Universal Recurrent Event Memories for Streaming Data
2023 INTERNATIONAL JOINT CONFERENCE ON NEURAL NETWORKS, IJCNN(2023)
摘要
In this paper, we propose a new event memory architecture (MemNet) for recurrent neural networks, which is universal for different types of time series data such as scalar, multivariate or symbolic. Unlike other external neural memory architectures, it stores key-value pairs, which separate the information for addressing and for content to improve the representation, as in the digital archetype. Moreover, the key-value pairs also avoid the compromise between memory depth and resolution that applies to memories constructed by the model state. One of the MemNet key characteristics is that it requires only linear adaptive mapping functions while implementing a nonlinear operation on the input data. MemNet architecture can be applied without modifications to scalar time series, logic operators on strings, and also to natural language processing, providing state-of-the-art results in all application domains such as the chaotic time series, the symbolic operation tasks, and the question-answering tasks (bAbI). Finally, controlled by five linear layers, MemNet requires a much smaller number of training parameters than other external memory networks as well as the transformer network. The space complexity of MemNet equals a single self-attention layer. It greatly improves the efficiency of the attention mechanism and opens the door for IoT applications.
更多查看译文
关键词
universal recurrent event memories,data
AI 理解论文
溯源树
样例
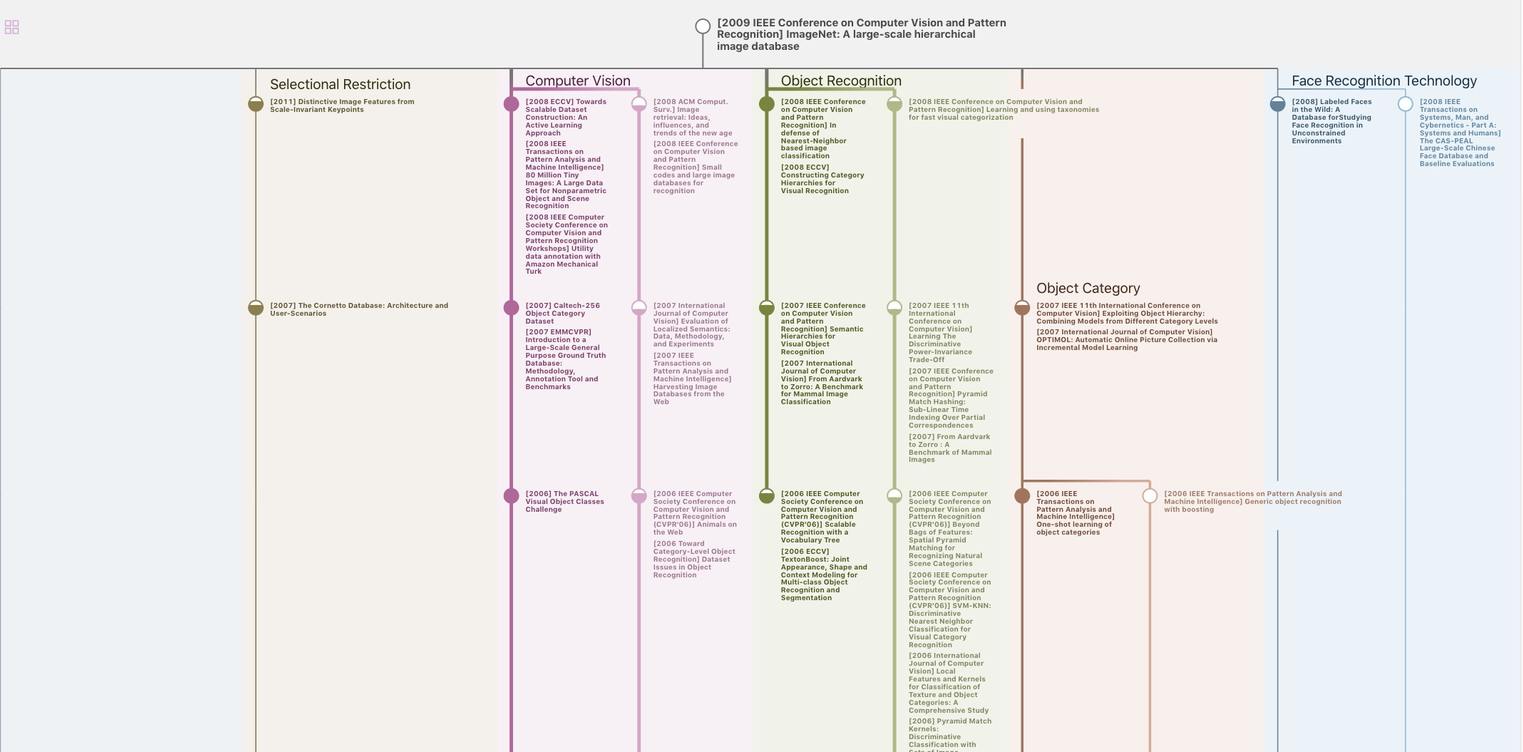
生成溯源树,研究论文发展脉络
Chat Paper
正在生成论文摘要