Retrieving the 21-cm signal from the Epoch of Reionization with learnt Gaussian process kernels
MONTHLY NOTICES OF THE ROYAL ASTRONOMICAL SOCIETY(2023)
摘要
Direct detection of the Cosmic Dawn and Epoch of Reionization via the redshifted 21-cm line of neutral Hydrogen will have unprecedented implications for studying structure formation in the early Universe. This exciting goal is challenged by the difficulty of extracting the faint 21-cm signal buried beneath bright astrophysical foregrounds and contaminated by numerous systematics. Here, we focus on improving the Gaussian Process Regression (GPR) signal separation method originally developed for LOFAR observations. We address a key limitation of the current approach by incorporating covariance prior models learnt from 21-cm signal simulations using variational autoencoder (VAE) and interpolatory autoencoder (IAE). Extensive tests are conducted to evaluate GPR, VAE-GPR, and IAE-GPR in different scenarios. Our findings reveal that the new method outperforms standard GPR in component separation tasks. Moreover, the improved method demonstrates robustness when applied to signals not represented in the training set. It also presents a certain degree of resilience to data systematics, highlighting its ability to effectively mitigate their impact on the signal recovery process. However, our findings also underscore the importance of accurately characterizing and understanding these systematics to achieve successful detection. Our generative approaches provide good results even with limited training data, offering a valuable advantage when a large training set is not feasible. Comparing the two algorithms, IAE-GPR shows slightly higher fidelity in recovering power spectra compared to VAE-GPR. These advancements highlight the strength of generative approaches and optimize the analysis techniques for future 21-cm signal detection at high redshifts.
更多查看译文
关键词
methods: data analysis,methods: statistical,techniques: interferometric,cosmology: observations,reionization,first stars
AI 理解论文
溯源树
样例
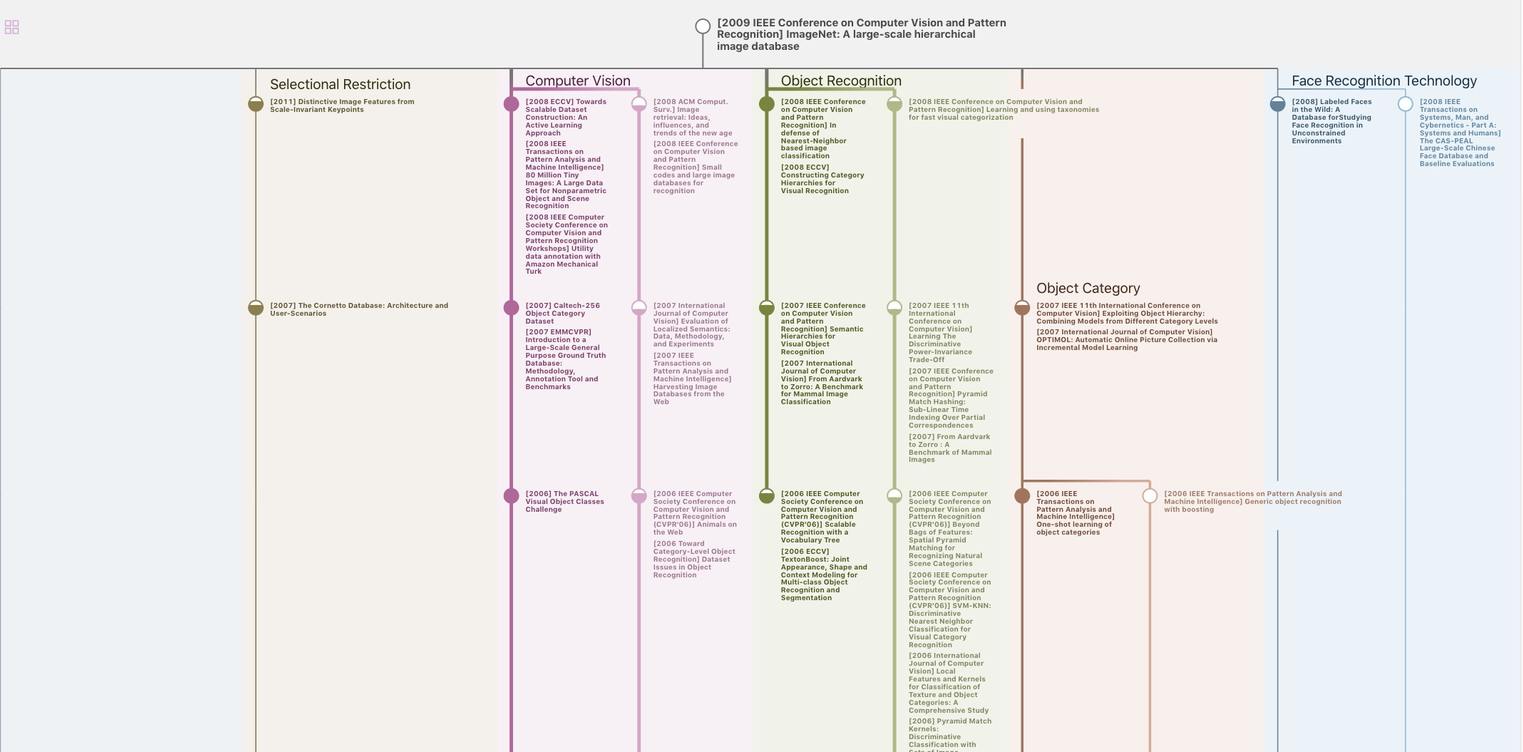
生成溯源树,研究论文发展脉络
Chat Paper
正在生成论文摘要