Using Synthetic MRI and Radiomics to Predict Treatment Response in Triple-Negative Breast Cancer
RADIOLOGY-IMAGING CANCER(2023)
摘要
HomeRadiology: Imaging CancerVol. 5, No. 4 PreviousNext CommentaryFree AccessUsing Synthetic MRI and Radiomics to Predict Treatment Response in Triple-Negative Breast CancerMargaret Houser, Jocelyn A. Rapelyea Margaret Houser, Jocelyn A. Rapelyea Author AffiliationsFrom the Department of Radiology, The George Washington University School of Medicine and Health Sciences, 2150 Pennsylvania Ave NW, Washington, DC 20037.Address correspondence to J.A.R. (email: [email protected]).Margaret HouserJocelyn A. Rapelyea Published Online:Jul 28 2023https://doi.org/10.1148/rycan.230095MoreSectionsPDF ToolsImage ViewerAdd to favoritesCiteTrack CitationsPermissionsReprints ShareShare onFacebookTwitterLinked In See also the article by Hwang et al in this issue.Margaret Houser, MD, is a breast imaging fellow and junior faculty at The George Washington University Hospital. During her 3rd year of radiology residency, she was selected to participate in the Resident Academic Leadership Development Program organized by the Association of University Radiologists. Her research interests include MRI in women's imaging; she co-authored a book chapter on MRI of the pelvis in female infertility and recently published an article on emerging uses of breast MRI.Download as PowerPointOpen in Image Viewer Jocelyn A. Rapelyea, MD, is board certified in diagnostic radiology and is a professor at The George Washington University School of Medicine and Health Sciences. Dr Rapelyea also serves as the associate director of breast imaging. She has received numerous honors and appointments throughout her career, including fellowship in the Society of Breast Imaging and the American College of Radiology. She has published abstracts, articles, and chapters and has made various national and international presentations.Download as PowerPointOpen in Image Viewer Breast MRI is the most sensitive tool in evaluating breast cancer, compared with other current clinical imaging modalities. Its use as a diagnostic and screening tool has demonstrated superior performance, allowing for discrimination between benign and malignant disease. Although various techniques, such as diffusion-weighted imaging and newer ultrafast techniques, have improved the assessment of breast lesions, several studies reported increased specificity for malignant and benign differentiation with T2-weighted imaging (1). An emerging technique called synthetic MRI (SyMRI) uses T2 relaxation times and echo times to help distinguish between normal and abnormal tissue and measure their properties with improved accuracy. T2 relaxation time depends on the chemical components of the tissue and can help detect pathologic changes from normal tissue.The use of SyMRI is expanding beyond accurately diagnosing breast cancer from enhancing masses in dynamic contrast-enhanced MRI, with important implications for clinical practice. Zhang and colleagues (2) accurately predicted Ki-67 expression levels in invasive ductal cancer using SyMRI. Meanwhile, Du et al (3) demonstrated that SyMRI T1 values were linked to the triple-negative subtype, and a combination of T2 parameters could enhance the discrimination of the luminal A subtype in invasive breast cancer. SyMRI does not require an injection of gadolinium-based contrast agents; instead, it is used as a fast noncontrast sequence acquisition and has been found to provide the same high-quality diagnostic information as does conventional MRI (4). Certain authors have successfully used SyMRI and dynamic contrast-enhanced MRI to forecast the response of neoadjuvant treatment in patients with triple-negative breast cancer (5). In the current study from this issue of Radiology: Imaging Cancer, Hwang et al (6) evaluated quantitative T2 values in individuals with triple-negative breast cancer in the neoadjuvant setting. Others have supported the findings that the T2 value is significantly lower in responders to treatment compared with nonresponders (7).Researchers have demonstrated promising results in the growing field of SyMRI of the breast. In 2021, Fujioka et al (8) found that SyMRI T1- and T2-weighted sequences had similar diagnostic capabilities as conventionally acquired sequences; only fat-saturated T2 images did not provide the same information. Chung et al (9) reported that with the help of artificial intelligence (AI) and deep learning, contrast-enhanced T1-weighted breast MRI studies could be simulated by acquiring five precontrast sequences and can yield diagnostic information regarding important breast cancer postcontrast imaging characteristics.In the current article, Hwang et al (6) are, to our knowledge, the first to apply radiomics and AI to breast SyMRI. They looked at the ability of radiomic features at SyMRI to predict pathologic complete response to neoadjuvant systemic chemotherapy in 181 participants with triple-negative breast cancer. As is well known, triple-negative breast cancers are aggressive and have a poor prognosis. Because these tumors lack estrogen and progesterone receptors, hormone therapy and drugs that target human epidermal growth factor receptor 2, or HER2, protein are not helpful; thus, chemotherapy is the primary treatment option. In the current prospective study, participants underwent systemic chemotherapy for up to 24 weeks before undergoing a postchemotherapy MRI examination to determine tumor response before surgery. Imaging that demonstrates a complete response to chemotherapy before surgery, as opposed to no complete response, indicates better outcomes (10). The ability to predict a patient's response to chemotherapy treatment through specific imaging features can help personalize the chemotherapy lengths and management for every patient.The study by Hwang et al (6) yielded impressive results: 15, 10, and 12 radiomic features on synthetically produced proton density, T1, and T2 maps, respectively, predicted pathologic complete response to chemotherapy after only four chemotherapy cycles, with an impressively high area under the receiver operating characteristic curve of greater than 0.70. Using midtreatment SyMRI scans, the authors found that AI and SyMRI maps can analyze features to distinguish between participants who have had a pathologic complete response to chemotherapy and those who have not. The study demonstrates that SyMRI mapping provides an advantage over conventional MRI studies by accurately representing the physical properties of the imaged tissue without being affected by different protocols or scanners. This allows for a more precise understanding of the tissue's properties, leading to better patient outcomes (6). Patients who do not demonstrate specific imaging features can stop toxic chemotherapy, and alternative treatment plans can be pursued.SyMRI is an emerging technology that enables the reconstruction of multiple imaging sequences from a single scan acquisition. While the authors have presented a promising predictive model of pathologic response and a way to individualize patient chemotherapy treatment plans, one should be mindful of the resources needed to implement such a system. It is true that SyMRI decreases time in the MRI scanner and negates the need to inject patients with gadolinium-based media. Additionally, it eliminates misregistration from motion, as all synthetic sequences are based upon a single acquired sequence. However, it should be noted that tumor segmentation needed for subsequent AI analysis is highly time-consuming for the MRI technologist, and the radiologist's interpretation time may also increase. Given additional postprocessing and interpretation time and the upfront expense to purchase the technology needed for such a program, using AI and SyMRI to determine a patient's pathologic response to chemotherapy may only be feasible for some practices.Once such technology is widely accessible and technically feasible to implement in daily workflow, it is prudent to clearly delineate the role of SyMRI in breast imaging. This original and innovative study shows that SyMRI can be a diagnostic tool to discriminate between patients with triple-negative breast cancer undergoing systemic chemotherapy who will respond well to treatment and those who will not. Future studies can further expand upon the possible indications of SyMRI. Contrast-enhanced mammography is another emerging tool that helps provide valuable diagnostic information; while typically a screening examination, it has been emerging as a potential alternative to contrast-enhanced MRI. Future studies looking at how these tools can work together to provide the best individualized care for our patients would be a welcome addition to the literature.Disclosures of conflicts of interest: M.H. No relevant relationships. J.A.R. Honorarium from GE Healthcare for speakers bureau service; associate editor for Radiology: Imaging Cancer.Authors declared no funding for this work.References1. Arponen O, Masarwah A, Sutela A, et al. Incidentally detected enhancing lesions found in breast MRI: analysis of apparent diffusion coefficient and T2 signal intensity significantly improves specificity. Eur Radiol 2016;26(12):4361–4370. Crossref, Medline, Google Scholar2. Zhang L, Hao J, Guo J, Zhao X, Yin X. Predicting of Ki-67 expression level using diffusion-weighted and synthetic magnetic resonance imaging in invasive ductal breast cancer. Breast J 2023;2023:6746326. Crossref, Medline, Google Scholar3. Du S, Gao S, Zhang L, Yang X, Qi X, Li S. Improved discrimination of molecular subtypes in invasive breast cancer: Comparison of multiple quantitative parameters from breast MRI. Magn Reson Imaging 2021;77:148–158. Crossref, Medline, Google Scholar4. Tanenbaum LN, Tsiouris AJ, Johnson AN, et al. Synthetic MRI for clinical neuroimaging: Results of the Magnetic Resonance Image Compilation (MAGiC) prospective, multicenter, multireader trial. AJNR Am J Neuroradiol 2017;38(6):1103–1110. Crossref, Medline, Google Scholar5. Matsuda M, Fukuyama N, Matsuda T, et al. Utility of synthetic MRI in predicting pathological complete response of various breast cancer subtypes prior to neoadjuvant chemotherapy. Clin Radiol 2022;77(11):855–863. Crossref, Medline, Google Scholar6. Hwang KP, Elshafeey NA, Kotrotsou A, et al. A radiomics model based on synthetic MRI acquisition for predicting neoadjuvant systemic treatment response in triple-negative breast cancer. Radiol Imaging Cancer 2023;5(4):e230009. Google Scholar7. Tan PC, Pickles MD, Lowry M, Manton DJ, Turnbull LW. Lesion T(2) relaxation times and volumes predict the response of malignant breast lesions to neoadjuvant chemotherapy. Magn Reson Imaging 2008;26(1):26–34. Crossref, Medline, Google Scholar8. Fujioka T, Mori M, Oyama J, et al. Investigating the image quality and utility of Synthetic MRI in the Breast. Magn Reson Med Sci 2021;20(4):431–438. Crossref, Medline, Google Scholar9. Chung M, Calabrese E, Mongan J, et al. Deep learning to simulate contrast-enhanced breast MRI of invasive breast cancer. Radiology 2022;306(3):e213199 [Published correction appears in Radiology 2023;306(3):e239004.] Link, Google Scholar10. Spring LM, Fell G, Arfe A, et al. Pathologic complete response after neoadjuvant chemotherapy and impact on breast cancer recurrence and survival: a comprehensive meta-analysis. Clin Cancer Res 2020;26(12):2838–2848. Crossref, Medline, Google ScholarArticle HistoryReceived: June 20 2023Revision requested: June 20 2023Revision received: July 5 2023Accepted: July 10 2023Published online: July 28 2023 FiguresReferencesRelatedDetailsAccompanying This ArticleA Radiomics Model Based on Synthetic MRI Acquisition for Predicting Neoadjuvant Systemic Treatment Response in Triple-Negative Breast CancerJul 28 2023Radiology: Imaging CancerRecommended Articles RSNA Education Exhibits RSNA Case Collection Vol. 5, No. 4 Metrics Altmetric Score PDF download
更多查看译文
关键词
synthetic mri,breast cancer,radiomics,triple-negative
AI 理解论文
溯源树
样例
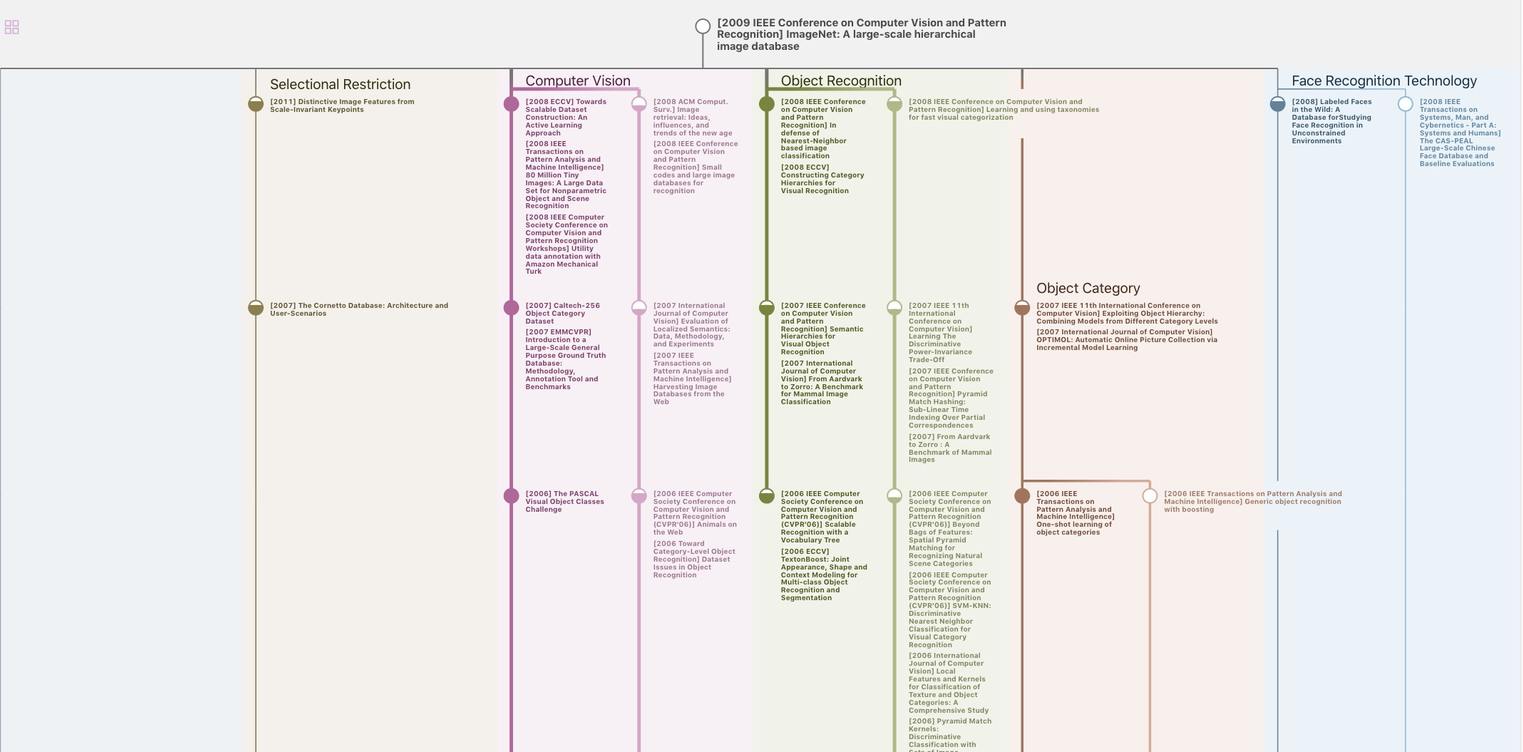
生成溯源树,研究论文发展脉络
Chat Paper
正在生成论文摘要