Selective Perception: Optimizing State Descriptions with Reinforcement Learning for Language Model Actors
CoRR(2023)
摘要
Large language models (LLMs) are being applied as actors for sequential decision making tasks in domains such as robotics and games, utilizing their general world knowledge and planning abilities. However, previous work does little to explore what environment state information is provided to LLM actors via language. Exhaustively describing high-dimensional states can impair performance and raise inference costs for LLM actors. Previous LLM actors avoid the issue by relying on hand-engineered, task-specific protocols to determine which features to communicate about a state and which to leave out. In this work, we propose Brief Language INputs for DEcision-making Responses (BLINDER), a method for automatically selecting concise state descriptions by learning a value function for task-conditioned state descriptions. We evaluate BLINDER on the challenging video game NetHack and a robotic manipulation task. Our method improves task success rate, reduces input size and compute costs, and generalizes between LLM actors.
更多查看译文
关键词
state descriptions,selective perception,reinforcement
AI 理解论文
溯源树
样例
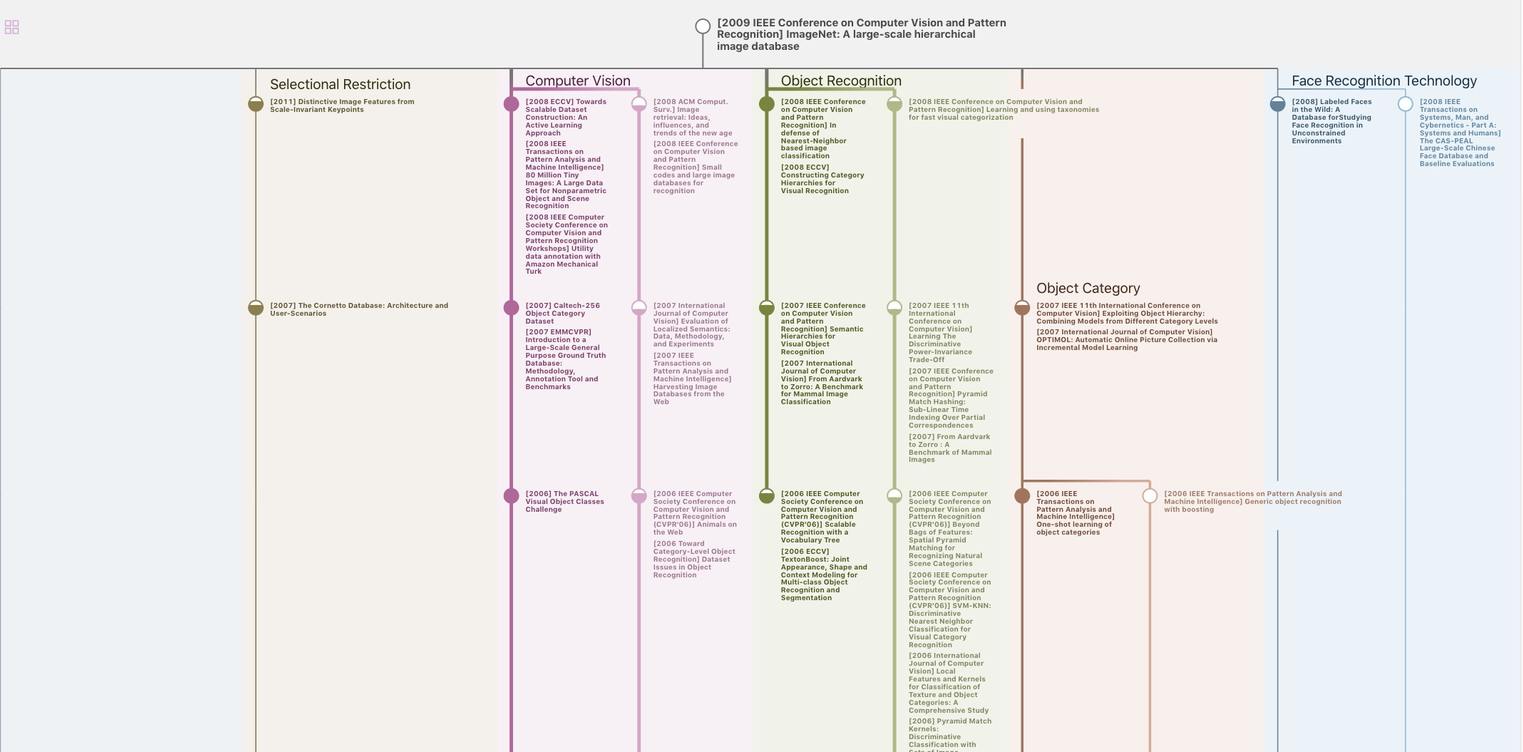
生成溯源树,研究论文发展脉络
Chat Paper
正在生成论文摘要