STAR-FC: Structure-Aware Face Clustering on Ultra-Large-Scale Graphs
IEEE transactions on pattern analysis and machine intelligence(2023)
摘要
Face clustering is a promising method for annotating unlabeled face images. Recent supervised approaches have boosted the face clustering accuracy greatly, however their performance is still far from satisfactory. These methods can be roughly divided into global-based and local-based ones. Global-based methods suffer from the limitation of training data scale, while local-based ones are inefficient for inference due to the use of numerous overlapped subgraphs. Previous approaches fail to tackle these two challenges simultaneously. To address the dilemma of large-scale training and efficient inference, we propose the STructure-AwaRe Face Clustering (STAR-FC) method. Specifically, we design a structure-preserving subgraph sampling strategy to explore the power of large-scale training data, which can increase the training data scale from
${10^{5}}$
to
${10^{7}}$
. On this basis, a novel hierarchical GCN training paradigm is further proposed for better capturing the dynamic local structure. During inference, the STAR-FC performs efficient full-graph clustering with two steps: graph parsing and graph refinement. And the concept of node intimacy is introduced in the second step to mine the local structural information, where a calibration module is further proposed for fairer edge scores. The STAR-FC gets 93.21 pairwise F-score on standard partial MS1M within 312 seconds, which far surpasses the state-of-the-arts while maintaining high inference efficiency. Furthermore, we are the first to train on an ultra-large-scale graph with 20 M nodes, and achieve superior inference results on 12 M testing data. Overall, as a simple and effective method, the proposed STAR-FC provides a strong baseline for large-scale face clustering.
更多查看译文
关键词
Face clustering,large-scale graph,graph convolutional network,hierarchical GCN training,node intimacy
AI 理解论文
溯源树
样例
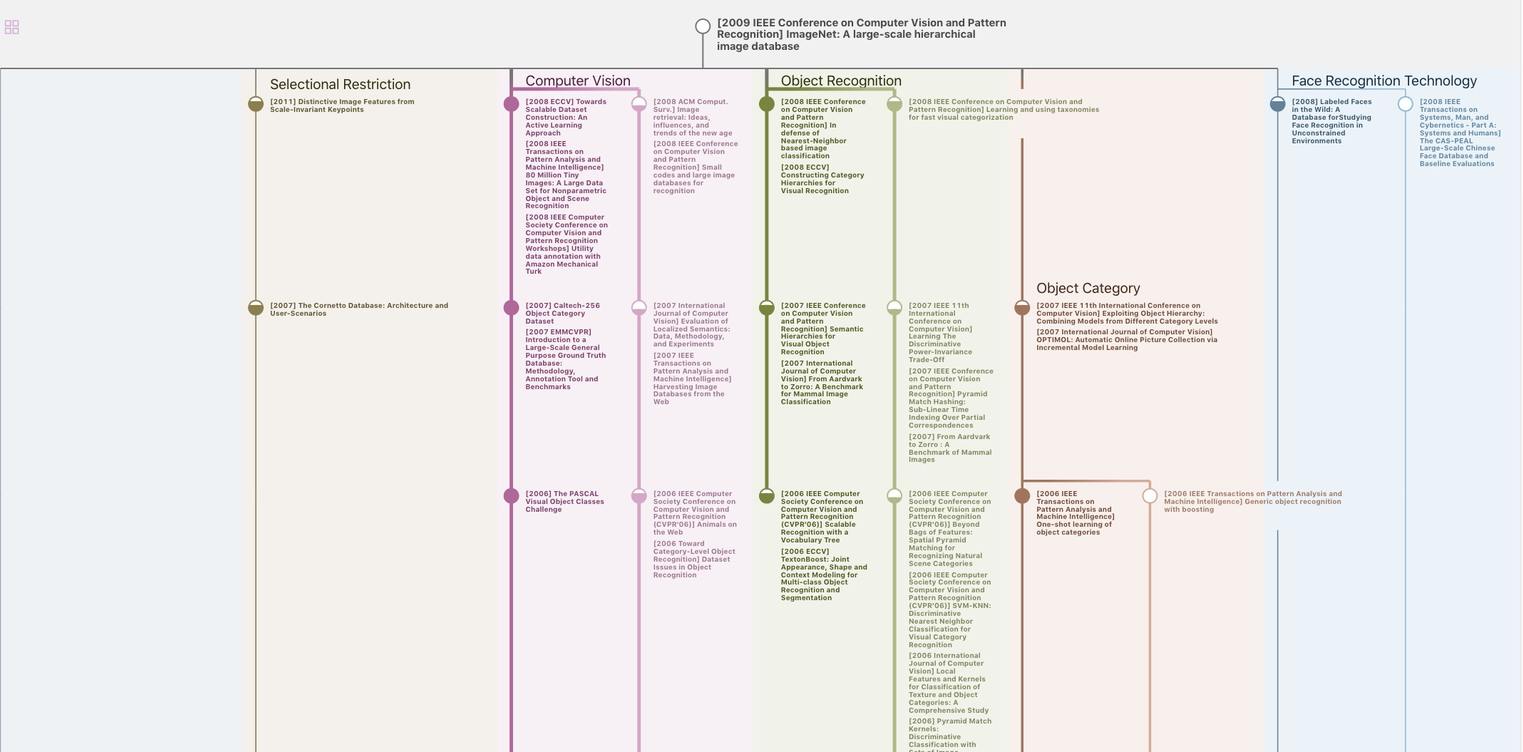
生成溯源树,研究论文发展脉络
Chat Paper
正在生成论文摘要