Open Problem: The Sample Complexity of Multi-Distribution Learning for VC Classes.
CoRR(2023)
摘要
Multi-distribution learning is a natural generalization of PAC learning to settings with multiple data distributions. There remains a significant gap between the known upper and lower bounds for PAC-learnable classes. In particular, though we understand the sample complexity of learning a VC dimension d class on $k$ distributions to be $O(\epsilon^{-2} \ln(k)(d + k) + \min\{\epsilon^{-1} dk, \epsilon^{-4} \ln(k) d\})$, the best lower bound is $\Omega(\epsilon^{-2}(d + k \ln(k)))$. We discuss recent progress on this problem and some hurdles that are fundamental to the use of game dynamics in statistical learning.
更多查看译文
关键词
sample complexity,learning,multi-distribution
AI 理解论文
溯源树
样例
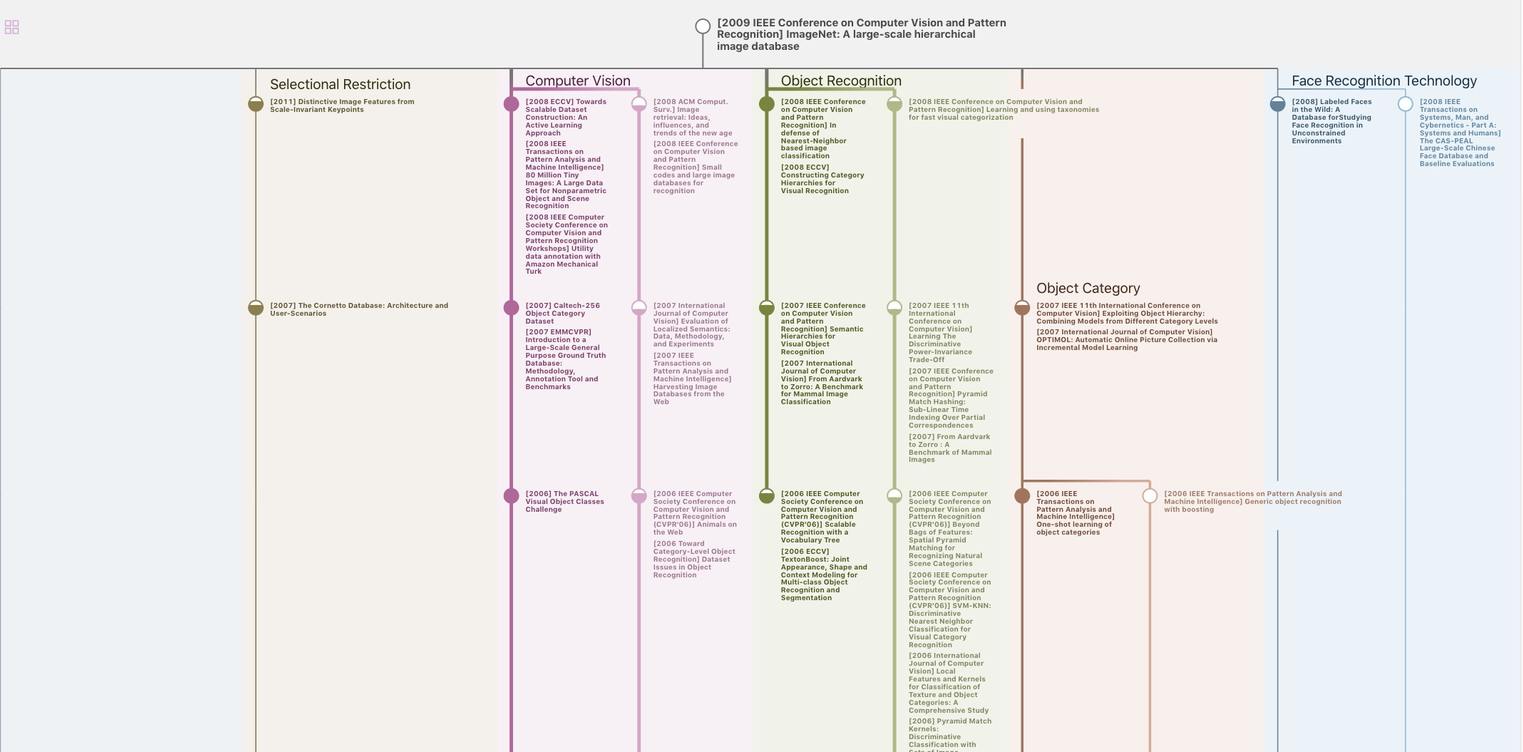
生成溯源树,研究论文发展脉络
Chat Paper
正在生成论文摘要