Cross modality generative learning framework for anatomical transitive Magnetic Resonance Imaging (MRI) from Electrical Impedance Tomography (EIT) image
Computerized medical imaging and graphics : the official journal of the Computerized Medical Imaging Society(2023)
摘要
This paper presents a cross-modality generative learning framework for transitive magnetic resonance imaging (MRI) from electrical impedance tomography (EIT). The proposed framework is aimed at converting low resolution EIT images to high-resolution wrist MRI images using a cascaded cycle generative adversarial network (CycleGAN) model. This model comprises three main components: the collection of initial EIT from the medical device, the generation of a high-resolution transitive EIT image from the corresponding MRI image for domain adaptation, and the coalescence of two CycleGAN models for cross-modality generation. The initial EIT image was generated at three different frequencies (70 kHz, 140 kHz, and 200 kHz) using a 16-electrode belt. Wrist T1-weighted images were acquired on a 1.5T MRI. A total of 19 normal volunteers were imaged using both EIT and MRI, which resulted in 713 paired EIT and MRI images. The cascaded CycleGAN, end to-end CycleGAN, and Pix2Pix models were trained and tested on the same cohort. The proposed method achieved the highest accuracy in bone detection, with 0.97 for the proposed cascaded CycleGAN, 0.68 for end to-end CycleGAN, and 0.70 for the Pix2Pix model. Visual inspection showed that the proposed method reduced bone-related errors in the MRI-style anatomical reference compared with end-to-end CycleGAN and Pix2Pix. Multifrequency EIT inputs reduced the testing normalized root mean squared error of MRI-style anatomical reference from 67.9% & PLUSMN; 12.7% to 61.4% & PLUSMN; 8.8% compared with that of single-frequency EIT. The mean conductivity values of fat and bone from regularized EIT were 0.0435 & PLUSMN; 0.0379 S/m and 0.0183 & PLUSMN; 0.0154 S/m, respectively, when the anatomical prior was employed. These results demonstrate that the proposed framework is able to generate MRI-style anatomical references from EIT images with a good degree of accuracy.
更多查看译文
关键词
Electrical Impedance Tomography,Magnetic Resonance Image,Generative networks,Medical images
AI 理解论文
溯源树
样例
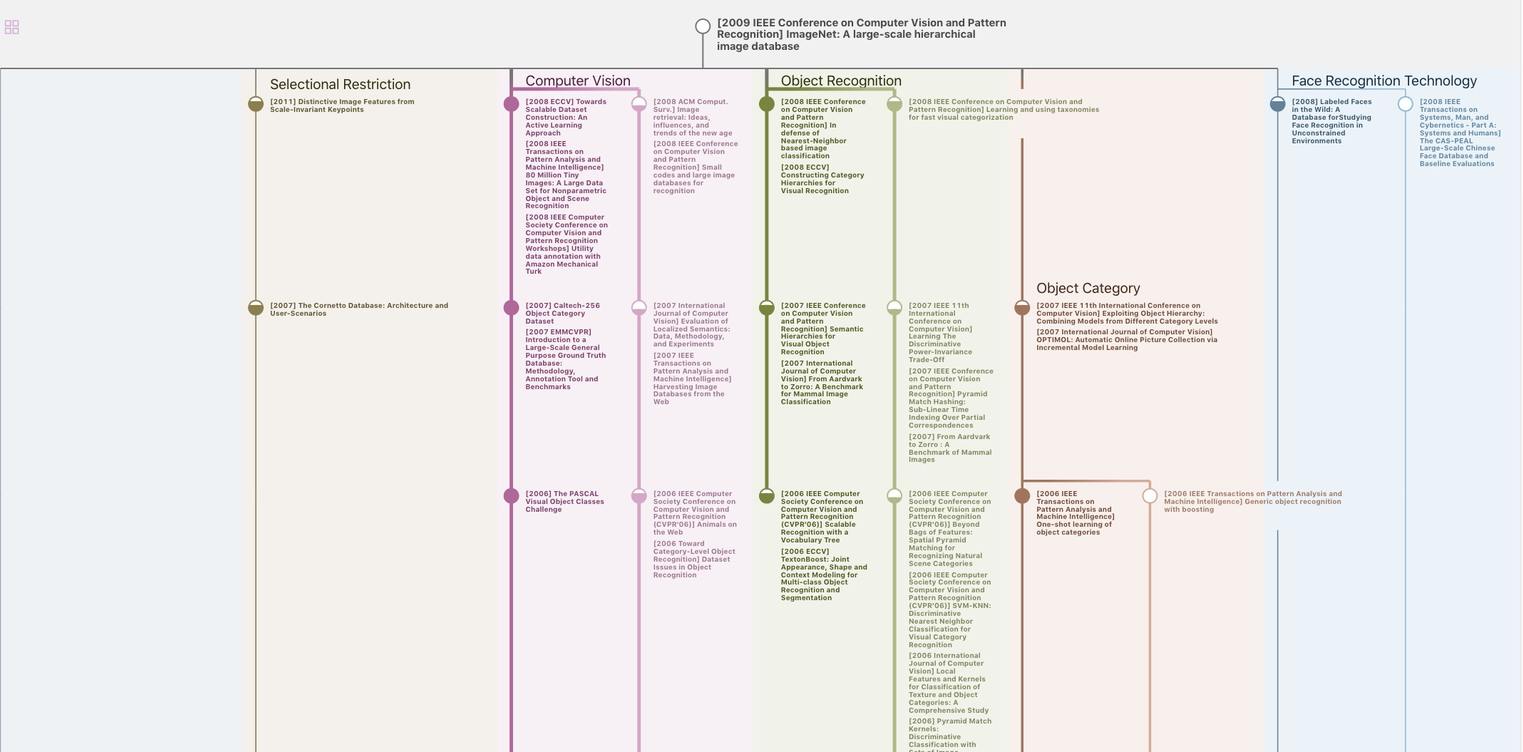
生成溯源树,研究论文发展脉络
Chat Paper
正在生成论文摘要