Towards Lightweight Deep Classification for Low-Resolution Synthetic Aperture Radar (SAR) Images: An Empirical Study.
Remote. Sens.(2023)
摘要
Numerous works have explored deep models for the classification of high-resolution natural images. However, limited investigation has been made into a deep classification for low-resolution synthetic aperture radar (SAR) images, which is a challenging yet important task in the field of remote sensing. Existing work adopted ROC-VGG, which has a huge amount of parameters, thus limiting its application in practical deployment. It remains unclear whether the techniques developed in high-resolution natural images to make the model lightweight can be effective for low-resolution SAR images. Therefore, with prior work as the baseline, this work conducts an empirical study, testing three popular lightweight techniques: (1) channel attention module; (2) spatial attention module; (3) multi-stream head. Our empirical results show that these lightweight techniques in the high-resolution natural image domain can also be effective in the low-resolution SAR domain. We reduce the parameters from 9.2M to 0.17M while improving the performance from 94.8% to 96.8%.
更多查看译文
关键词
lightweight deep classification,sar,radar,low-resolution
AI 理解论文
溯源树
样例
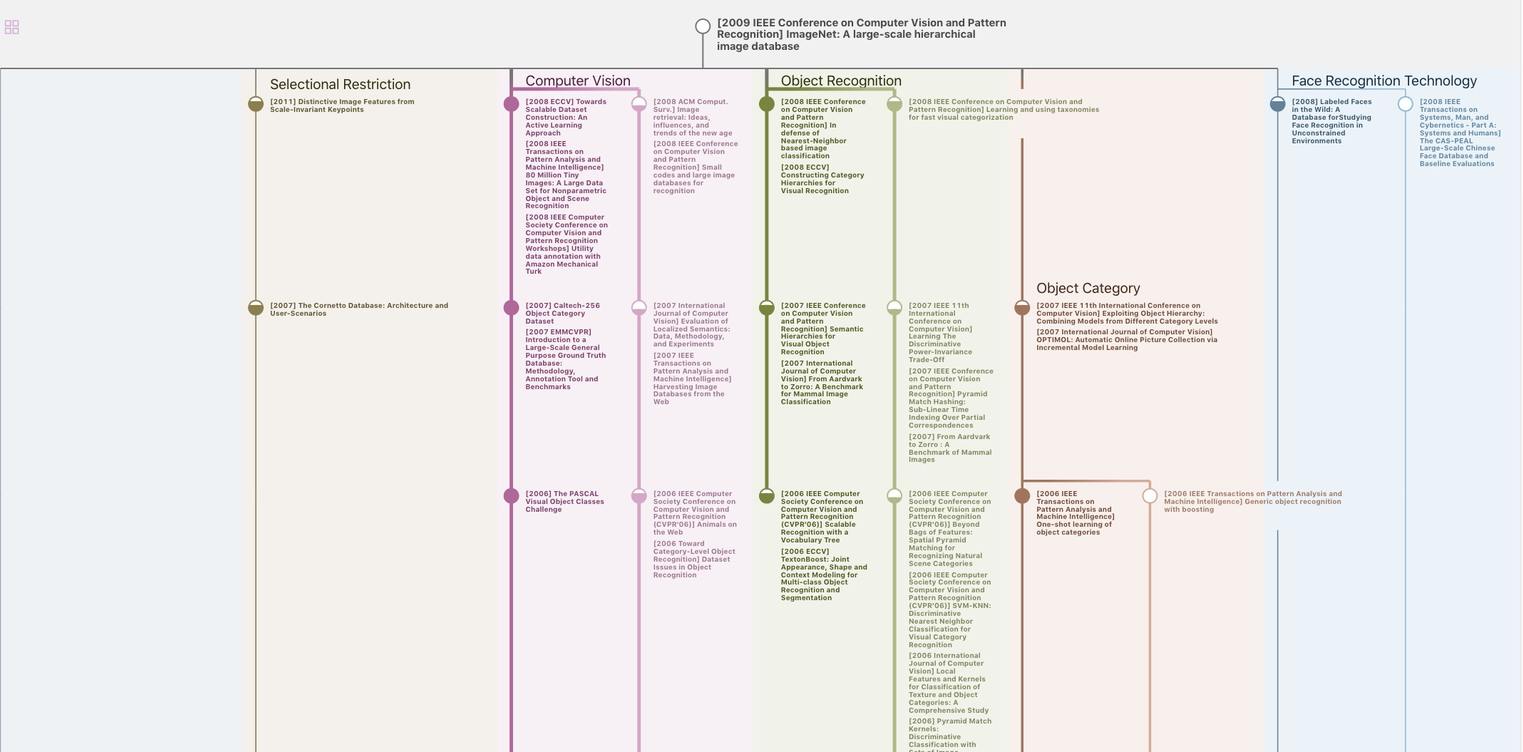
生成溯源树,研究论文发展脉络
Chat Paper
正在生成论文摘要