A Lightweight Pre-Crash Occupant Injury Prediction Model Distills Knowledge From Its Post-Crash Counterpart
JOURNAL OF BIOMECHANICAL ENGINEERING-TRANSACTIONS OF THE ASME(2024)
摘要
Accurate occupant injury prediction in near-collision scenarios is vital in guiding intelligent vehicles to find the optimal collision condition with minimal injury risks. Existing studies focused on boosting prediction performance by introducing deep-learning models but encountered computational burdens due to the inherent high model complexity. To better balance these two traditionally contradictory factors, this study proposed a training method for pre-crash injury prediction models, namely, knowledge distillation (KD)-based training. This method was inspired by the idea of knowledge distillation, an emerging model compression method. Technically, we first trained a high-accuracy injury prediction model using informative post-crash sequence inputs (i.e., vehicle crash pulses) and a relatively complex network architecture as an experienced "teacher". Following this, a lightweight pre-crash injury prediction model ("student") learned both from the ground truth in output layers (i.e., conventional prediction loss) and its teacher in intermediate layers (i.e., distillation loss). In such a step-by-step teaching framework, the pre-crash model significantly improved the prediction accuracy of occupant's head abbreviated injury scale (AIS) (i.e., from 77.2% to 83.2%) without sacrificing computational efficiency. Multiple validation experiments proved the effectiveness of the proposed KD-based training framework. This study is expected to provide reference to balancing prediction accuracy and computational efficiency of pre-crash injury prediction models, promoting the further safety improvement of next-generation intelligent vehicles.
更多查看译文
关键词
motor vehicle crashes,occupant protection,injury prediction,knowledge distillation,intelligent vehicles
AI 理解论文
溯源树
样例
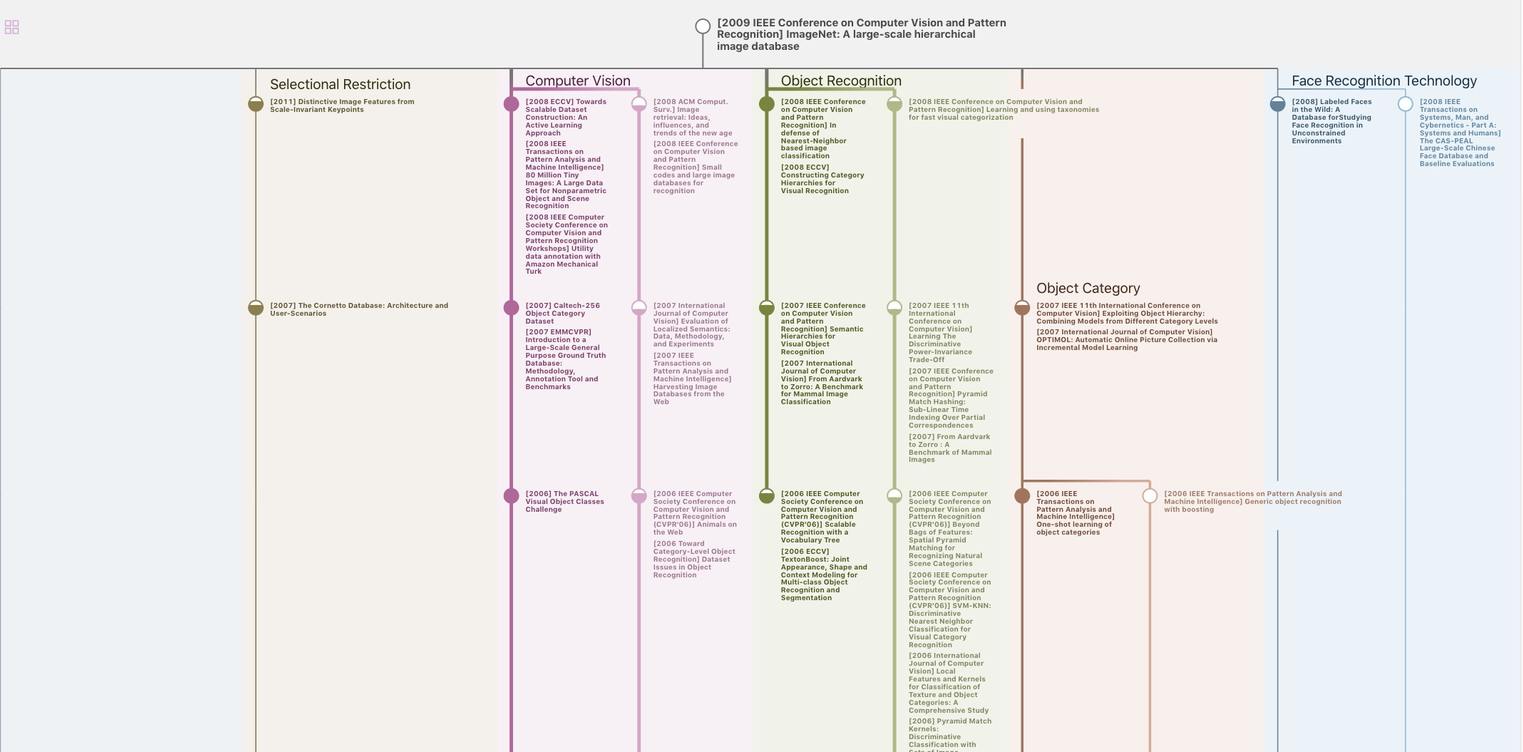
生成溯源树,研究论文发展脉络
Chat Paper
正在生成论文摘要