Multi-layer Aggregation as a Key to Feature-Based OOD Detection
UNCERTAINTY FOR SAFE UTILIZATION OF MACHINE LEARNING IN MEDICAL IMAGING, UNSURE 2023(2023)
摘要
Deep Learning models are easily disturbed by variations in the input images that were not observed during the training stage, resulting in unpredictable predictions. Detecting such Out-of-Distribution (OOD) images is particularly crucial in the context of medical image analysis, where the range of possible abnormalities is extremely wide. Recently, a new category of methods has emerged, based on the analysis of the intermediate features of a trained model. These methods can be divided into 2 groups: single-layer methods that consider the feature map obtained at a fixed, carefully chosen layer, and multi-layer methods that consider the ensemble of the feature maps generated by the model. While promising, a proper comparison of these algorithms is still lacking. In thiswork, we compared various feature-based OOD detection methods on a large spectra of OOD (20 types), representing approximately 7800 3D MRIs. Our experiments shed the light on two phenomenons. First, multi-layer methods consistently outperform single-layer approaches, which tend to have inconsistent behaviour depending on the type of anomaly. Second, the OOD detection performance is variable depending on the architecture of the underlying neural network. We provide our implementation of the feature-based OOD detectors at https://github.com/benolmbrt/MedicOOD.
更多查看译文
关键词
Uncertainty,Deep learning,Anomaly Detection,Medical images analysis
AI 理解论文
溯源树
样例
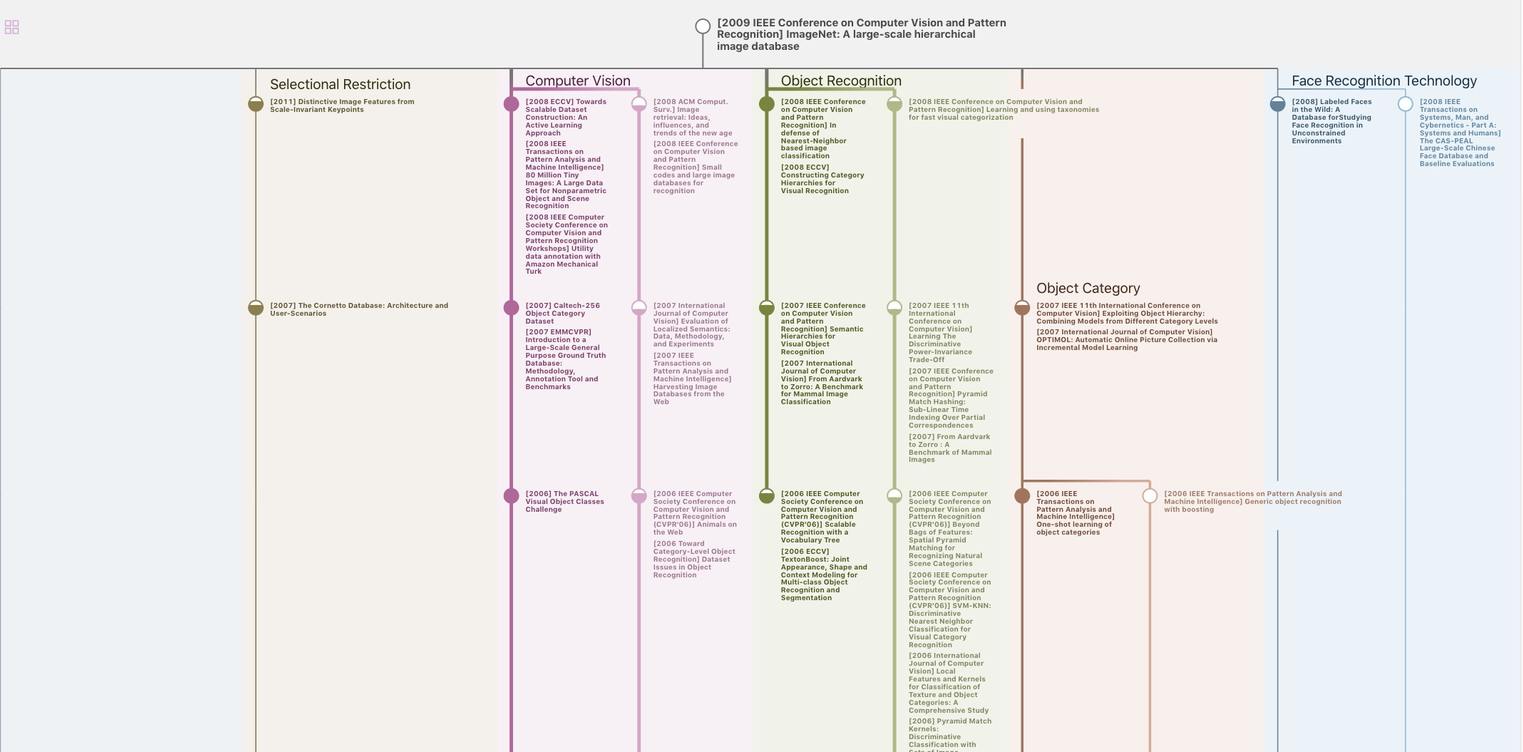
生成溯源树,研究论文发展脉络
Chat Paper
正在生成论文摘要