Negative Can Be Positive: Signed Graph Neural Networks for Recommendation.
Inf. Process. Manag.(2023)
摘要
Most of the existing GNN-based recommender system models focus on learning users' per-sonalized preferences from these (explicit/implicit) positive feedback to achieve personalized recommendations. However, in the real-world recommender system, the users' feedback be-havior also includes negative feedback behavior (e.g., click dislike button), which also reflects users' personalized preferences. How to utilize negative feedback is a challenging research problem. In this paper, we first qualitatively and quantitatively analyze the three kinds of negative feedback that widely existed in real-world recommender systems and investigate the role of negative feedback in recommender systems. We found that it is different from what we expected - not all negative items are ranked low, and some negative items are even ranked high in the overall items. Then, we propose a novel Signed Graph Neural Network Recommendation model (SiGRec) to encode the users' negative feedback behavior. Our SiGRec can learn positive and negative embeddings of users and items via positive and negative graph neural network encoders, respectively. Besides, we also define a new Sign Cosine (SiC) loss function to adaptively mine the information of negative feedback for different types of negative feedback. Extensive experiments on four datasets demonstrate the proposed model outperforms several existing models. Specifically, on the Zhihu dataset, SiGRec outperforms the unsigned GNN model (i.e., LightGCN), 27.58% 29.81%, and 31.21% in P@20, R@20, and nDCG@20, respectively. We hope our work can open the door to further exploring the negative feedback in recommendations.
更多查看译文
关键词
graph neural networks,recommendation,neural networks
AI 理解论文
溯源树
样例
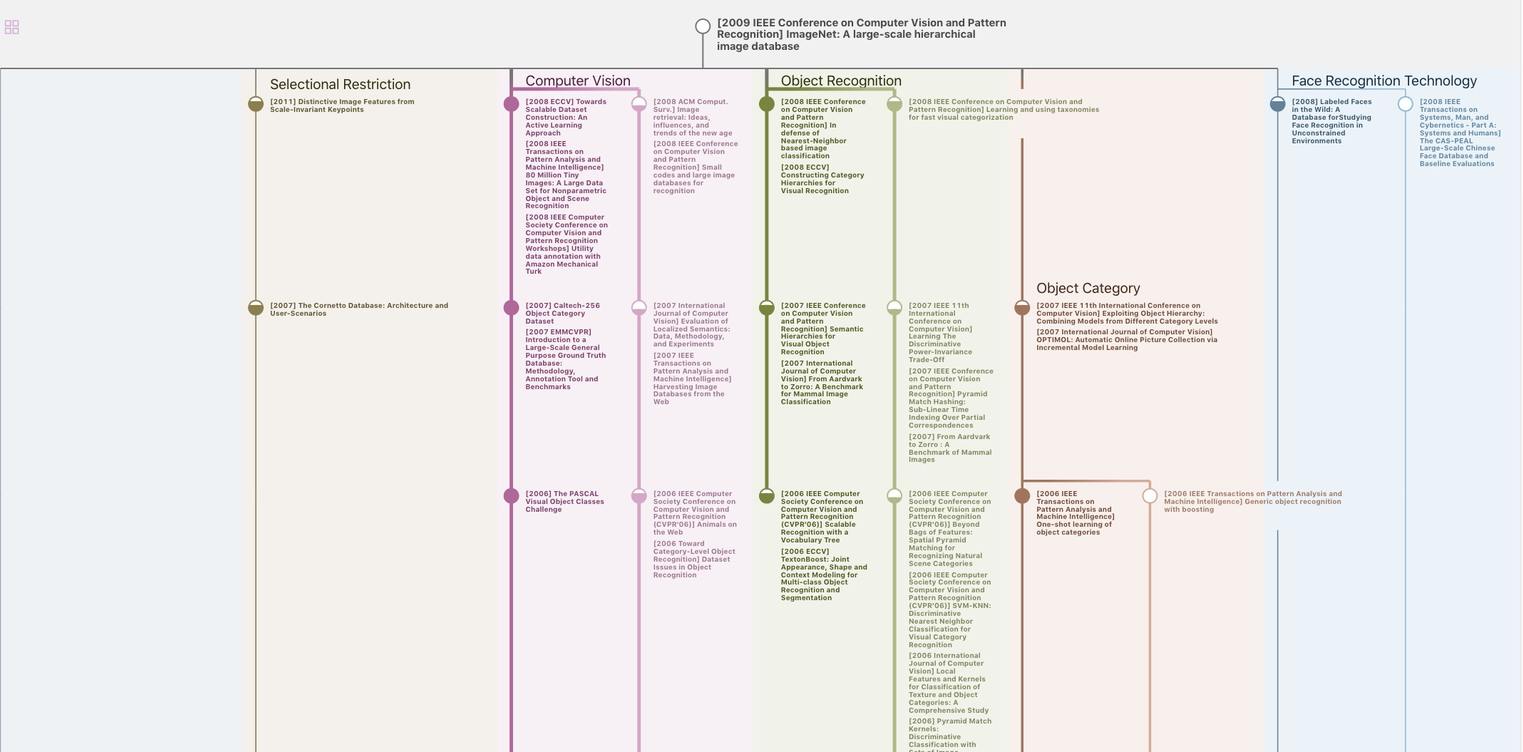
生成溯源树,研究论文发展脉络
Chat Paper
正在生成论文摘要