Multi-Fidelity Data Assimilation For Physics Inspired Machine Learning In Uncertainty Quantification Of Fluid Turbulence Simulations
arXiv (Cornell University)(2023)
摘要
Reliable prediction of turbulent flows is an important necessity across different fields of science and engineering. In Computational Fluid Dynamics (CFD) simulations, the most common type of models are eddy viscosity models that are computationally inexpensive but introduce a high degree of epistemic error. The Eigenspace Perturbation Method (EPM) attempts to quantify this predictive uncertainty via physics based perturbation in the spectral representation of the predictions. While the EPM outlines how to perturb, it does not address how much or even where to perturb. We address this need by introducing machine learning models to predict the details of the perturbation, thus creating a physics inspired machine learning (ML) based perturbation framework. In our choice of ML models, we focus on incorporating physics based inductive biases while retaining computational economy. Specifically we use a Convolutional Neural Network (CNN) to learn the correction between turbulence model predictions and true results. This physics inspired machine learning based perturbation approach is able to modulate the intensity and location of perturbations and leads to improved estimates of turbulence model errors.
更多查看译文
关键词
data assimilation,physics inspired machine learning
AI 理解论文
溯源树
样例
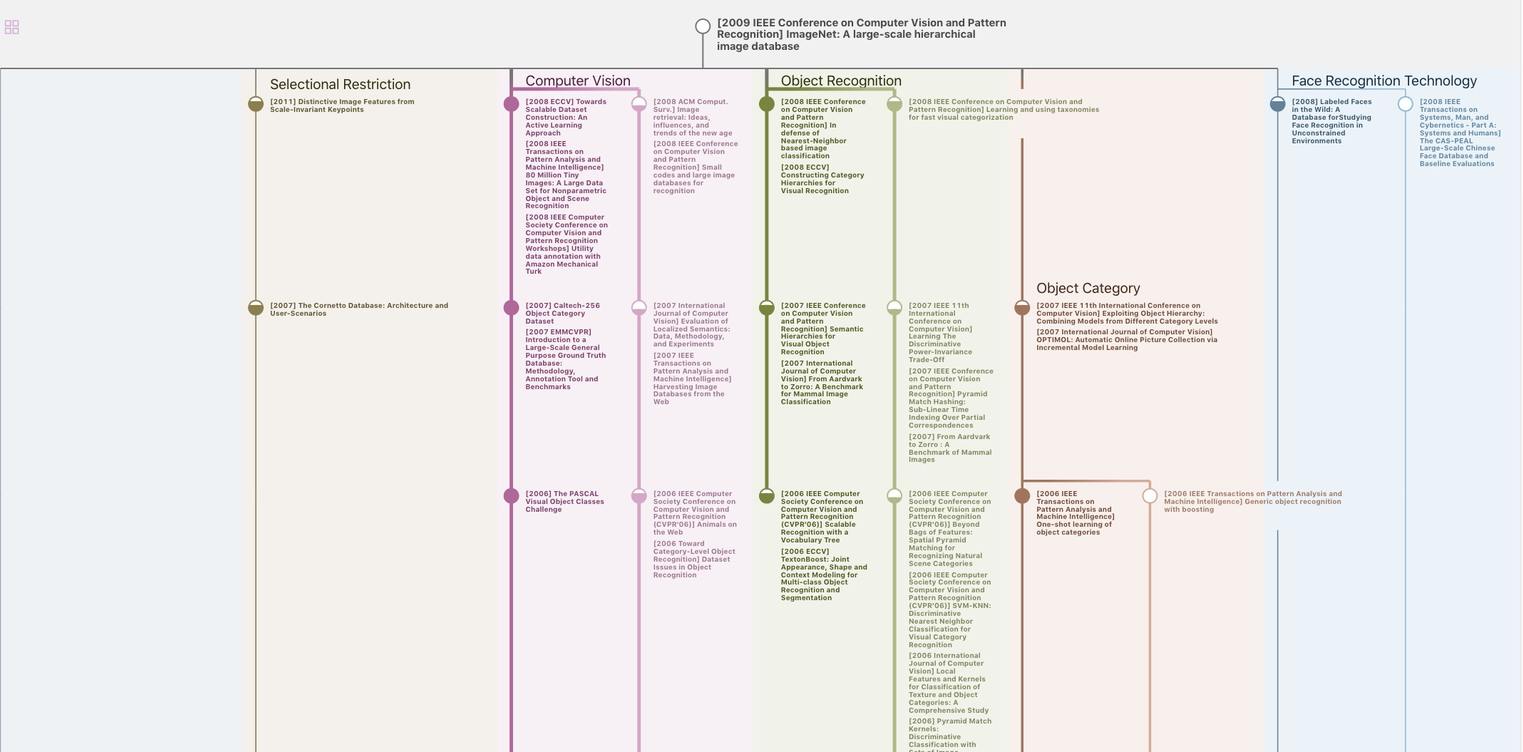
生成溯源树,研究论文发展脉络
Chat Paper
正在生成论文摘要