The RoboDepth Challenge: Methods and Advancements Towards Robust Depth Estimation
CoRR(2023)
摘要
Accurate depth estimation under out-of-distribution (OoD) scenarios, such as adverse weather conditions, sensor failure, and noise contamination, is desirable for safety-critical applications. Existing depth estimation systems, however, suffer inevitably from real-world corruptions and perturbations and are struggled to provide reliable depth predictions under such cases. In this paper, we summarize the winning solutions from the RoboDepth Challenge -- an academic competition designed to facilitate and advance robust OoD depth estimation. This challenge was developed based on the newly established KITTI-C and NYUDepth2-C benchmarks. We hosted two stand-alone tracks, with an emphasis on robust self-supervised and robust fully-supervised depth estimation, respectively. Out of more than two hundred participants, nine unique and top-performing solutions have appeared, with novel designs ranging from the following aspects: spatial- and frequency-domain augmentations, masked image modeling, image restoration and super-resolution, adversarial training, diffusion-based noise suppression, vision-language pre-training, learned model ensembling, and hierarchical feature enhancement. Extensive experimental analyses along with insightful observations are drawn to better understand the rationale behind each design. We hope this challenge could lay a solid foundation for future research on robust and reliable depth estimation and beyond. The datasets, competition toolkit, workshop recordings, and source code from the winning teams are publicly available on the challenge website.
更多查看译文
关键词
robust robodepth,robodepth challenge
AI 理解论文
溯源树
样例
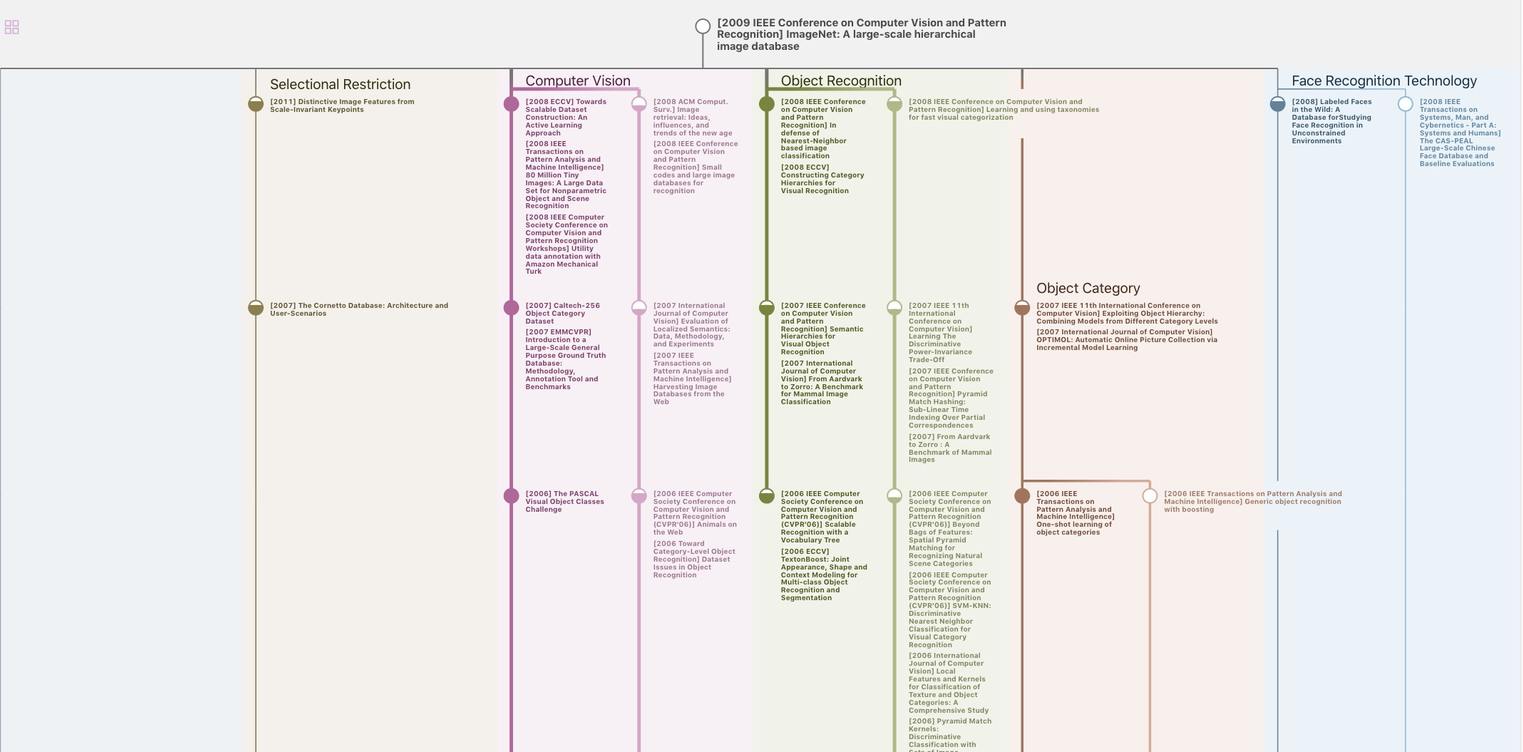
生成溯源树,研究论文发展脉络
Chat Paper
正在生成论文摘要