Proximal sensing provides clean, fast, and accurate quality control of organic and mineral fertilizers
ENVIRONMENTAL RESEARCH(2023)
摘要
Farms use large quantities of fertilizers from many sources, making quality control a challenging task, as the traditional wet-chemistry analyses are expensive, time consuming and not environmentally-friendly. As an alternative, this work proposes the use of portable X-ray fluorescence (pXRF) spectrometry and machine learning algorithms for rapid and low-cost estimation of macro and micronutrient contents in mineral and organic fertilizers. Four machine learning algorithms were tested. Whole (i.e., as delivered by the manufacturer) (CP) and ground (AQ) samples (429 in total) were analyzed to test the effect of fertilizer granulometry in prediction performance. Model validation indicated highly accurate predictions of macro (N: R2 = 0.92; P: 0.97; K: 0.99; Ca: 0.94, Mg: 0.98; S: 0.96) and micronutrients (B: 0.99; Cu: 0.99; Fe: 0.98; Mn: 0.91; Zn: 0.94) for both organic and mineral fertilizers. RPD values ranged from 2.31 to 9.23 for AQ samples, and Random Forest and Cubist Regression were the algorithms with the best performances. Even samples analyzed as they were received from the manufacturer (i.e., no grinding) provided accurate predictions, which accelerate the confirmation of nutrient contents contained in fertilizers. Results demonstrated the potential of pXRF data coupled with machine learning algorithms to assess nutrient composition in both mineral and organic fertilizers with high accuracy, allowing for clean, fast and accurate quality control. Sensor-driven quality assessment of fertilizers improves soil and plant health, crop management efficiency and food security with a reduced environmental footprint.
更多查看译文
关键词
pXRF,Machine learning,Green analysis,Soil health,Soil contamination,Macronutrients,Micronutrients
AI 理解论文
溯源树
样例
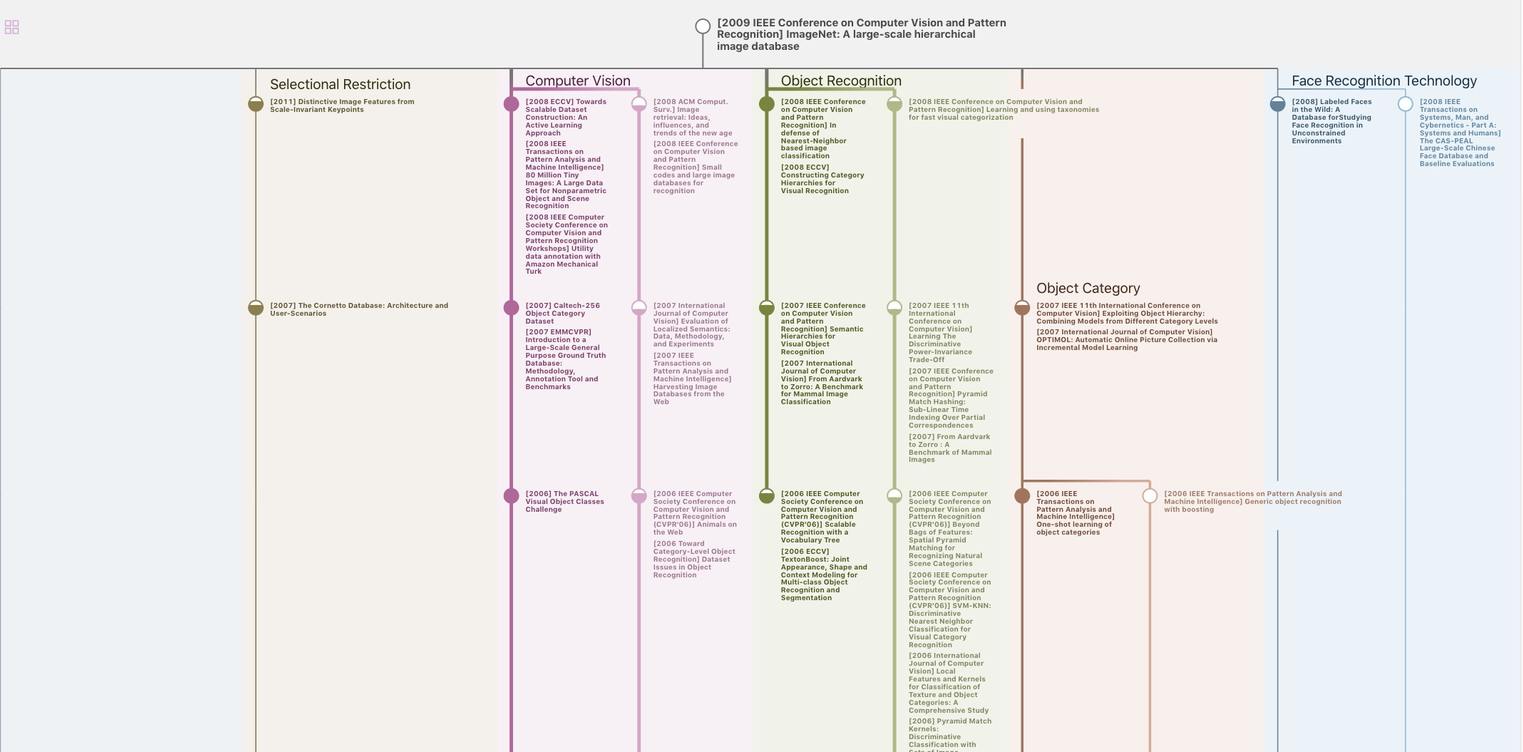
生成溯源树,研究论文发展脉络
Chat Paper
正在生成论文摘要