Tuning-free one-bit covariance estimation using data-driven dithering
CoRR(2023)
摘要
We consider covariance estimation of any subgaussian distribution from finitely many i.i.d. samples that are quantized to one bit of information per entry. Recent work has shown that a reliable estimator can be constructed if uniformly distributed dithers on $[-\lambda,\lambda]$ are used in the one-bit quantizer. This estimator enjoys near-minimax optimal, non-asymptotic error estimates in the operator and Frobenius norms if $\lambda$ is chosen proportional to the largest variance of the distribution. However, this quantity is not known a-priori, and in practice $\lambda$ needs to be carefully tuned to achieve good performance. In this work we resolve this problem by introducing a tuning-free variant of this estimator, which replaces $\lambda$ by a data-driven quantity. We prove that this estimator satisfies the same non-asymptotic error estimates - up to small (logarithmic) losses and a slightly worse probability estimate. Our proof relies on a new version of the Burkholder-Rosenthal inequalities for matrix martingales, which is expected to be of independent interest.
更多查看译文
关键词
Covariance estimation,Dithering,One-bit quantization
AI 理解论文
溯源树
样例
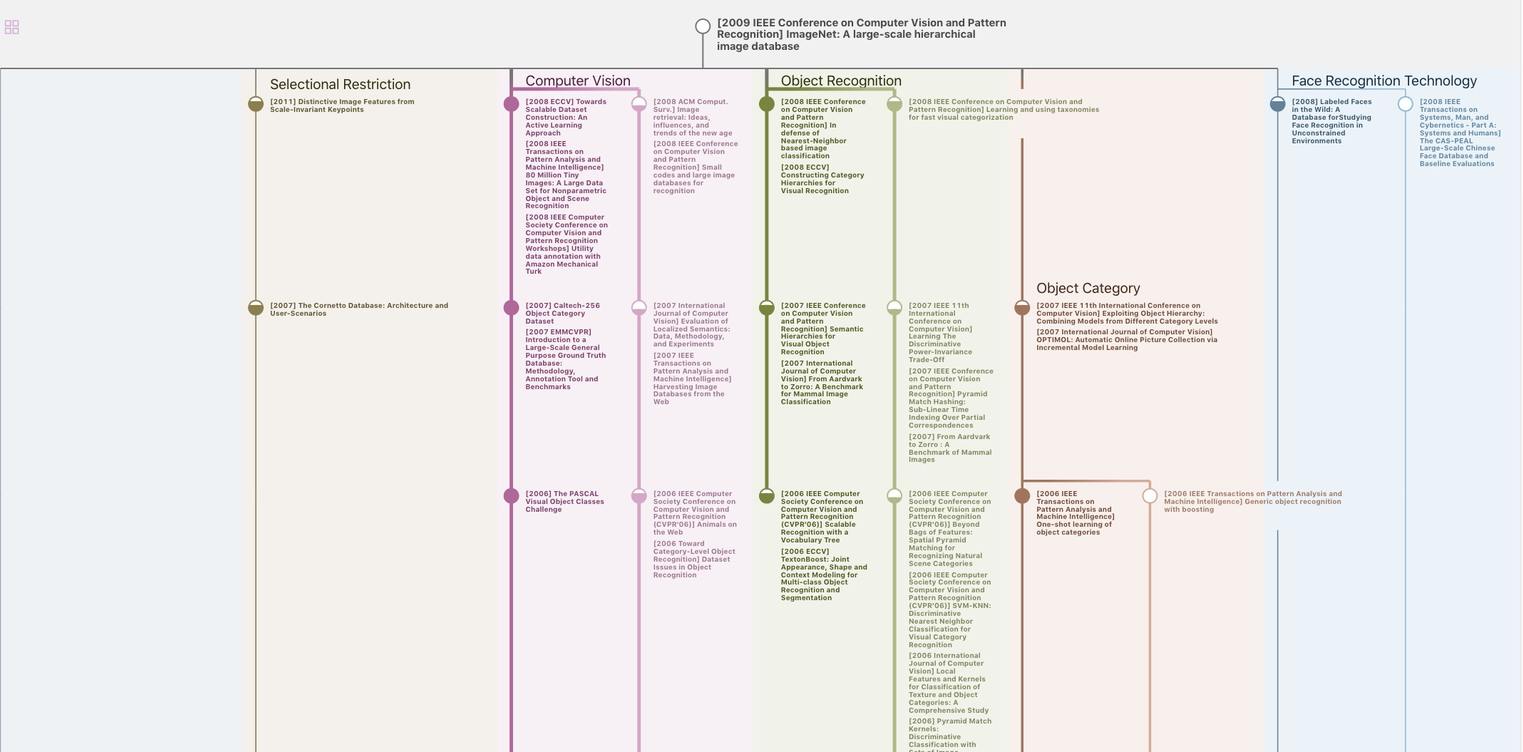
生成溯源树,研究论文发展脉络
Chat Paper
正在生成论文摘要