Investigating the Learning Behaviour of In-context Learning: A Comparison with Supervised Learning
CoRR(2023)
摘要
Large language models (LLMs) have shown remarkable capacity for in-context learning (ICL), where learning a new task from just a few training examples is done without being explicitly pre-trained. However, despite the success of LLMs, there has been little understanding of how ICL learns the knowledge from the given prompts. In this paper, to make progress toward understanding the learning behaviour of ICL, we train the same LLMs with the same demonstration examples via ICL and supervised learning (SL), respectively, and investigate their performance under label perturbations (i.e., noisy labels and label imbalance) on a range of classification tasks. First, via extensive experiments, we find that gold labels have significant impacts on the downstream in-context performance, especially for large language models; however, imbalanced labels matter little to ICL across all model sizes. Second, when comparing with SL, we show empirically that ICL is less sensitive to label perturbations than SL, and ICL gradually attains comparable performance to SL as the model size increases.
更多查看译文
关键词
learning behaviour,in-context
AI 理解论文
溯源树
样例
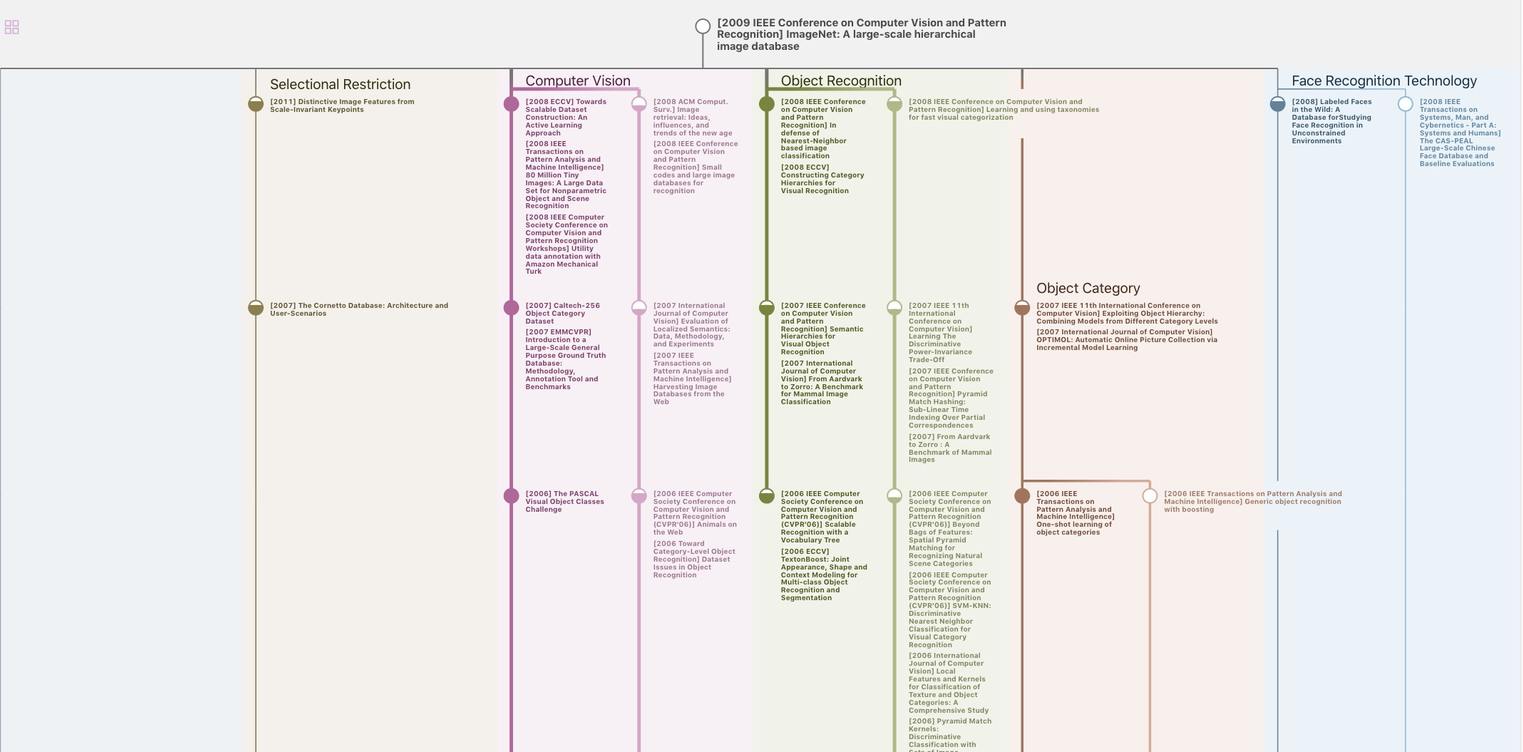
生成溯源树,研究论文发展脉络
Chat Paper
正在生成论文摘要