Limits to Reservoir Learning
CoRR(2023)
摘要
In this work, we bound a machine's ability to learn based on computational limitations implied by physicality. We start by considering the information processing capacity (IPC), a normalized measure of the expected squared error of a collection of signals to a complete basis of functions. We use the IPC to measure the degradation under noise of the performance of reservoir computers, a particular kind of recurrent network, when constrained by physical considerations. First, we show that the IPC is at most a polynomial in the system size $n$, even when considering the collection of $2^n$ possible pointwise products of the $n$ output signals. Next, we argue that this degradation implies that the family of functions represented by the reservoir requires an exponential number of samples to learn in the presence of the reservoir's noise. Finally, we conclude with a discussion of the performance of the same collection of $2^n$ functions without noise when being used for binary classification.
更多查看译文
关键词
reservoir,learning,limits
AI 理解论文
溯源树
样例
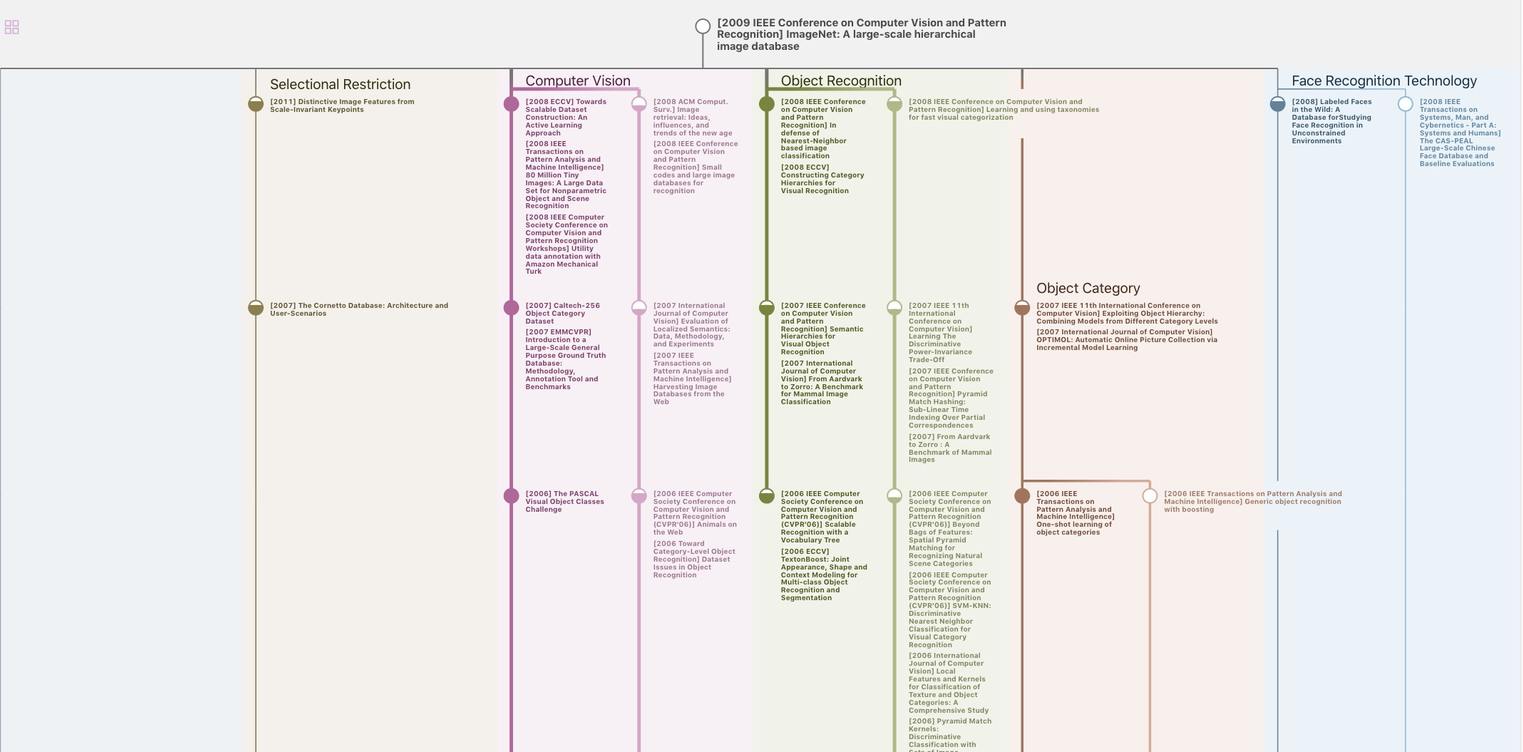
生成溯源树,研究论文发展脉络
Chat Paper
正在生成论文摘要