Automated detection and segmentation of pulmonary embolisms on computed tomography pulmonary angiography (CTPA) using deep learning but without manual outlining
Medical image analysis(2023)
摘要
We present a novel computer algorithm to automatically detect and segment pulmonary embolisms (PEs) on computed tomography pulmonary angiography (CTPA). This algorithm is based on deep learning but does not require manual outlines of the PE regions. Given a CTPA scan, both intra-and extra-pulmonary arteries were firstly segmented. The arteries were then partitioned into several parts based on size (radius). Adaptive thresholding and constrained morphological operations were used to identify suspicious PE regions within each part. The confidence of a suspicious region to be PE was scored based on its contrast in the arteries. This approach was applied to the publicly available RSNA Pulmonary Embolism CT Dataset (RSNA-PE) to identify three-dimensional (3-D) PE negative and positive image patches, which were used to train a 3-D Recurrent Residual U-Net (R2-Unet) to automatically segment PE. The feasibility of this computer algorithm was validated on an independent test set consisting of 91 CTPA scans acquired from a different medical institute, where the PE regions were manually located and outlined by a thoracic radiologist (>18 years' experience). An R2-Unet model was also trained and validated on the manual outlines using a 5-fold cross-validation method. The CNN model trained on the high-confident PE regions showed a Dice coefficient of 0.676 & PLUSMN;0.168 and a false positive rate of 1.86 per CT scan, while the CNN model trained on the manual outlines demonstrated a Dice coefficient of 0.647 & PLUSMN;0.192 and a false positive rate of 4.20 per CT scan. The former model performed significantly better than the latter model (p<0.01). The promising performance of the developed PE detection and segmentation algorithm suggests the feasibility of training a deep learning network without dedicating significant efforts to manual annotations of the PE regions on CTPA scans.
更多查看译文
关键词
Pulmonary embolism,Pulmonary angiography,Manual outlining,Deep learning
AI 理解论文
溯源树
样例
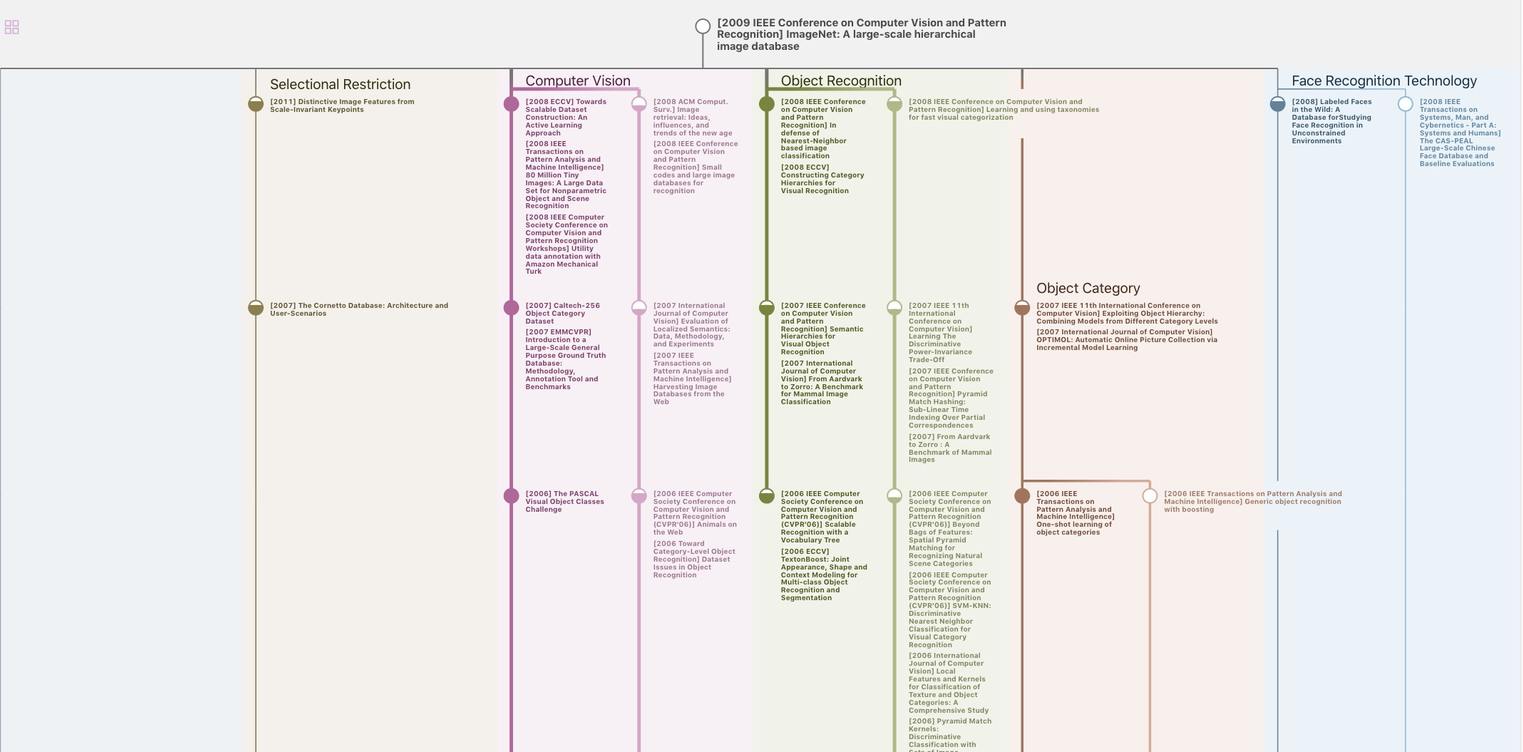
生成溯源树,研究论文发展脉络
Chat Paper
正在生成论文摘要