Anatomically Guided Cross-Domain Repair and Screening for Ultrasound Fetal Biometry.
IEEE journal of biomedical and health informatics(2023)
摘要
Ultrasound based estimation of fetal biometry is extensively used to diagnose prenatal abnormalities and to monitor fetal growth, for which accurate segmentation of the fetal anatomy is a crucial prerequisite. Although deep neural network-based models have achieved encouraging results on this task, inevitable distribution shifts in ultrasound images can still result in severe performance drop in real world deployment scenarios. In this paper, we propose a complete ultrasound fetal examination system to deal with this troublesome problem by repairing and screening the anatomically implausible results. Our system consists of three main components: a routine segmentation network, a fetal anatomical key points guided repair network, and a shape-coding based selective screener. Guided by the anatomical key points, our repair network has stronger cross-domain repair capabilities, which can substantially improve the outputs of the segmentation network. By quantifying the distance between an arbitrary segmentation mask to its corresponding anatomical shape class, the proposed shape-coding based selective screener can then effectively reject the entire implausible results that cannot be fully repaired. Extensive experiments demonstrate that our proposed framework has strong anatomical guarantee and outperforms other methods in three different cross-domain scenarios. We release source codes and datasets on our GitHub: https://github.com/gaojun0821/RepairNet.
更多查看译文
关键词
Fetal ultrasound, domain adaptation, segmen-tation, anatomical key points, Hu moment
AI 理解论文
溯源树
样例
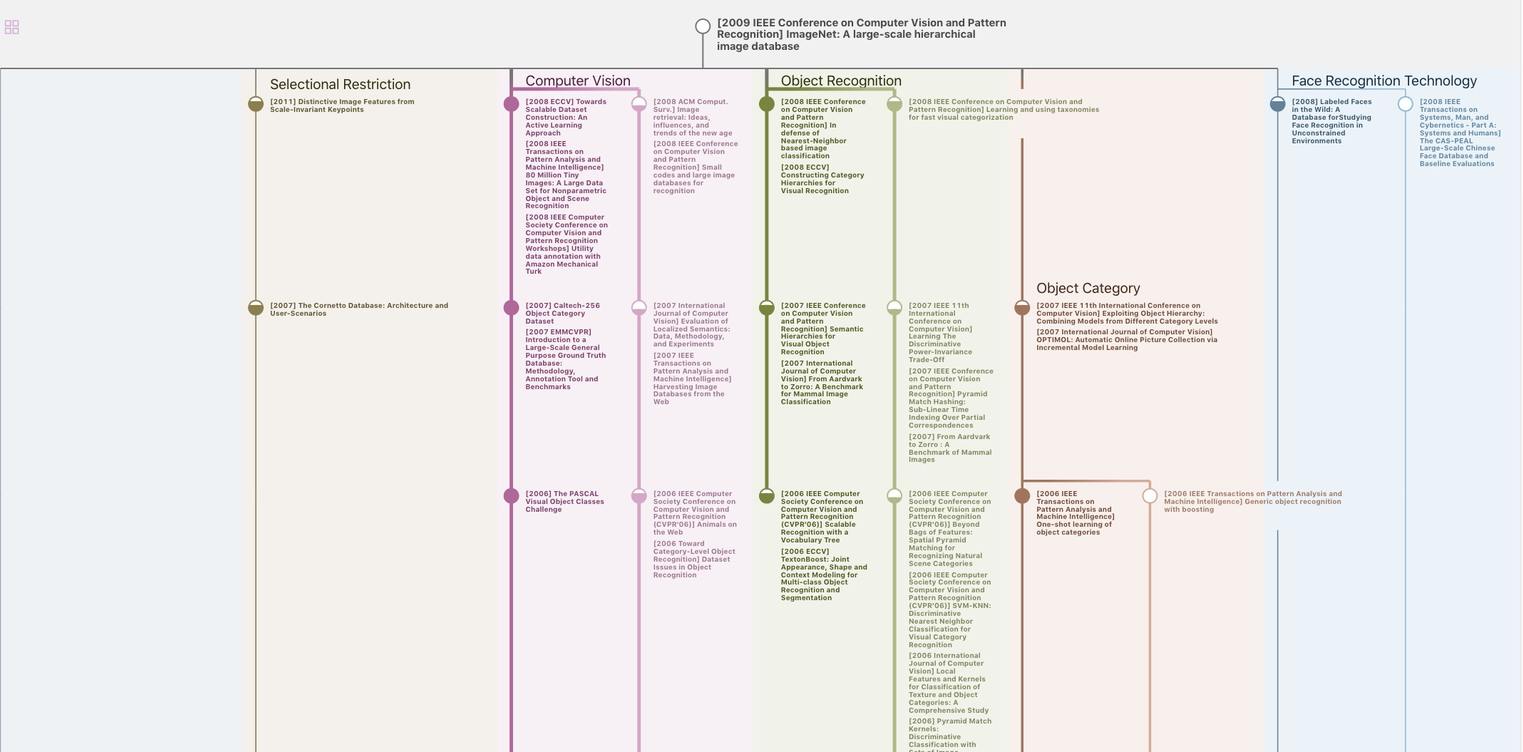
生成溯源树,研究论文发展脉络
Chat Paper
正在生成论文摘要