HiHGNN: Accelerating HGNNs through Parallelism and Data Reusability Exploitation
IEEE Transactions on Parallel and Distributed Systems(2023)
摘要
Heterogeneous graph neural networks (HGNNs) have emerged as powerful
algorithms for processing heterogeneous graphs (HetGs), widely used in many
critical fields. To capture both structural and semantic information in HetGs,
HGNNs first aggregate the neighboring feature vectors for each vertex in each
semantic graph and then fuse the aggregated results across all semantic graphs
for each vertex. Unfortunately, existing graph neural network accelerators are
ill-suited to accelerate HGNNs. This is because they fail to efficiently tackle
the specific execution patterns and exploit the high-degree parallelism as well
as data reusability inside and across the processing of semantic graphs in
HGNNs.
In this work, we first quantitatively characterize a set of representative
HGNN models on GPU to disclose the execution bound of each stage,
inter-semantic-graph parallelism, and inter-semantic-graph data reusability in
HGNNs. Guided by our findings, we propose a high-performance HGNN accelerator,
HiHGNN, to alleviate the execution bound and exploit the newfound parallelism
and data reusability in HGNNs. Specifically, we first propose a bound-aware
stage-fusion methodology that tailors to HGNN acceleration, to fuse and
pipeline the execution stages being aware of their execution bounds. Second, we
design an independency-aware parallel execution design to exploit the
inter-semantic-graph parallelism. Finally, we present a similarity-aware
execution scheduling to exploit the inter-semantic-graph data reusability.
Compared to the state-of-the-art software framework running on NVIDIA GPU T4
and GPU A100, HiHGNN respectively achieves an average 41.5× and
8.6× speedup as well as 106× and 73× energy efficiency
with quarter the memory bandwidth of GPU A100.
更多查看译文
关键词
Heterogeneous graph neural network,Graph neural network,HGNN accelerator,GNN accelerator,HGNN,GNN
AI 理解论文
溯源树
样例
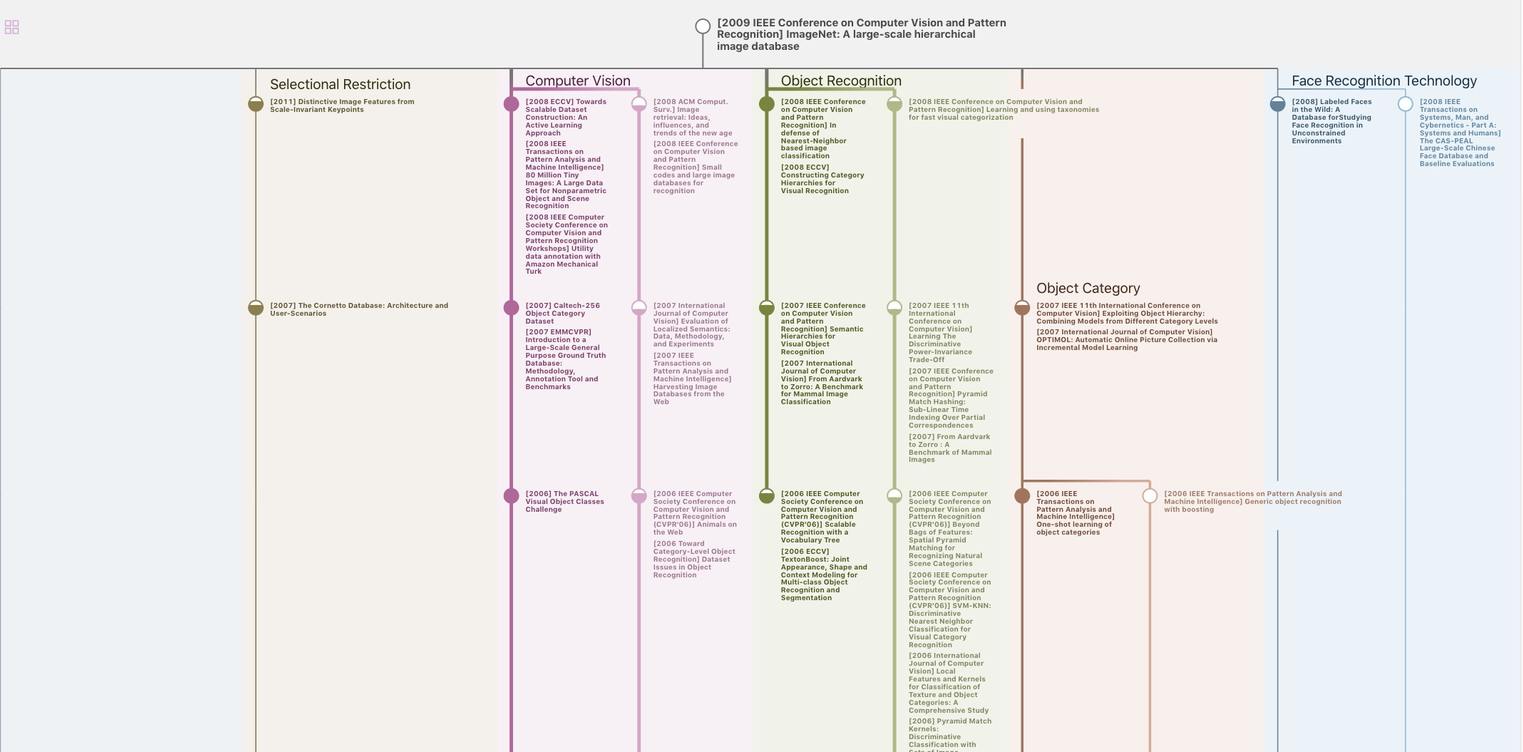
生成溯源树,研究论文发展脉络
Chat Paper
正在生成论文摘要