Multi-View Clustering With Graph Learning for scRNA-Seq Data
IEEE-ACM TRANSACTIONS ON COMPUTATIONAL BIOLOGY AND BIOINFORMATICS(2023)
摘要
Advances in single-cell biotechnologies have generated the single-cell RNA sequencing (scRNA-seq) of gene expression profiles at cell levels, providing an opportunity to study cellular distribution. Although significant efforts developed in their analysis, many problems remain in studying cell types distribution because of the heterogeneity, high dimensionality, and noise of scRNA-seq. In this study, a multi-view clustering with graph learning algorithm (MCGL) for scRNA-seq data is proposed, which consists of multi-view learning, graph learning, and cell type clustering. In order to avoid a single feature space of scRNA-seq being inadequate to comprehensively characterize the functions of cells, MCGL constructs the multiple feature spaces and utilizes multi-view learning to comprehensively characterize scRNA-seq data from different perspectives. MCGL adaptively learns the similarity graphs of cells that overcome the dependence on fixed similarity, transforming scRNA-seq analysis into the analysis of multi-view clustering. MCGL decomposes the networks of cells into view-specific and common networks in multi-view learning, which better characterizes the topological relationship of cells. MCGL simultaneously utilizes multiple types of cell-cell networks and fully exploits the connection relationship between cells through the complementarity between networks to improve clustering performance. The graph learning, graph factorization, and cell-type clustering processes are accomplished simultaneously under one optimization framework. The performance of the MCGL algorithm is validated with ten scRNA-seq datasets from different scales, and experimental results imply that the proposed algorithm significantly outperforms fourteen state-of-the-art scRNA-seq algorithms.
更多查看译文
关键词
Clustering algorithms,Feature extraction,Gene expression,Optimization,Graph neural networks,Periodic structures,Topology,Graph learning,multi-view clustering,non-negative matrix factorization,representation learning,ScRNA-seq data
AI 理解论文
溯源树
样例
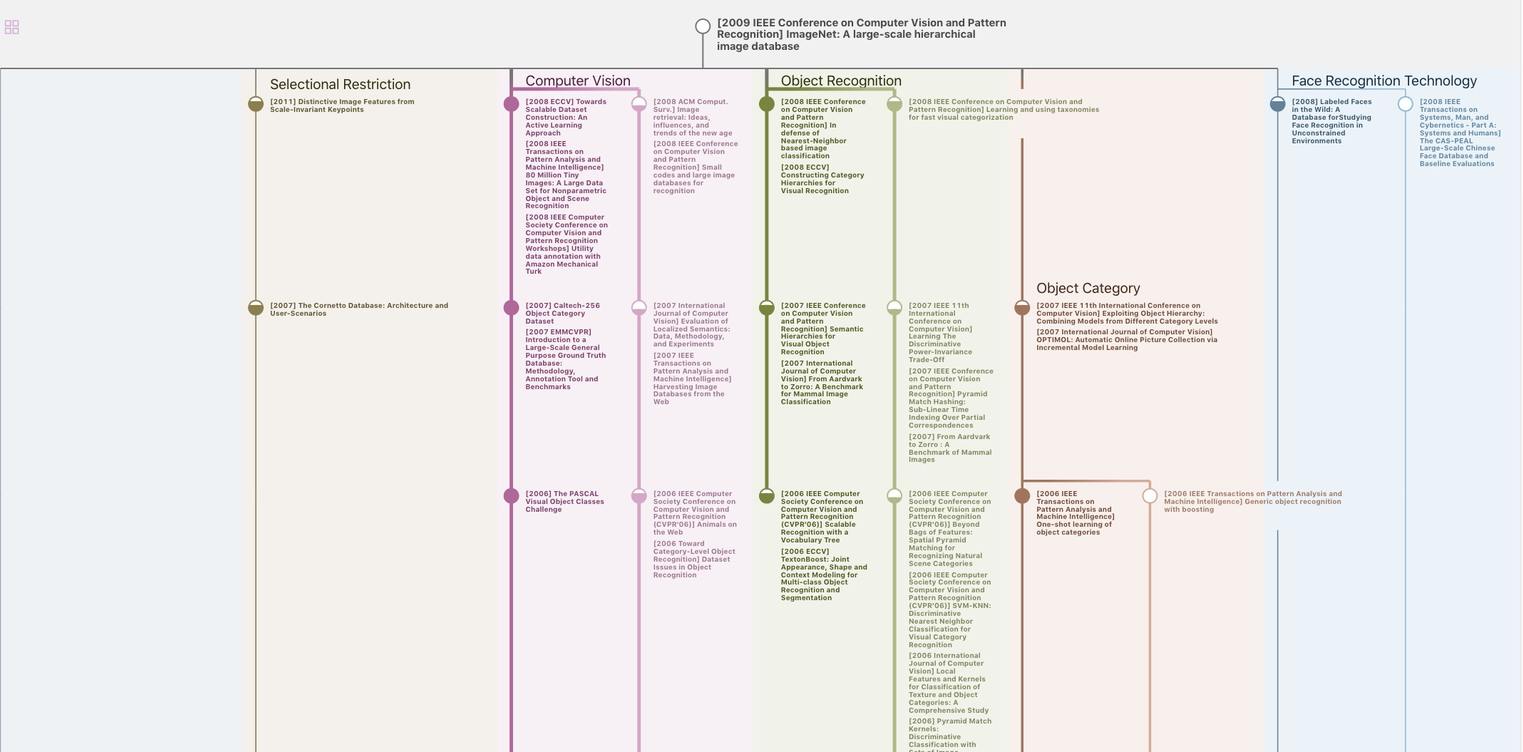
生成溯源树,研究论文发展脉络
Chat Paper
正在生成论文摘要