Optimal Estimation in Mixed-Membership Stochastic Block Models
CoRR(2023)
摘要
Community detection is one of the most critical problems in modern network science. Its applications can be found in various fields, from protein modeling to social network analysis. Recently, many papers appeared studying the problem of overlapping community detection, where each node of a network may belong to several communities. In this work, we consider Mixed-Membership Stochastic Block Model (MMSB) first proposed by Airoldi et al. (2008). MMSB provides quite a general setting for modeling overlapping community structure in graphs. The central question of this paper is to reconstruct relations between communities given an observed network. We compare different approaches and establish the minimax lower bound on the estimation error. Then, we propose a new estimator that matches this lower bound. Theoretical results are proved under fairly general conditions on the considered model. Finally, we illustrate the theory in a series of experiments.
更多查看译文
关键词
optimal estimation,stochastic,block,mixed-membership
AI 理解论文
溯源树
样例
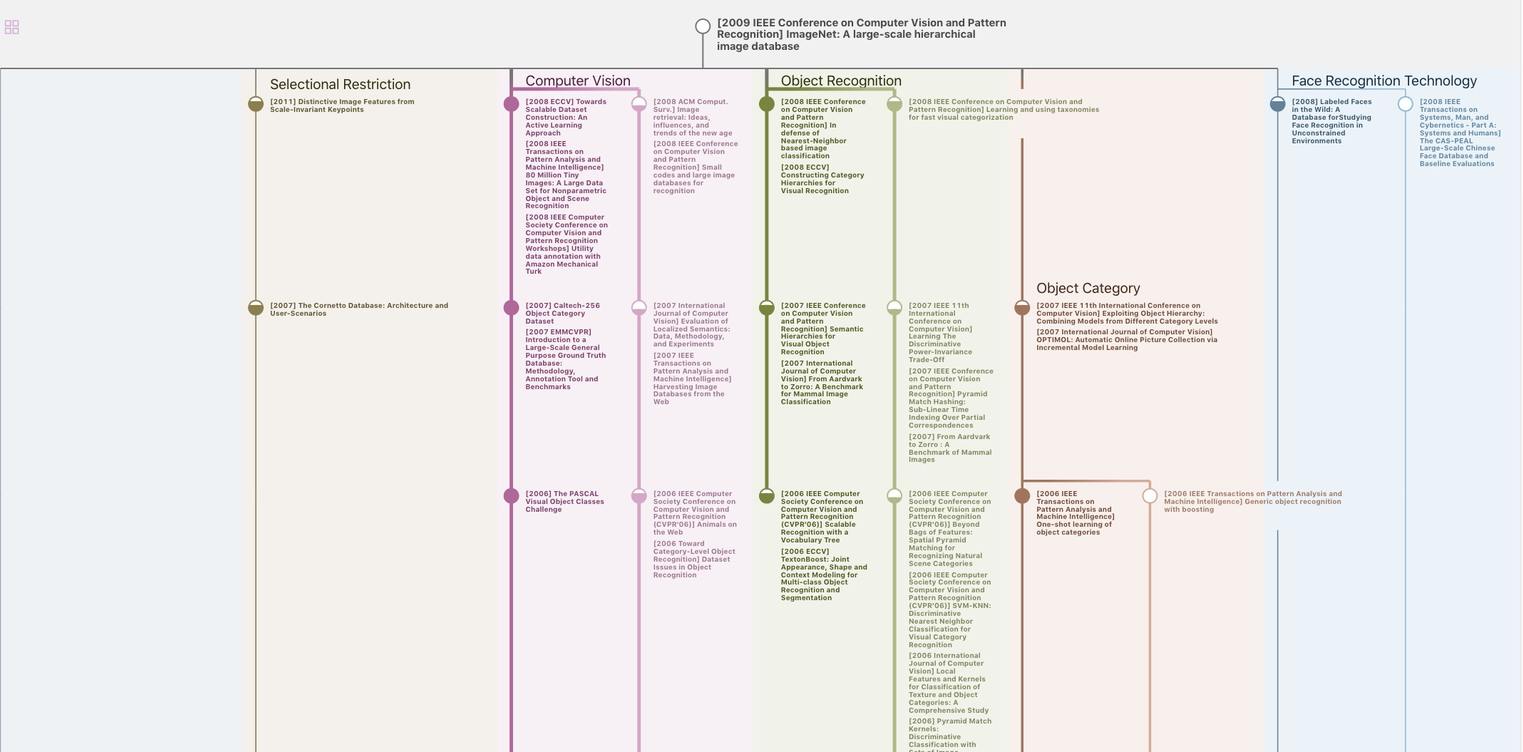
生成溯源树,研究论文发展脉络
Chat Paper
正在生成论文摘要