Shrink-Perturb Improves Architecture Mixing during Population Based Training for Neural Architecture Search
CoRR(2023)
摘要
In this work, we show that simultaneously training and mixing neural networks is a promising way to conduct Neural Architecture Search (NAS). For hyperparameter optimization, reusing the partially trained weights allows for efficient search, as was previously demonstrated by the Population Based Training (PBT) algorithm. We propose PBT-NAS, an adaptation of PBT to NAS where architectures are improved during training by replacing poorly-performing networks in a population with the result of mixing well-performing ones and inheriting the weights using the shrink-perturb technique. After PBT-NAS terminates, the created networks can be directly used without retraining. PBT-NAS is highly parallelizable and effective: on challenging tasks (image generation and reinforcement learning) PBT-NAS achieves superior performance compared to baselines (random search and mutation-based PBT).
更多查看译文
关键词
architecture mixing,shrink-perturb
AI 理解论文
溯源树
样例
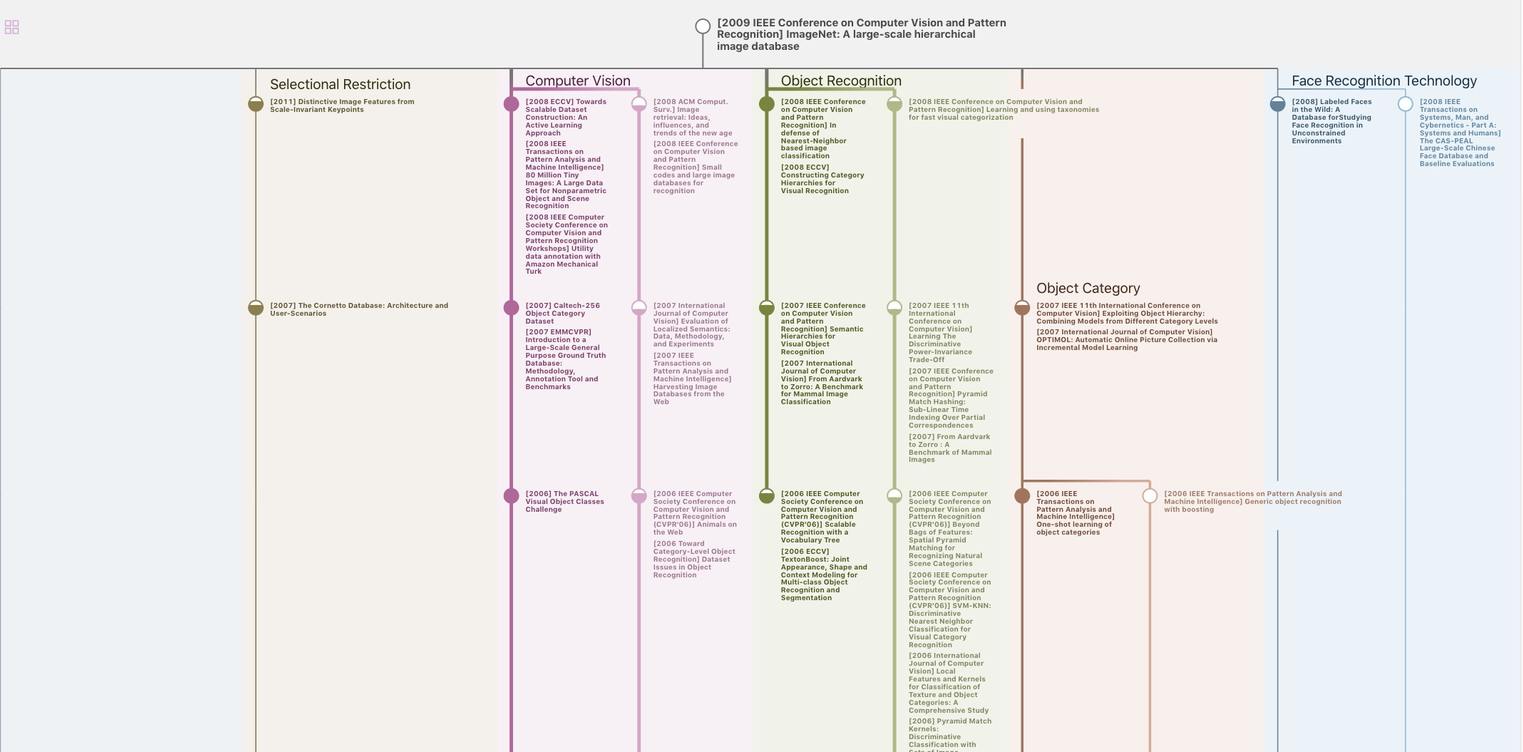
生成溯源树,研究论文发展脉络
Chat Paper
正在生成论文摘要