Matching Patients to Clinical Trials with Large Language Models
arxiv(2023)
摘要
Clinical trials are often hindered by the challenge of patient recruitment.
In this work, we introduce TrialGPT, a first-of-its-kind large language model
(LLM) framework to assist patient-to-trial matching. Given a patient note,
TrialGPT predicts the patient's eligibility on a criterion-by-criterion basis
and then consolidates these predictions to assess the patient's eligibility for
the target trial. We evaluate the trial-level prediction performance of
TrialGPT on three publicly available cohorts of 184 patients with over 18,000
trial annotations. We also engaged three physicians to label over 1,000
patient-criterion pairs to assess its criterion-level prediction accuracy.
Experimental results show that TrialGPT achieves a criterion-level accuracy of
87.3
(88.7
eligibility judgments, and they outperform the best-competing models by 32.6
to 57.2
reveals that TrialGPT can significantly reduce the screening time (by 42.6
a real-life clinical trial matching task. These results and analyses have
demonstrated promising opportunities for clinical trial matching with LLMs such
as TrialGPT.
更多查看译文
AI 理解论文
溯源树
样例
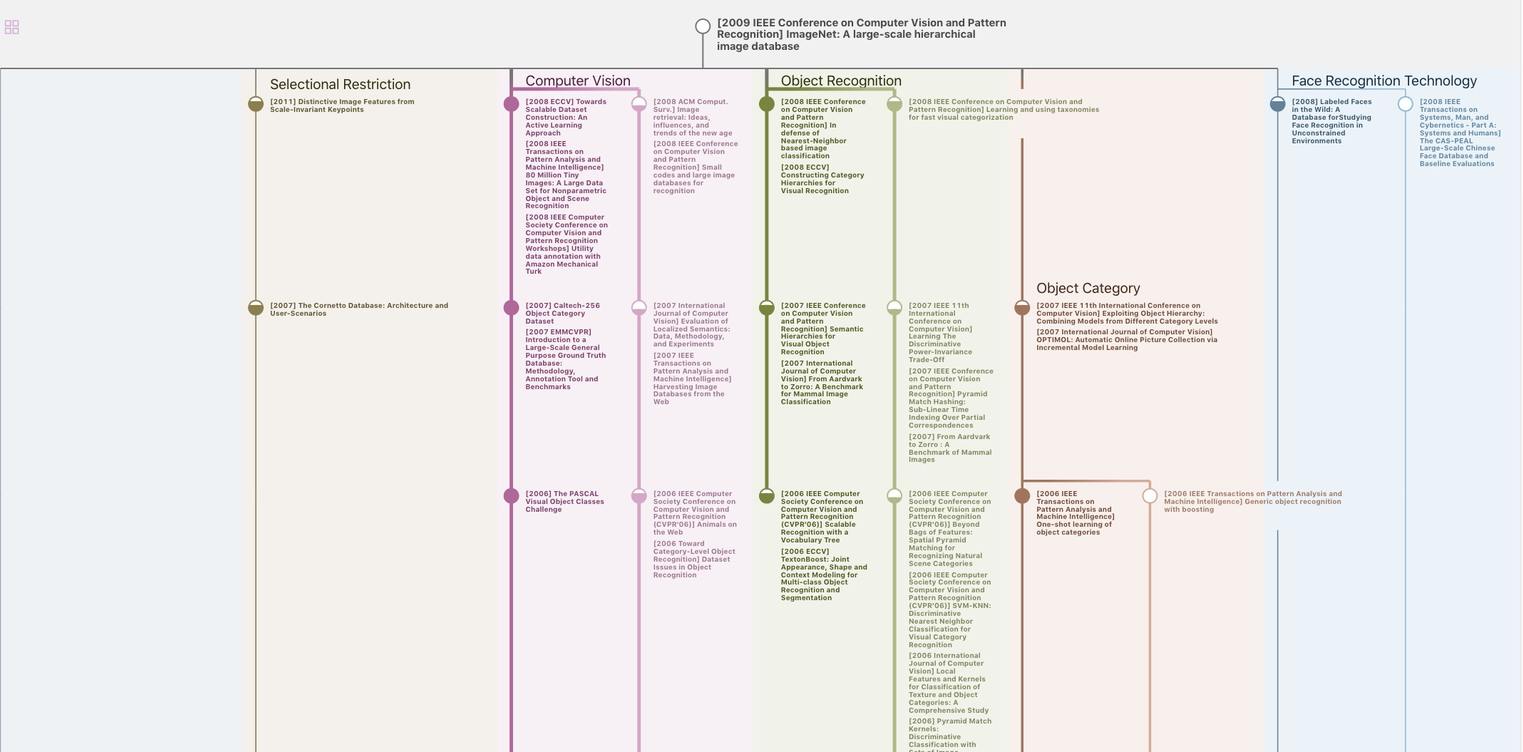
生成溯源树,研究论文发展脉络
Chat Paper
正在生成论文摘要