A Model for Every User and Budget: Label-Free and Personalized Mixed-Precision Quantization
CoRR(2023)
摘要
Recent advancement in Automatic Speech Recognition (ASR) has produced large
AI models, which become impractical for deployment in mobile devices. Model
quantization is effective to produce compressed general-purpose models, however
such models may only be deployed to a restricted sub-domain of interest. We
show that ASR models can be personalized during quantization while relying on
just a small set of unlabelled samples from the target domain. To this end, we
propose myQASR, a mixed-precision quantization method that generates tailored
quantization schemes for diverse users under any memory requirement with no
fine-tuning. myQASR automatically evaluates the quantization sensitivity of
network layers by analysing the full-precision activation values. We are then
able to generate a personalised mixed-precision quantization scheme for any
pre-determined memory budget. Results for large-scale ASR models show how
myQASR improves performance for specific genders, languages, and speakers.
更多查看译文
关键词
budget,personalized
AI 理解论文
溯源树
样例
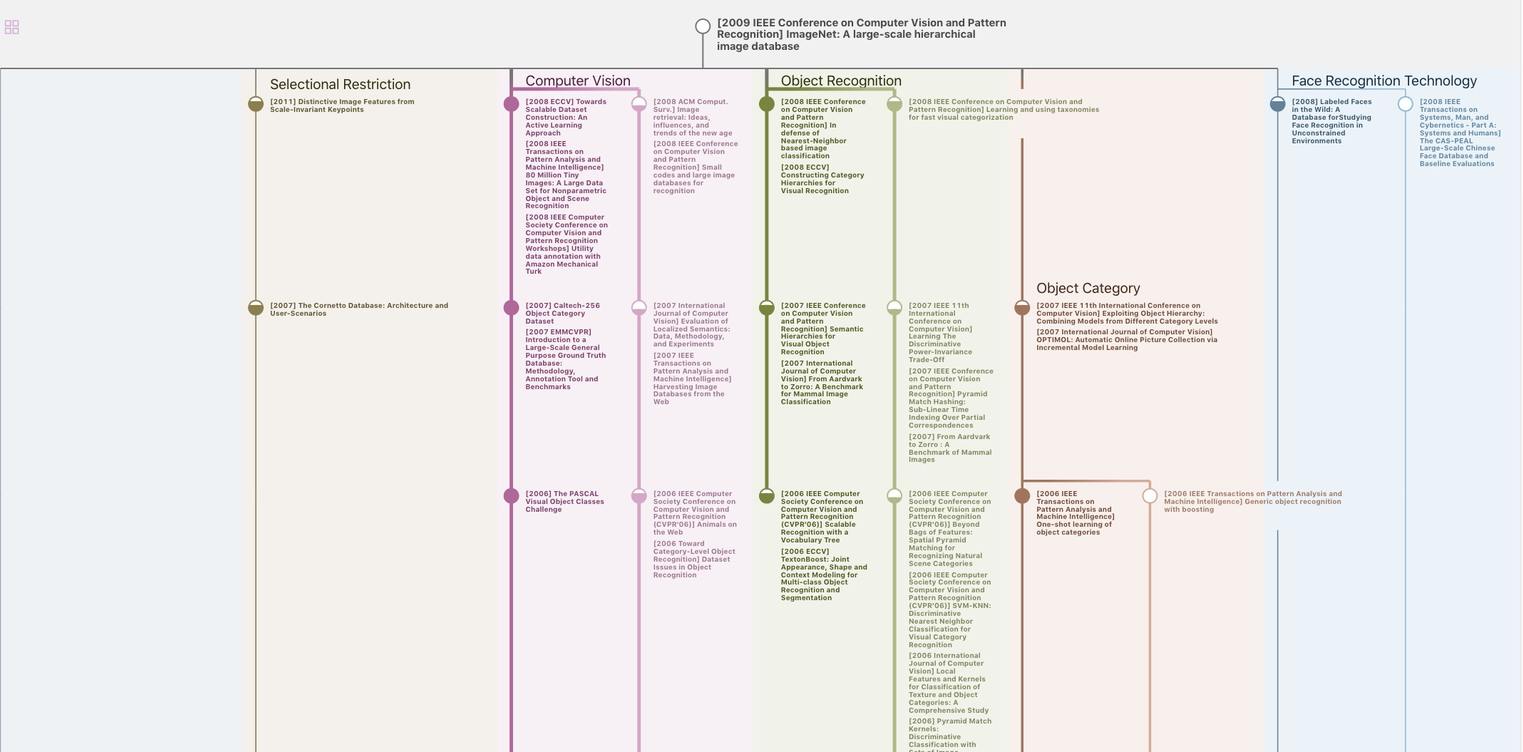
生成溯源树,研究论文发展脉络
Chat Paper
正在生成论文摘要