Defocus Blur Synthesis and Deblurring via Interpolation and Extrapolation in Latent Space
COMPUTER ANALYSIS OF IMAGES AND PATTERNS, CAIP 2023, PT II(2023)
摘要
Though modern microscopes have an autofocusing system to ensure optimal focus, out-of-focus images can still occur when cells within the medium are not all in the same focal plane, affecting the image quality for medical diagnosis and analysis of diseases. We propose a method that can deblur images as well as synthesize defocus blur. We train autoencoders with implicit and explicit regularization techniques to enforce linearity relations among the representations of different blur levels in the latent space. This allows for the exploration of different blur levels of an object by linearly interpolating/extrapolating the latent representations of images taken at different focal planes. Compared to existing works, we use a simple architecture to synthesize images with flexible blur levels, leveraging the linear latent space. Our regularized autoencoders can effectively mimic blur and deblur, increasing data variety as a data augmentation technique and improving the quality of microscopic images, which would be beneficial for further processing and analysis. The code is available at https://github.com/nis-research/linear-latent-blur.
更多查看译文
关键词
Microscope images,Deblurring,Defocus blur synthesis,Regularized autoencoders
AI 理解论文
溯源树
样例
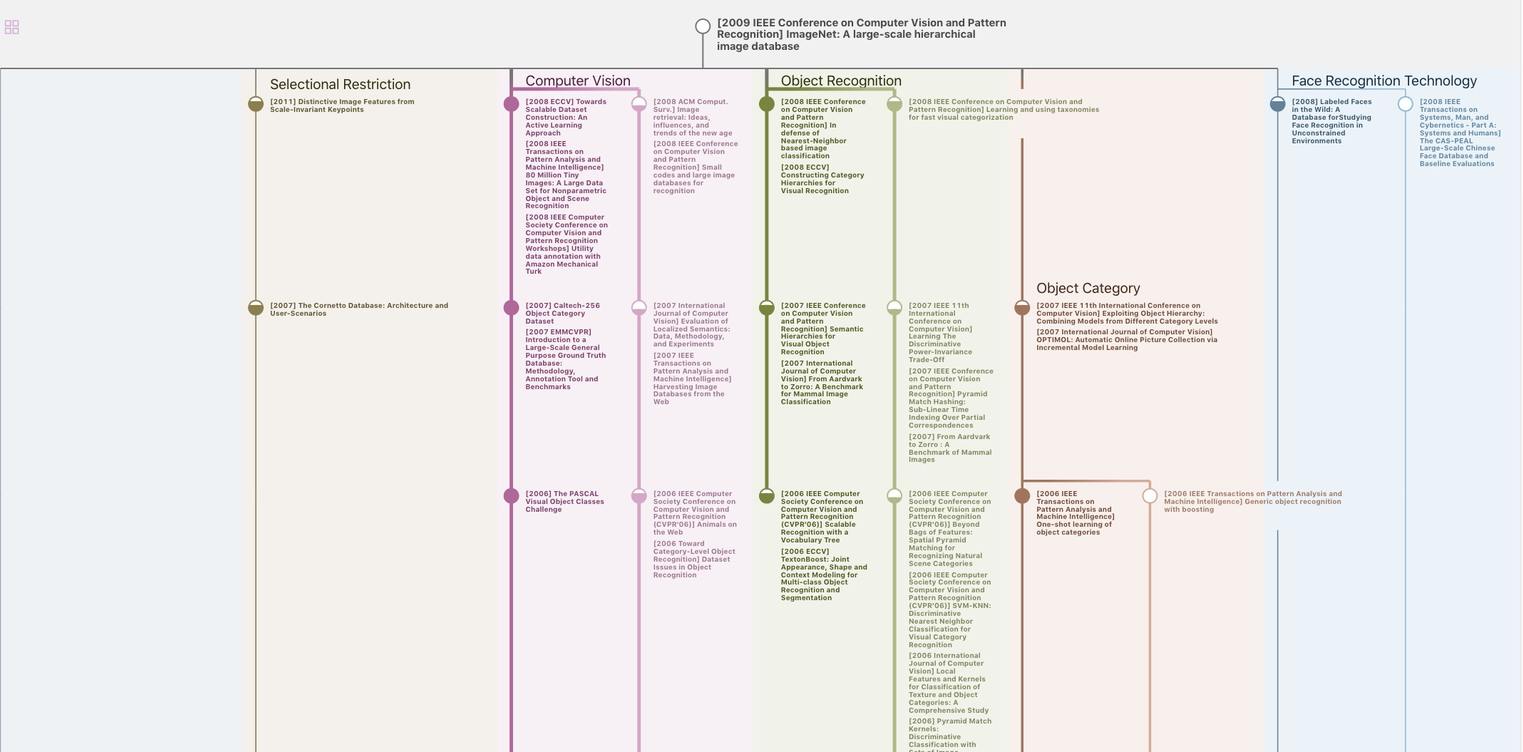
生成溯源树,研究论文发展脉络
Chat Paper
正在生成论文摘要