Comparison of Si-GA-PLS and Si-CARS-PLS build algorithms for quantitation of total polyphenols in black tea using the spectral analytical system
JOURNAL OF THE SCIENCE OF FOOD AND AGRICULTURE(2023)
摘要
BACKGROUNDThe objective of the current study was to compare two machine learning approaches for the quantification of total polyphenols by choosing the optimal spectral intervals utilizing the synergy interval partial least squares (Si-PLS) model. To increase the resilience of built models, the genetic algorithm (GA) and competitive adaptive reweighted sampling (CARS) were applied to a subset of variables. RESULTSThe collected spectral data were divided into 19 sub-interval selections totaling 246 variables, yielding the lowest root mean square error of cross-validation (RMSECV). The performance of the model was evaluated using the correlation coefficient for calibration (R-C), prediction (R-P), RMSECV, root mean square error of prediction (RMSEP) and residual predictive deviation (RPD) value. The Si-GA-PLS model produced the following results: PCs = 9; R-C = 0.915; RMSECV = 1.39; R-P = 0.8878; RMSEP = 1.62; and RPD = 2.32. The performance of the Si-CARS-PLS model was noted to be best at PCs = 10, while R-C = 0.9723, RMSECV = 0.81, R-P = 0.9114, RMSEP = 1.45 and RPD = 2.59. CONCLUSIONThe build model's prediction ability was amended in the order PLS < Si-PLS < CARS-PLS when full spectroscopic data were used and Si-PLS < Si-GA-PLS < Si-CARS-PLS when interval selection was performed with the Si-PLS model. Finally, the developed method was successfully used to quantify total polyphenols in tea. & COPY; 2023 Society of Chemical Industry.
更多查看译文
关键词
total polyphenol, Si-GA-PLS, Si-CARS-PLS, variable selection, black tea
AI 理解论文
溯源树
样例
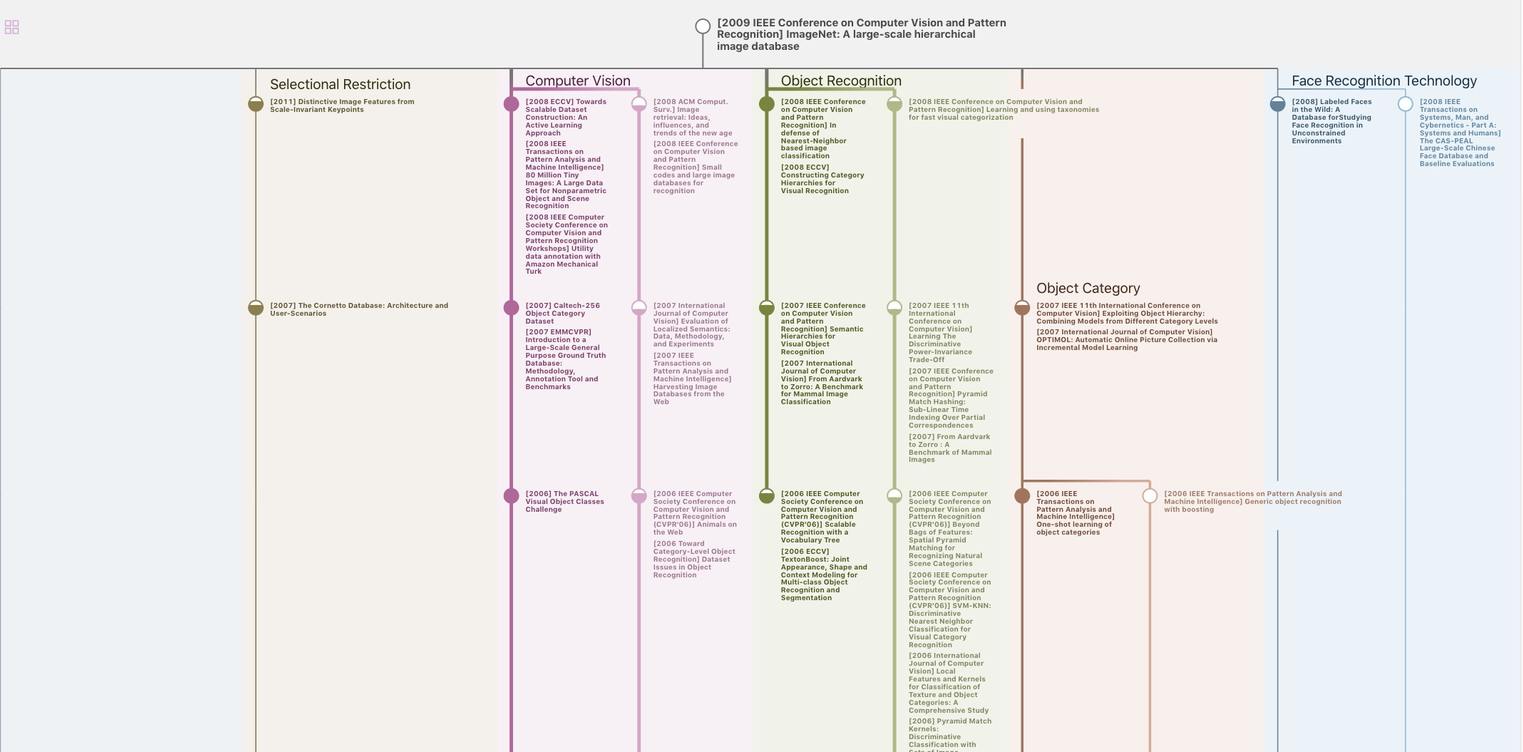
生成溯源树,研究论文发展脉络
Chat Paper
正在生成论文摘要