A Practical Recipe for Federated Learning Under Statistical\n Heterogeneity Experimental Design
IEEE Transactions on Artificial Intelligence(2023)
Abstract
Federated Learning (FL) has been an area of active research in recent years. There have been numerous studies in FL to make it more successful in the presence of data heterogeneity. However, despite the existence of many publications, the state of progress in the field is unknown. Many of the works use inconsistent experimental settings and there are no comprehensive studies on the effect of FL-specific experimental variables on the results and practical insights for a more comparable and consistent FL experimental setup. Furthermore, the existence of several benchmarks and confounding variables has further complicated the issue of inconsistency and ambiguity. In this work, we present the first comprehensive study on the effect of FL-specific experimental variables in relation to each other and performance results, bringing several insights and recommendations for designing a meaningful and well-incentivized FL experimental setup. We further aid the community by releasing FedZoo-Bench, an open-source library based on PyTorch with pre-implementation of 22 state-of-the-art methods, and a broad set of standardized and customizable features available at https://github.com/MMorafah/FedZoo-Bench. We also provide a comprehensive comparison of several state-of-the-art (SOTA) methods to better understand the current state of the field and existing limitations.
MoreTranslated text
Key words
Benchmark,Data Heterogeneity,Exper-imental Design,Federated Learning,Machine Learning,Non-IID Data
AI Read Science
Must-Reading Tree
Example
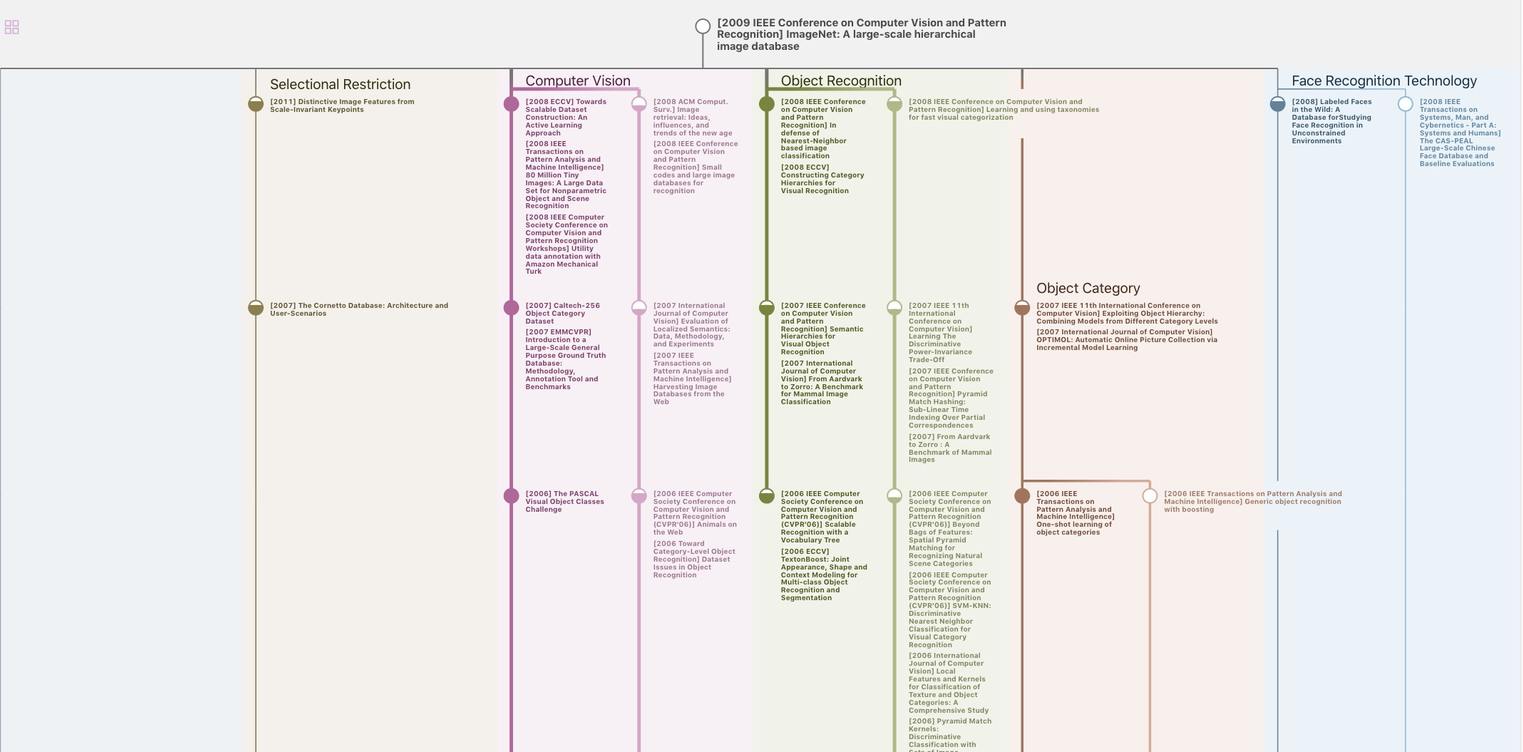
Generate MRT to find the research sequence of this paper
Chat Paper
Summary is being generated by the instructions you defined