Image Synthesis and Modified BlendMask Instance Segmentation for Automated Nanoparticle Phenotyping
IEEE TRANSACTIONS ON MEDICAL IMAGING(2023)
摘要
Automated nanoparticle phenotyping is a critical aspect of high-throughput drug research, which requires analyzing nanoparticle size, shape, and surface topography from microscopy images. To automate this process, we present an instance segmentation pipeline that partitions individual nanoparticles on microscopy images. Our pipeline makes two key contributions. Firstly, we synthesize diverse and approximately realistic nanoparticle images to improve robust learning. Secondly, we improve the BlendMask model to segment tiny, overlapping, or sparse particle images. Specifically, we propose a parameterized approach for generating novel pairs of single particles and their masks, encouraging greater diversity in the training data. To synthesize more realistic particle images, we explore three particle placement rules and an image selection criterion. The improved one-stage instance segmentation network extracts distinctive features of nanoparticles and their context at both local and global levels, which addresses the data challenges associated with tiny, overlapping, or sparse nanoparticles. Extensive experiments demonstrate the effectiveness of our pipeline for automating nanoparticle partitioning and phenotyping in drug research using microscopy images.
更多查看译文
关键词
Image segmentation,Nanoparticles,Image synthesis,Feature extraction,Pipelines,Task analysis,Scanning electron microscopy,Microscope image synthesis,instance segmentation,deep learning,nanoparticle phenotyping
AI 理解论文
溯源树
样例
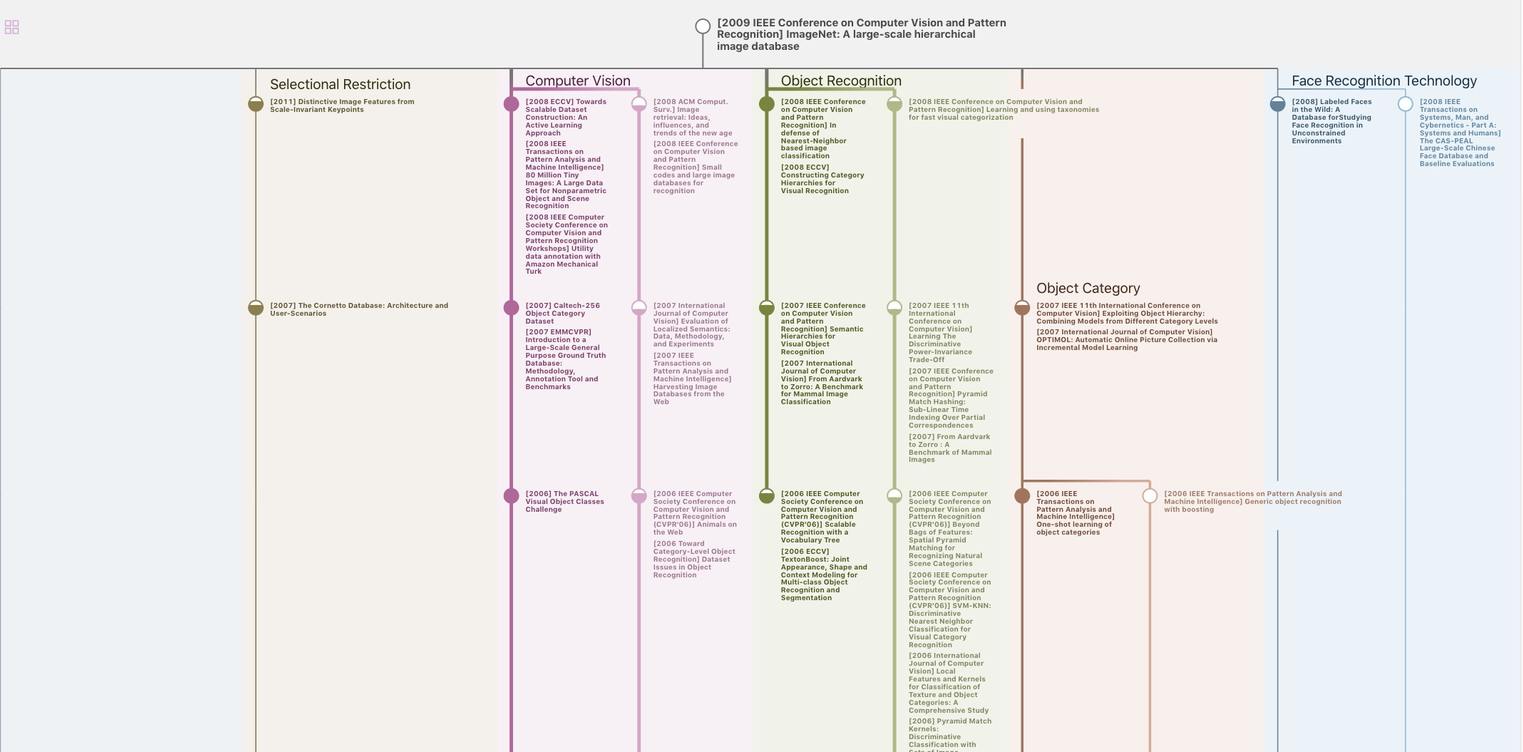
生成溯源树,研究论文发展脉络
Chat Paper
正在生成论文摘要