General-Purpose Multi-Modal OOD Detection Framework
CoRR(2023)
摘要
Out-of-distribution (OOD) detection identifies test samples that differ from the training data, which is critical to ensuring the safety and reliability of machine learning (ML) systems. While a plethora of methods have been developed to detect uni-modal OOD samples, only a few have focused on multi-modal OOD detection. Current contrastive learning-based methods primarily study multi-modal OOD detection in a scenario where both a given image and its corresponding textual description come from a new domain. However, real-world deployments of ML systems may face more anomaly scenarios caused by multiple factors like sensor faults, bad weather, and environmental changes. Hence, the goal of this work is to simultaneously detect from multiple different OOD scenarios in a fine-grained manner. To reach this goal, we propose a general-purpose weakly-supervised OOD detection framework, called WOOD, that combines a binary classifier and a contrastive learning component to reap the benefits of both. In order to better distinguish the latent representations of in-distribution (ID) and OOD samples, we adopt the Hinge loss to constrain their similarity. Furthermore, we develop a new scoring metric to integrate the prediction results from both the binary classifier and contrastive learning for identifying OOD samples. We evaluate the proposed WOOD model on multiple real-world datasets, and the experimental results demonstrate that the WOOD model outperforms the state-of-the-art methods for multi-modal OOD detection. Importantly, our approach is able to achieve high accuracy in OOD detection in three different OOD scenarios simultaneously. The source code will be made publicly available upon publication.
更多查看译文
关键词
detection,general-purpose,multi-modal
AI 理解论文
溯源树
样例
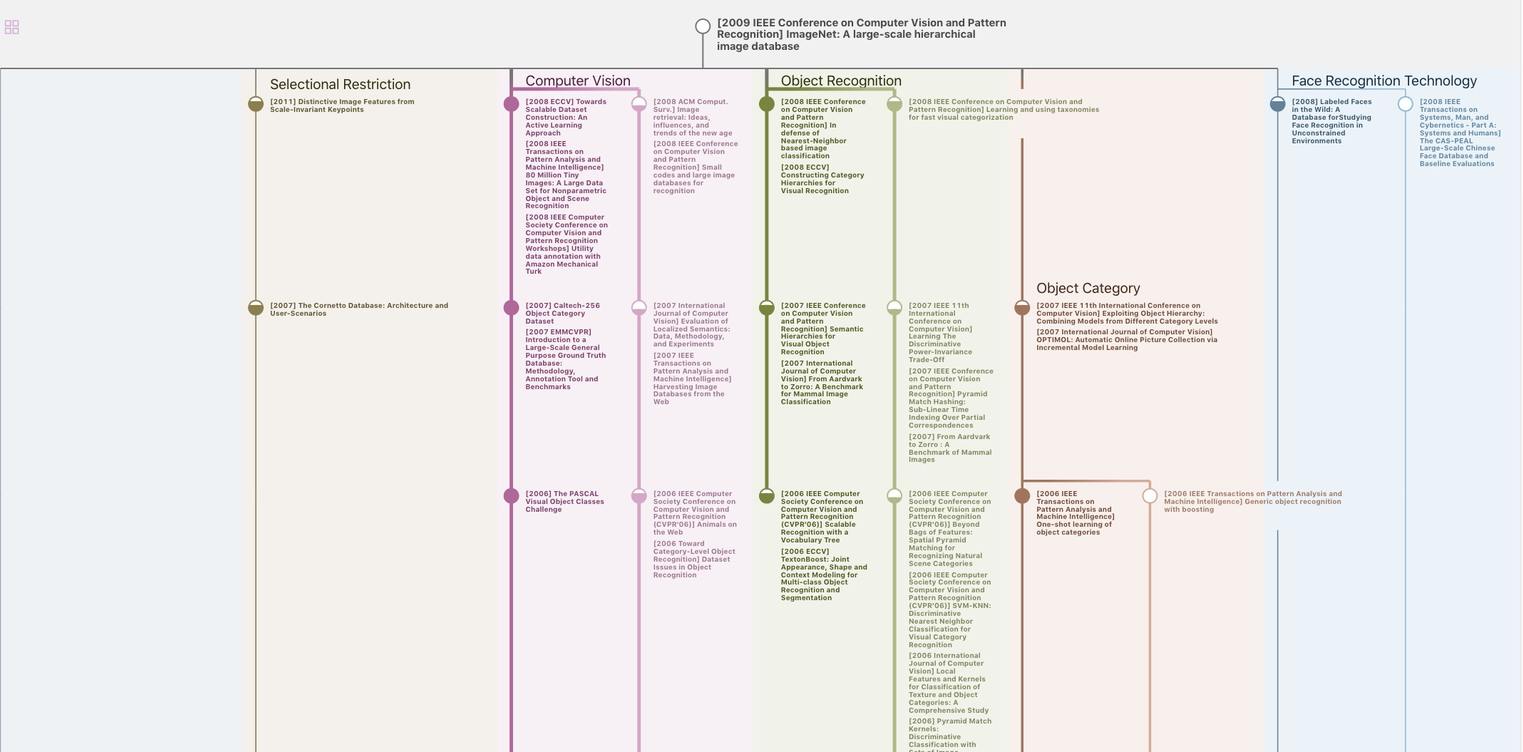
生成溯源树,研究论文发展脉络
Chat Paper
正在生成论文摘要