An 82-nW 0.53-pJ/SOP Clock-Free Spiking Neural Network With 40-s Latency for AIoT Wake-Up Functions Using a Multilevel-Event-Driven Bionic Architecture and Computing-in-Memory Technique
IEEE Transactions on Circuits and Systems I: Regular Papers(2023)
摘要
This article presents a clock-free spiking neural network (SNN) intelligent inference engine (IIE) for artificial intelligence of things (AIoT) sensor nodes, which often operate in random-sparse-event (RSE) scenarios. The IIE drastically reduces the system's long-term average (LTA) power consumption, improves energy efficiency, and achieves microsecond level inference latency. Three techniques are proposed: 1) A clockfree SNN architecture without clock tree, frame generator, and arbiter, is driven by the output spikes, which are encoded with level-crossing (LC) sampling method; the circuit activity is completely related to event activity and spike rates, dramatically reducing the overall power consumption and latency. 2) The bioinspired leaky-integrate-fire (LIF) neurons directly extract the time-domain information from asynchronous spikes, reducing the network size and number of operations. 3) The computing-in-memory (CIM) and mixed-signal synapse-neuron circuits are employed to increase the SNN parallelism and avoid weight movements, thus improving the energy efficiency and response speed. The measured LTA power is bounded at 82 nW while the event-driven chip is on call and waiting for events; the energy efficiency is 0.53 pJ per synapse operation (SOP), only 1/3 that of state-of-the-art methods at 4bit weights even with 180 nm technology. We demonstrate electrocardiogram (ECG) recognition as a typical AIoT application, and the power consumption is less than 350 nW. The measured accuracy of abnormal ECG detection is 90.5%. Moreover, the latency is only 40 mu s to realize real-time NN inference. This work provides an effective solution for AIoT nodes that require both ultralow power and fast response.
更多查看译文
关键词
Neurons,Micromechanical devices,Feature extraction,Power demand,Behavioral sciences,Energy efficiency,Computer architecture,Spiking neural network (SNN),clock-free,event-driven architecture,computing in memory (CIM)
AI 理解论文
溯源树
样例
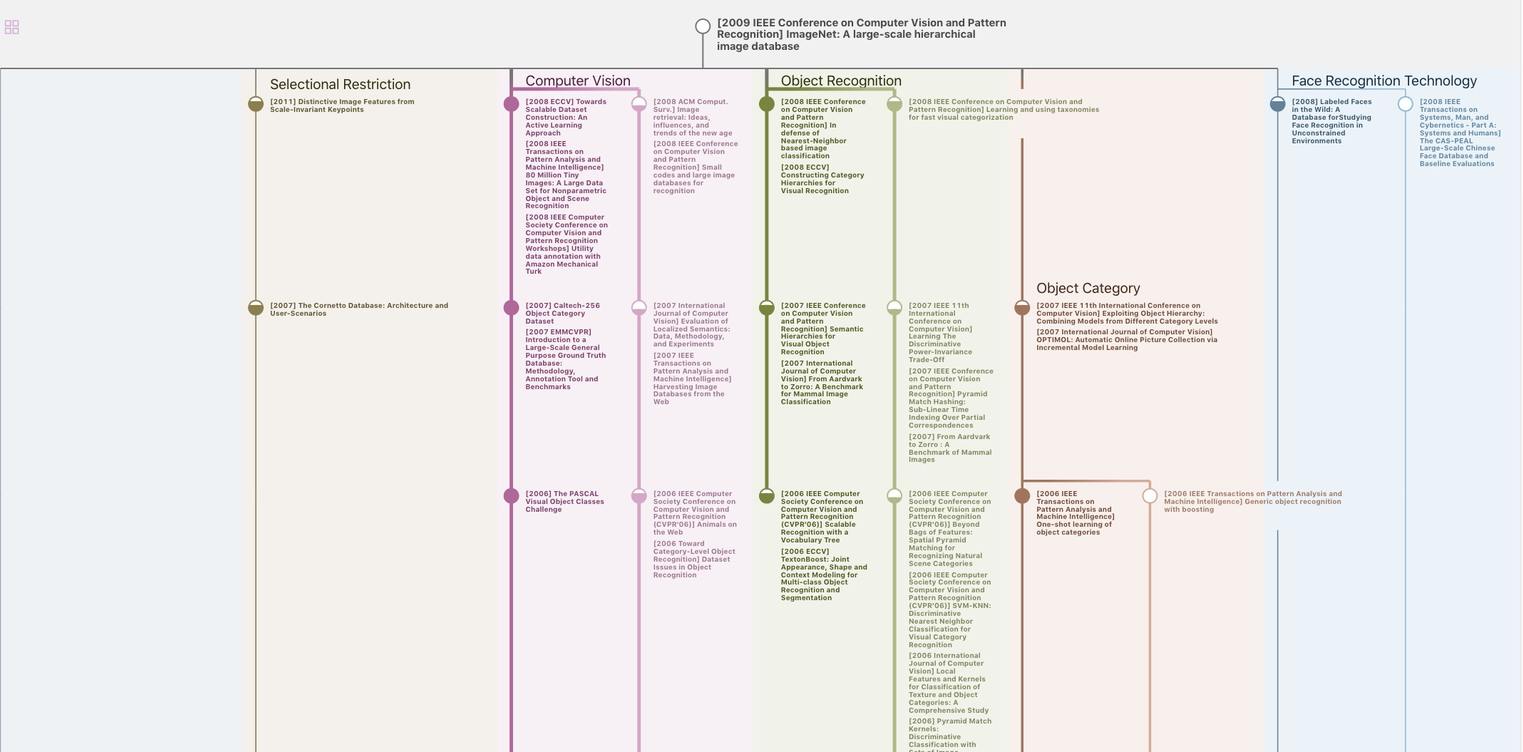
生成溯源树,研究论文发展脉络
Chat Paper
正在生成论文摘要