Machine-learned acceleration for molecular dynamics in CASTEP
JOURNAL OF CHEMICAL PHYSICS(2023)
摘要
Machine learning (ML) methods are of rapidly growing interest for materials modeling, and yet, the use of ML interatomic potentials for new systems is often more demanding than that of established density-functional theory (DFT) packages. Here, we describe computational methodology to combine the CASTEP first-principles simulation software with the on-the-fly fitting and evaluation of ML interatomic potential models. Our approach is based on regular checking against DFT reference data, which provides a direct measure of the accuracy of the evolving ML model. We discuss the general framework and the specific solutions implemented, and we present an example application to high-temperature molecular-dynamics simulations of carbon nanostructures. The code is freely available for academic research.
更多查看译文
关键词
molecular dynamics,castep,acceleration,machine-learned
AI 理解论文
溯源树
样例
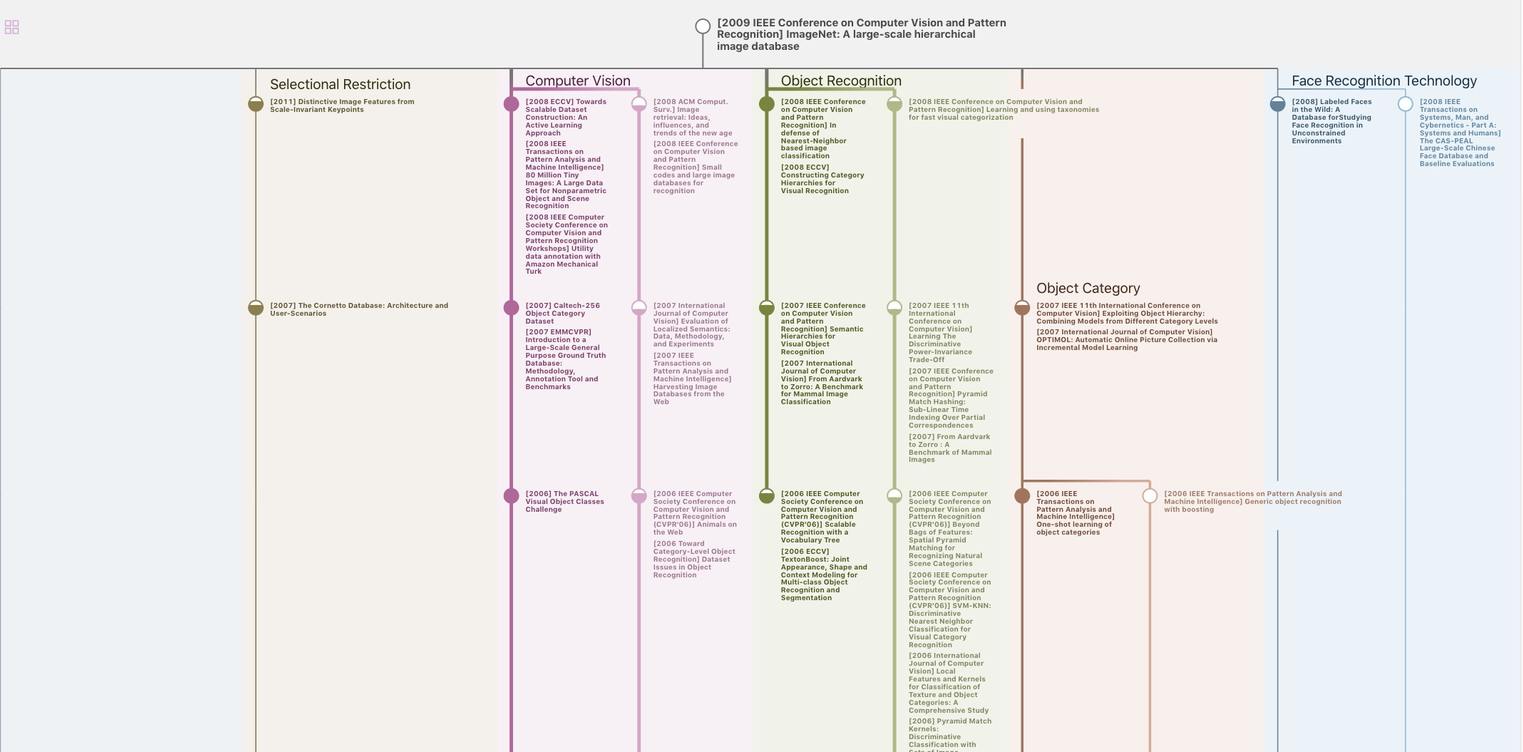
生成溯源树,研究论文发展脉络
Chat Paper
正在生成论文摘要