbalance are two serious issues that should be addressed. Despite the important number of existing multi-label classification methods, the widespread class imbalance among labels has not been adequately addressed. Two main issues should be solved to come up with an effective classifier for imbalanced multi-label data. On the one hand, the imbalance could occur between labels and/or within a label. The “Between-labels imbalance” occurs where the imbalance is between labels however the “Within-label imbalance” occurs where the imbalance is in the label itself and it could occur across multiple labels. On the other hand, the labels’ processing order heavily influences the quality of a multi-label classifier. To deal with these challenges, we propose in this paper a bi-level evolutionary approach for the optimized induction of multivariate decision trees, where the upper-level role is to design the classifiers while the lower-level approximates the optimal labels’ ordering for each classifier. Our proposed method, named BIMLC-GA (Bi-level Imbalanced Multi-Label Classification Genetic Algorithm), is compared to several state-of-the-art methods across a variety of imbalanced multi-label data sets from several application fields and then applied on the miRNA-related diseases case study. The statistical analysis of the obtained results shows the merits of our proposal.
更多查看译文
关键词
Multi-label classification,Class imbalance,Bi-level optimization,Indicator-based evolutionary algorithm
AI 理解论文
溯源树
样例
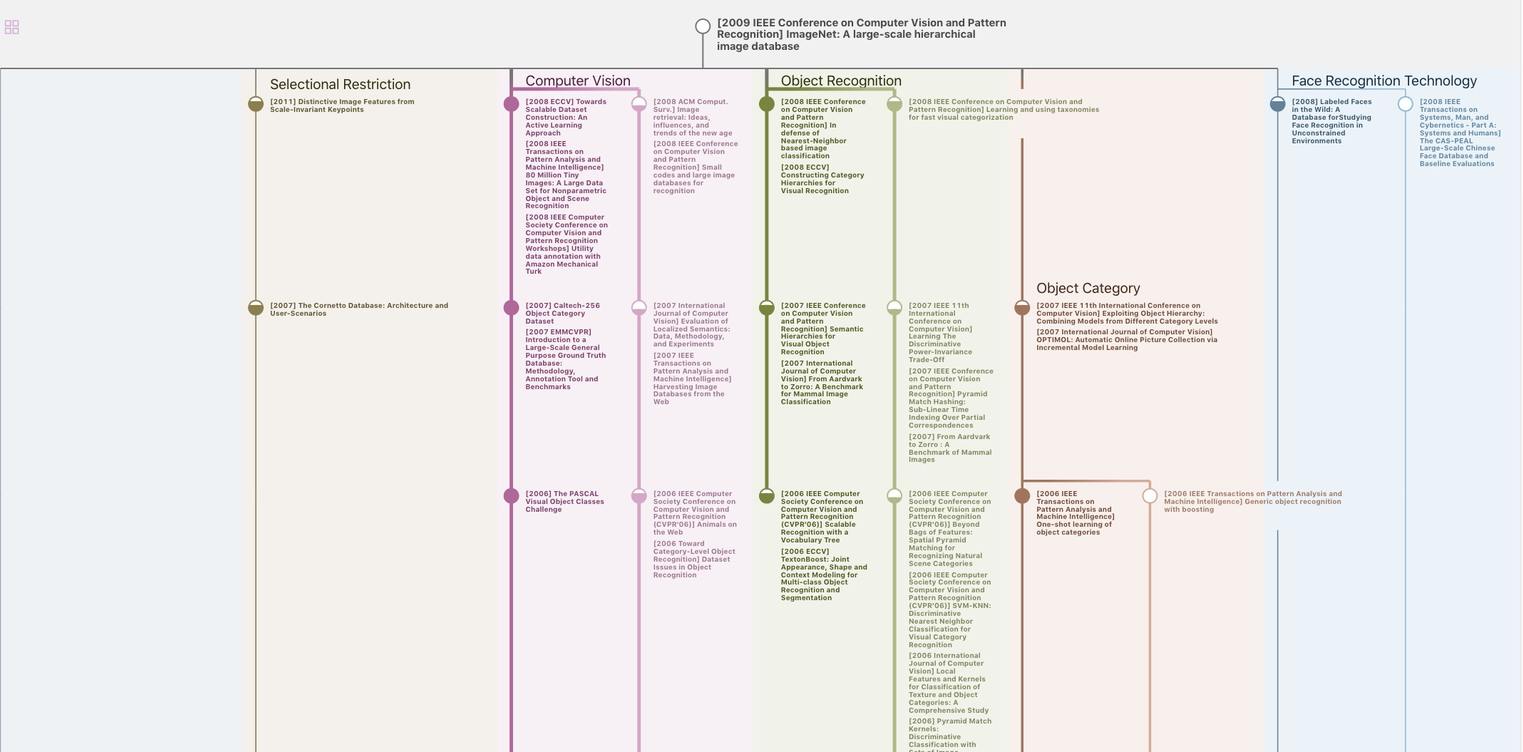
生成溯源树,研究论文发展脉络
Chat Paper
正在生成论文摘要