Learning to Collaborate by Grouping: a Consensus-oriented Strategy for Multi-agent Reinforcement Learning
CoRR(2023)
摘要
Multi-agent systems require effective coordination between groups and individuals to achieve common goals. However, current multi-agent reinforcement learning (MARL) methods primarily focus on improving individual policies and do not adequately address group-level policies, which leads to weak cooperation. To address this issue, we propose a novel Consensus-oriented Strategy (CoS) that emphasizes group and individual policies simultaneously. Specifically, CoS comprises two main components: (a) the vector quantized group consensus module, which extracts discrete latent embeddings that represent the stable and discriminative group consensus, and (b) the group consensus-oriented strategy, which integrates the group policy using a hypernet and the individual policies using the group consensus, thereby promoting coordination at both the group and individual levels. Through empirical experiments on cooperative navigation tasks with both discrete and continuous spaces, as well as Google research football, we demonstrate that CoS outperforms state-of-the-art MARL algorithms and achieves better collaboration, thus providing a promising solution for achieving effective coordination in multi-agent systems.
更多查看译文
关键词
collaborate,grouping,reinforcement,consensus-oriented,multi-agent
AI 理解论文
溯源树
样例
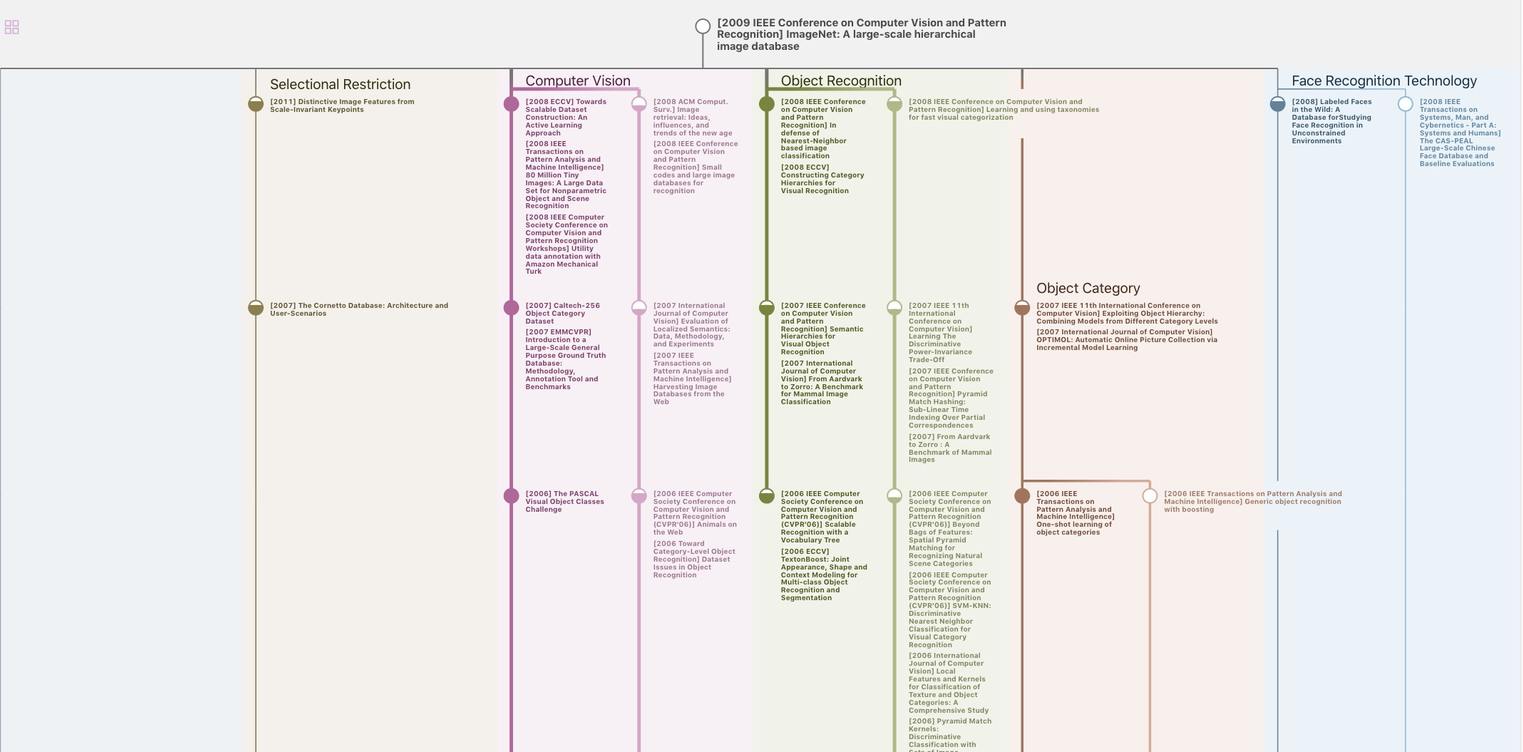
生成溯源树,研究论文发展脉络
Chat Paper
正在生成论文摘要