The shaky foundations of large language models and foundation models for electronic health records
NPJ digital medicine(2023)
摘要
The success of foundation models such as ChatGPT and AlphaFold has spurred significant interest in building similar models for electronic medical records (EMRs) to improve patient care and hospital operations. However, recent hype has obscured critical gaps in our understanding of these models’ capabilities. In this narrative review, we examine 84 foundation models trained on non-imaging EMR data (i.e., clinical text and/or structured data) and create a taxonomy delineating their architectures, training data, and potential use cases. We find that most models are trained on small, narrowly-scoped clinical datasets (e.g., MIMIC-III) or broad, public biomedical corpora (e.g., PubMed) and are evaluated on tasks that do not provide meaningful insights on their usefulness to health systems. Considering these findings, we propose an improved evaluation framework for measuring the benefits of clinical foundation models that is more closely grounded to metrics that matter in healthcare.
更多查看译文
关键词
electronic health records,large language models,language models
AI 理解论文
溯源树
样例
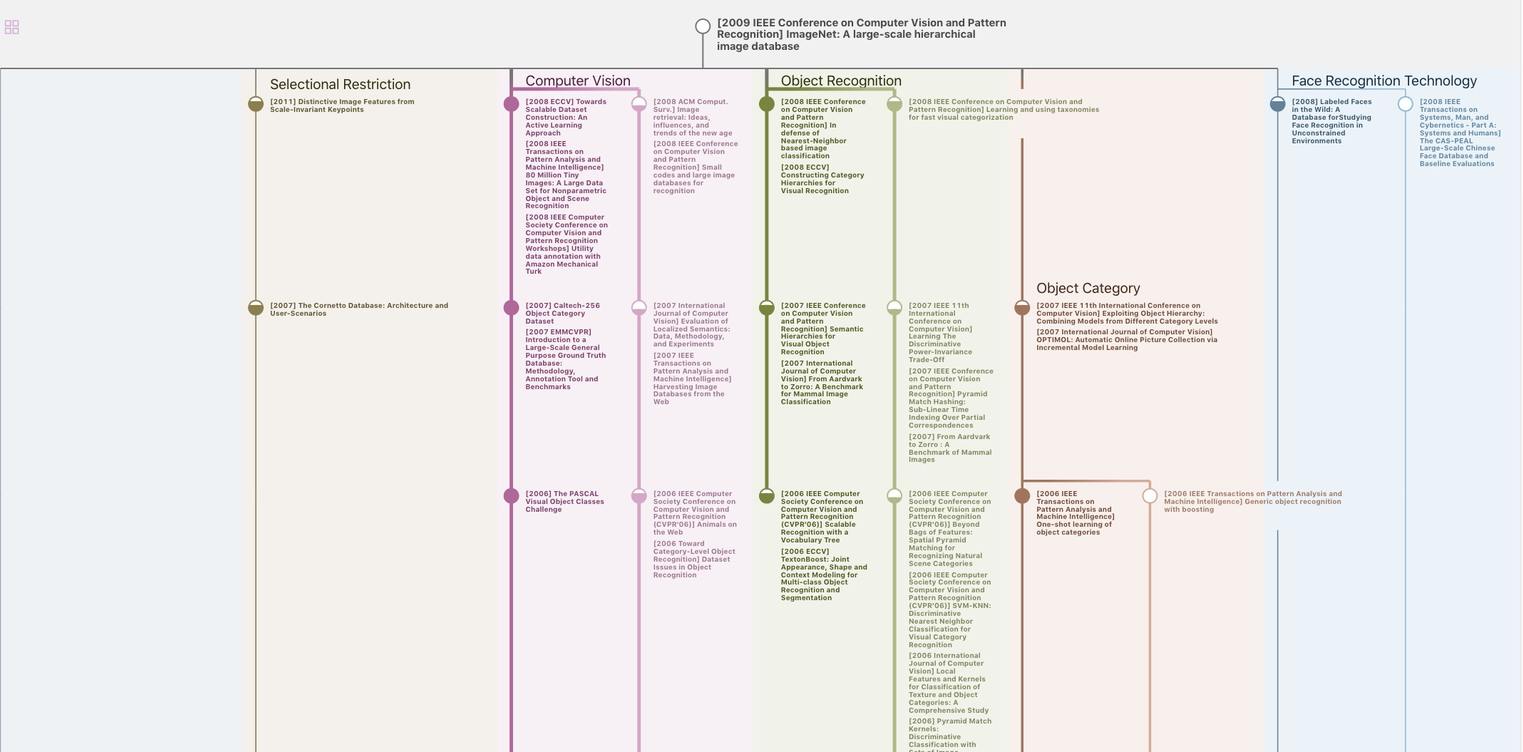
生成溯源树,研究论文发展脉络
Chat Paper
正在生成论文摘要