Integrating processed-based models and machine learning for crop yield prediction
CoRR(2023)
摘要
Crop yield prediction typically involves the utilization of either theory-driven process-based crop growth models, which have proven to be difficult to calibrate for local conditions, or data-driven machine learning methods, which are known to require large datasets. In this work we investigate potato yield prediction using a hybrid meta-modeling approach. A crop growth model is employed to generate synthetic data for (pre)training a convolutional neural net, which is then fine-tuned with observational data. When applied in silico, our meta-modeling approach yields better predictions than a baseline comprising a purely data-driven approach. When tested on real-world data from field trials (n=303) and commercial fields (n=77), the meta-modeling approach yields competitive results with respect to the crop growth model. In the latter set, however, both models perform worse than a simple linear regression with a hand-picked feature set and dedicated preprocessing designed by domain experts. Our findings indicate the potential of meta-modeling for accurate crop yield prediction; however, further advancements and validation using extensive real-world datasets is recommended to solidify its practical effectiveness.
更多查看译文
关键词
crop yield
AI 理解论文
溯源树
样例
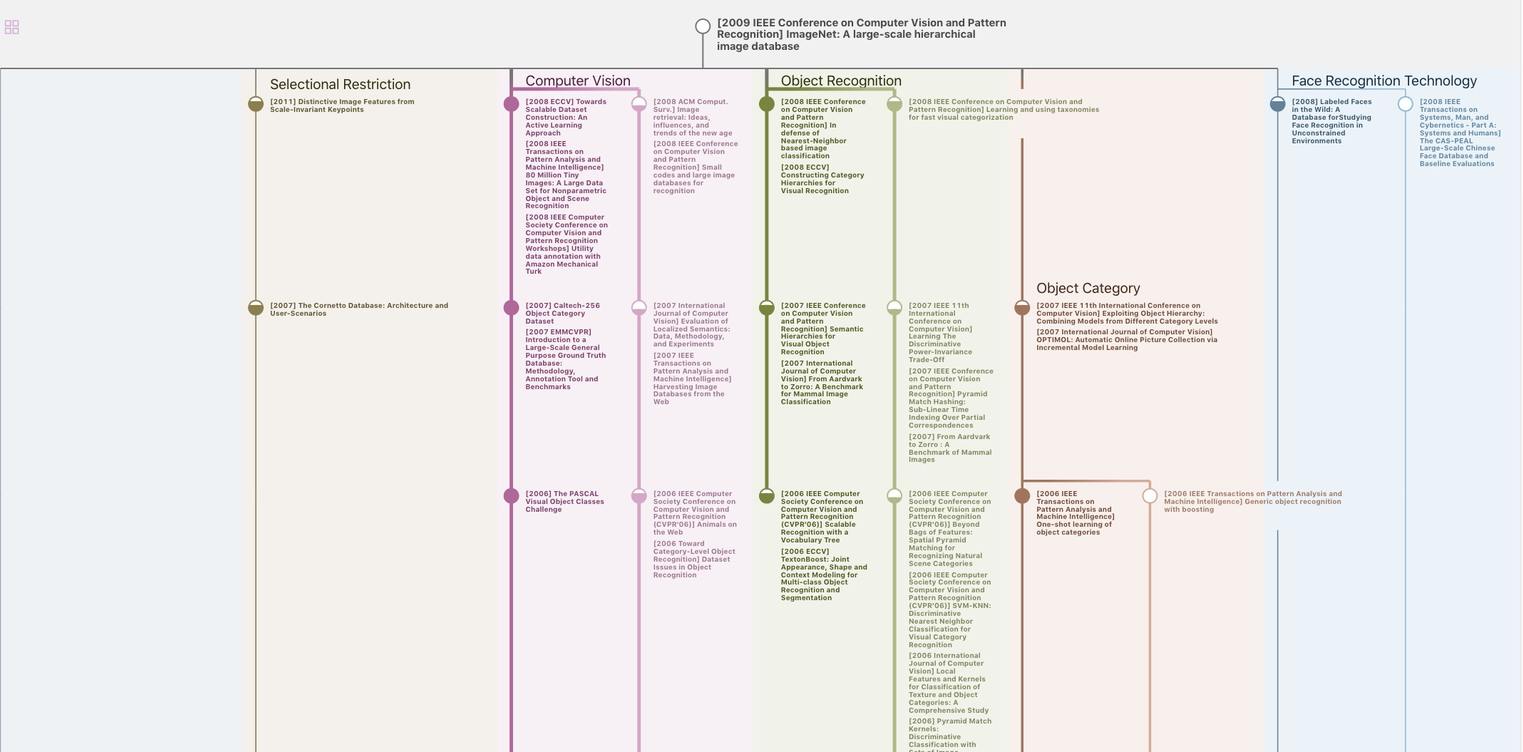
生成溯源树,研究论文发展脉络
Chat Paper
正在生成论文摘要