A novel structural damage detection method using a hybrid IDE-BP model.
Knowl. Based Syst.(2023)
摘要
Recently, artificial intelligence has been widely applied in the field of structural damage detection. In this study, a back-propagation neural network (BPNN) hybrid with an improved differential evolution algorithm (IDE) is proposed to advance the development of structural damage detection. Generally, most engineering structures' dynamic characteristics can be directly obtained by modal analysis, and the corresponding vibration features can provide information on structural damage. Thus, based on the results of modal analysis, artificial intelligent methods such as BP neural network find their way to locate the damage and quantify the damage severity. However, the existing research shows that the original BP has insufficient accuracy in structural damage detection, especially cannot effectively deal with complex structural damage. In this study, an improved differential evolution algorithm is proposed, introducing a group sampling strategy and an adaptive mutation strategy. Then, the proposed IDE and BP neural networks are hybridized into IDE-BP to promote the neural network's data classification and regression ability. Subsequently, based on the IDE-BP model, a method for detecting the location and severity of multi-damage scenarios is established. The decision tree algorithm (DT) is utilized to identify the number of damaged elements. To verify the effectiveness of the proposed damage detection method, the IDE-BP model is applied to the damage detection for a steel pipe and a cantilever plate. To study the anti-noise ability and robustness of the IDE-BP model, the noise and incomplete modes are introduced. Compared with the original BP, SVM, KNN, PSO-BP, and DE-BP, the numerical results show that the damage detection method based on the IDE-BP model performs better for all single and multiple damage scenarios.(c) 2023 Elsevier B.V. All rights reserved.
更多查看译文
关键词
hybrid ide–bp,damage
AI 理解论文
溯源树
样例
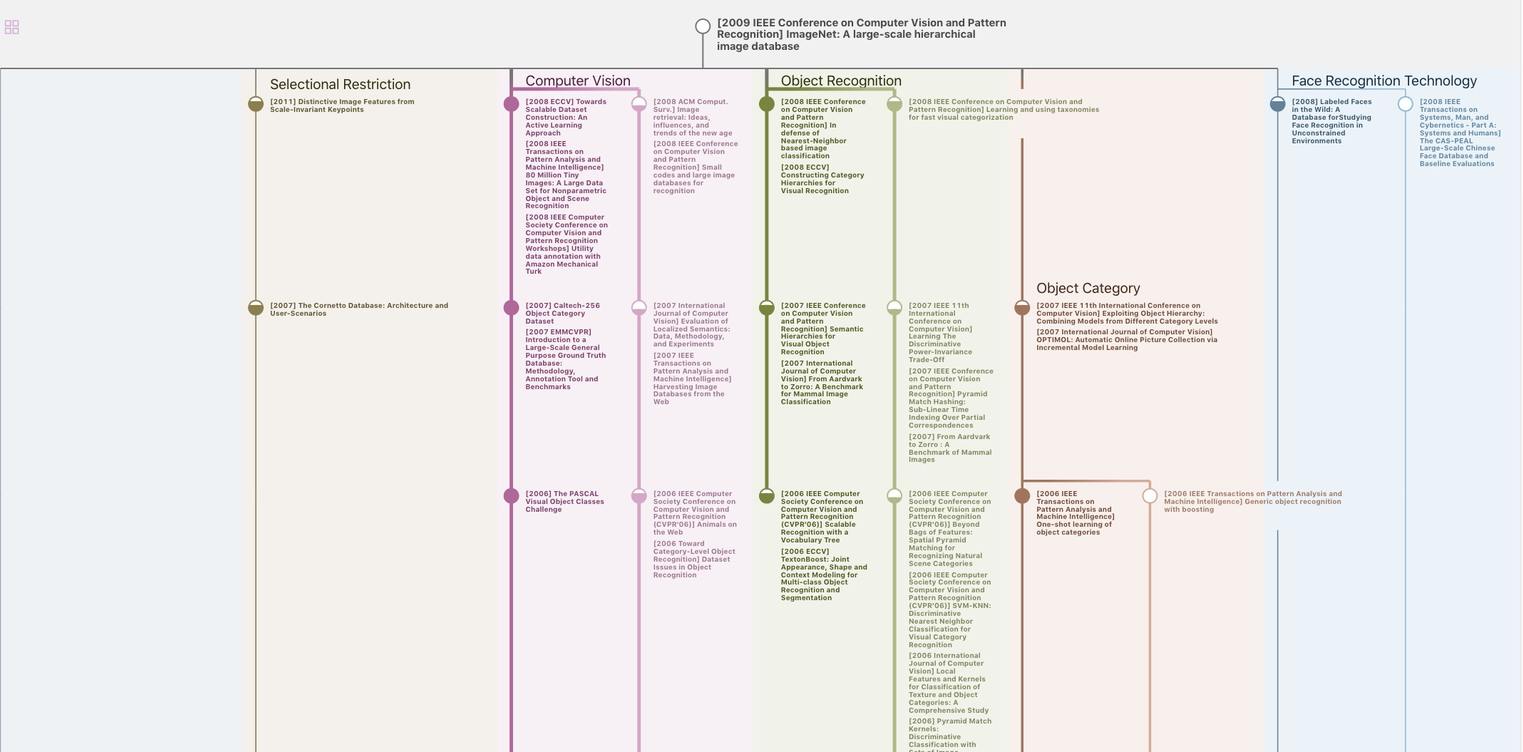
生成溯源树,研究论文发展脉络
Chat Paper
正在生成论文摘要