Self-Supervised Digital Histopathology Image Disentanglement for Arbitrary Domain Stain Transfer
IEEE TRANSACTIONS ON MEDICAL IMAGING(2023)
摘要
Diagnosis of cancerous diseases relies on digital histopathology images from stained slides. However, the staining varies among medical centers, which leads to a domain gap of staining. Existing generative adversarial network (GAN) based stain transfer methods highly rely on distinct domains of source and target, and cannot handle unseen domains. To overcome these obstacles, we propose a self-supervised disentanglement network (SDN) for domain-independent optimization and arbitrary domain stain transfer. SDN decomposes an image into features of content and stain. By exchanging the stain features, the staining style of an image is transferred to the target domain. For optimization, we propose a novel self-supervised learning policy based on the consistency of stain and content among augmentations from one instance. Therefore, the process of training SDN is independent on the domain of training data, and thus SDN is able to tackle unseen domains. Exhaustive experiments demonstrate that SDN achieves the top performance in intra-dataset and cross-dataset stain transfer compared with the state-of-the-art stain transfer models, while the number of parameters in SDN is three orders of magnitude smaller parameters than that of compared models. Through stain transfer, SDN improves AUC of downstream classification model on unseen data without fine-tuning. Therefore, the proposed disentanglement framework and self-supervised learning policy have significant advantages in eliminating the stain gap among multi-center histopathology images.
更多查看译文
关键词
Stain normalization,stain transfer,histopathological image analysis,digital pathology,computational pathology,self-supervised learning
AI 理解论文
溯源树
样例
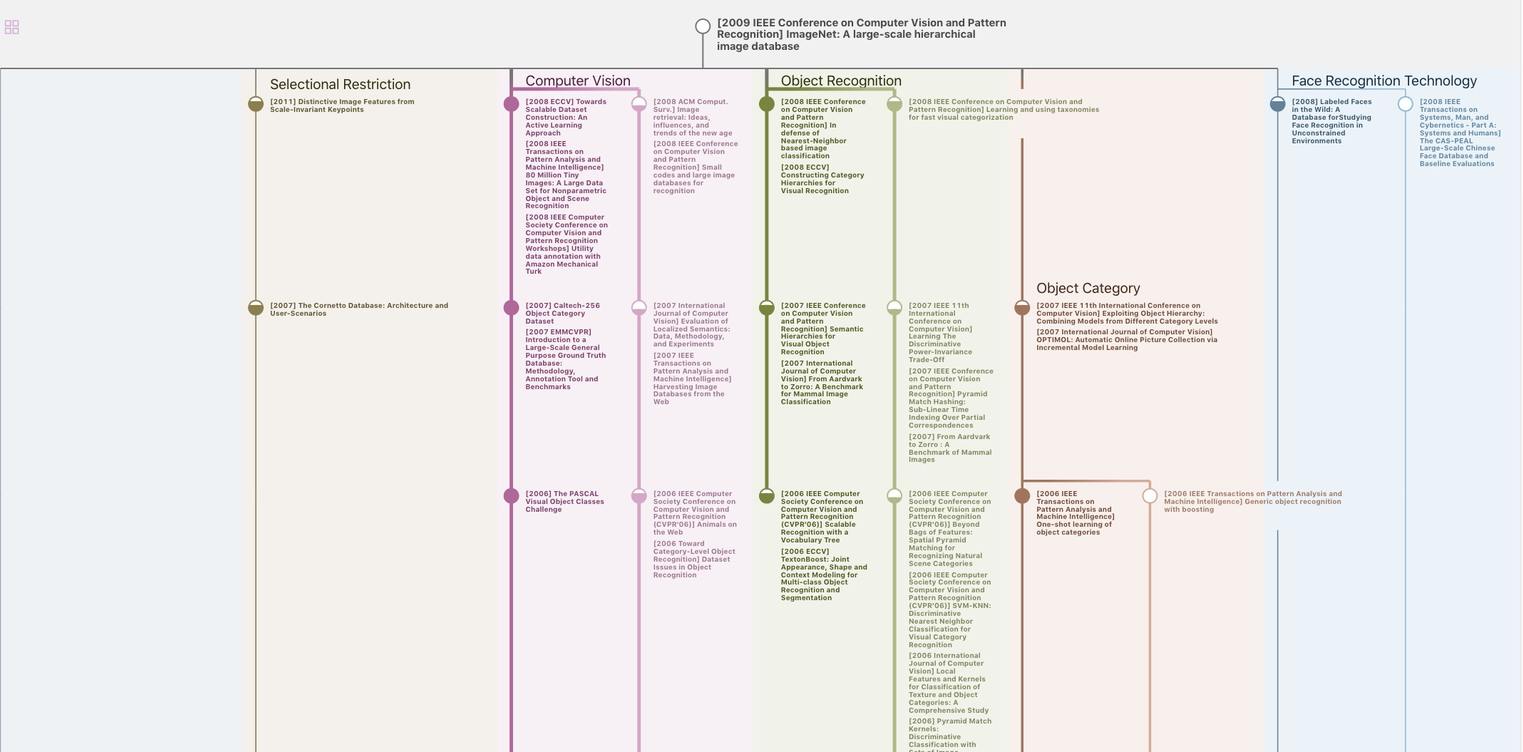
生成溯源树,研究论文发展脉络
Chat Paper
正在生成论文摘要