Network Traffic Classification based on Single Flow Time Series Analysis
2023 19TH INTERNATIONAL CONFERENCE ON NETWORK AND SERVICE MANAGEMENT, CNSM(2023)
摘要
Network traffic monitoring using IP flows is used to handle the current challenge of analyzing encrypted network communication. Nevertheless, the packet aggregation into flow records naturally causes information loss; therefore, this paper proposes a novel flow extension for traffic features based on the time series analysis of the Single Flow Time series, i.e., a time series created by the number of bytes in each packet and its timestamp. We propose 69 universal features based on the statistical analysis of data points, time domain analysis, packet distribution within the flow timespan, time series behavior, and frequency domain analysis. We have demonstrated the usability and universality of the proposed feature vector for various network traffic classification tasks using 15 well-known publicly available datasets. Our evaluation shows that the novel feature vector achieves classification performance similar or better than related works on both binary and multiclass classification tasks. In more than half of the evaluated tasks, the classification performance increased by up to 5 %.
更多查看译文
关键词
time series,unevenly spaced time series,time series analysis,classification,lomb-scargle periodogram,spectral analysis,network traffic,machine learning
AI 理解论文
溯源树
样例
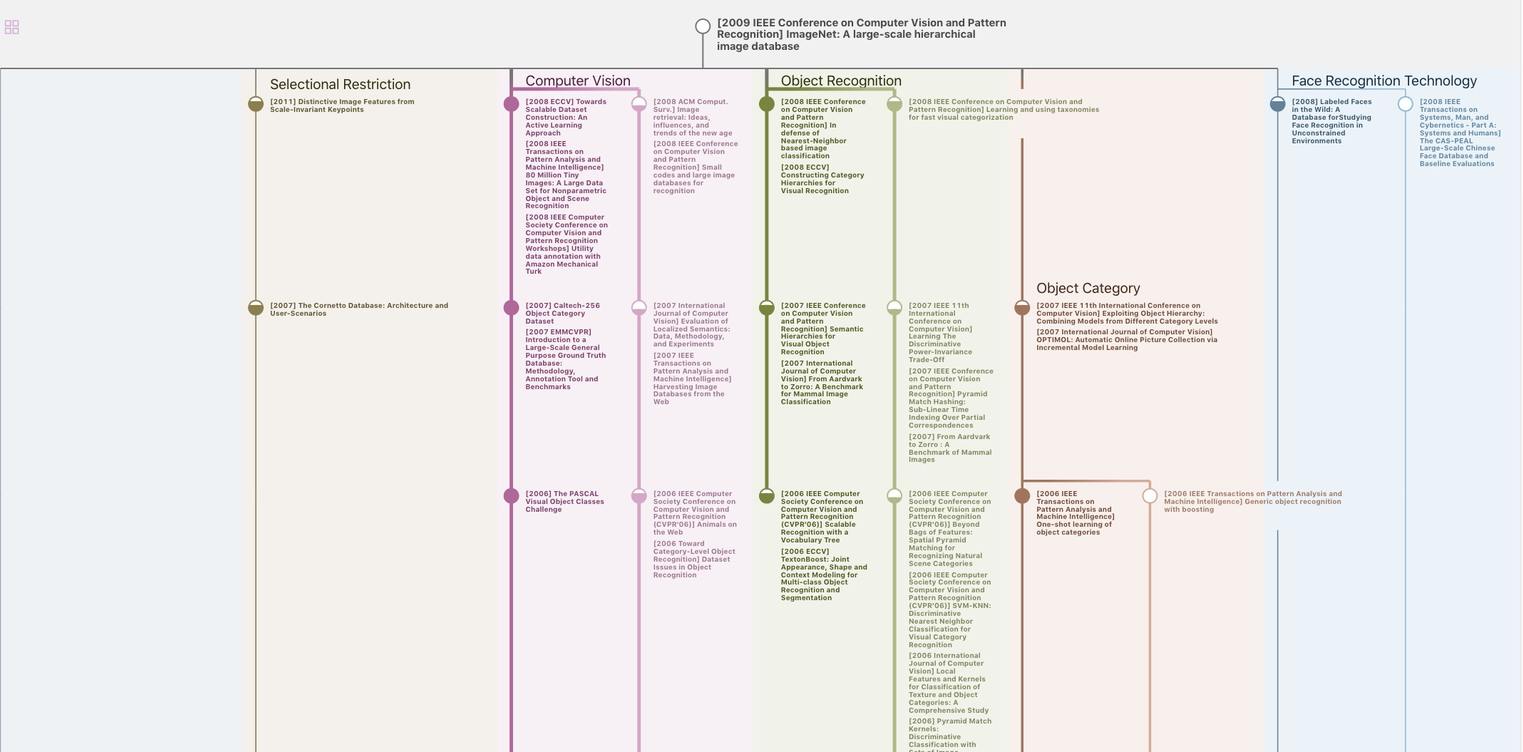
生成溯源树,研究论文发展脉络
Chat Paper
正在生成论文摘要