Convergence Analysis of Randomized SGDA under NC-PL Condition for Stochastic Minimax Optimization Problems
arXiv (Cornell University)(2023)
摘要
We introduce a new analytic framework to analyze the convergence of the Randomized Stochastic Gradient Descent Ascent (RSGDA) algorithm for stochastic minimax optimization problems. Under the so-called NC-PL condition on one of the variables, our analysis improves the state-of-the-art convergence results in the current literature and hence broadens the applicable range of the RSGDA. We also introduce a simple yet effective strategy to accelerate RSGDA , and empirically validate its efficiency on both synthetic data and real data.
更多查看译文
关键词
randomized sgda,stochastic,optimization
AI 理解论文
溯源树
样例
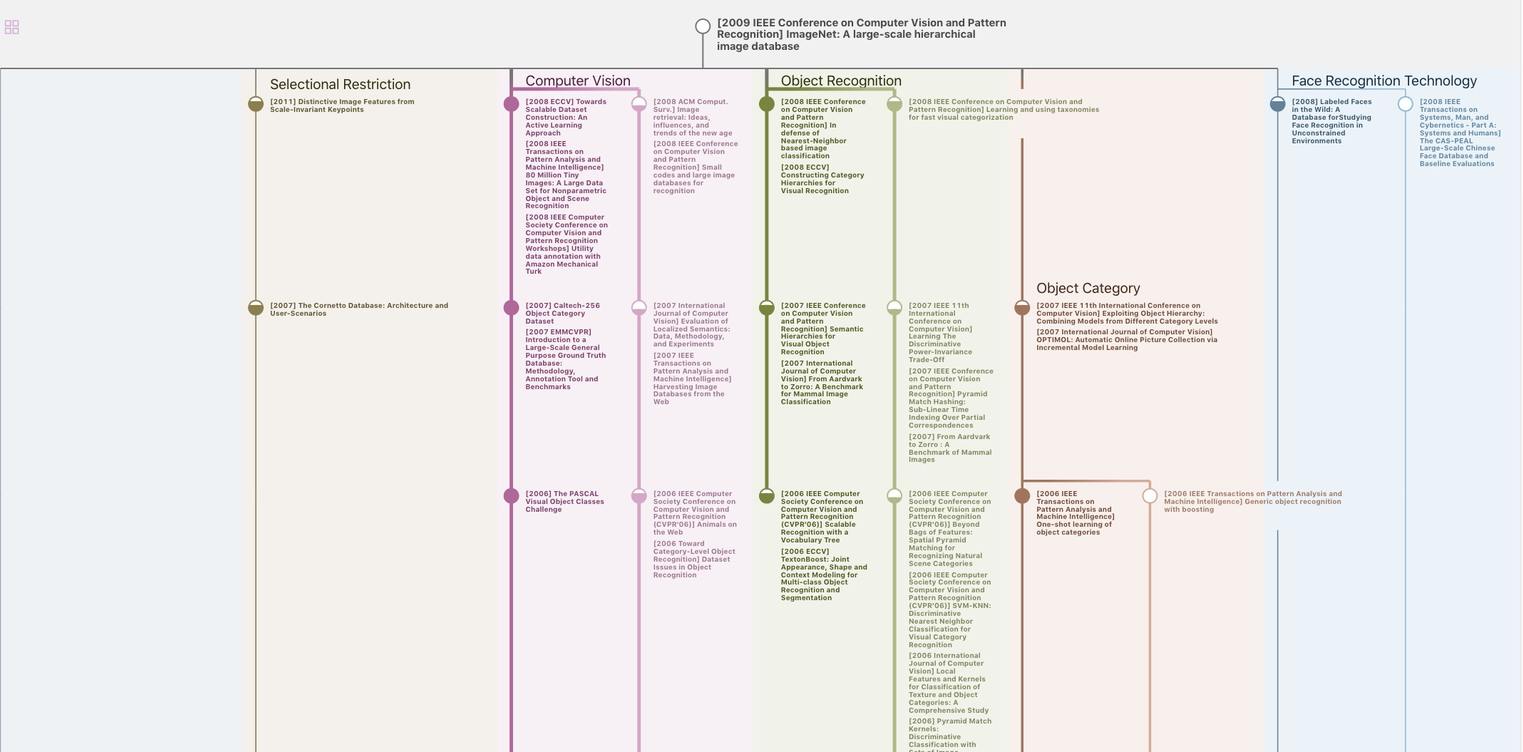
生成溯源树,研究论文发展脉络
Chat Paper
正在生成论文摘要