Prompting Large Language Models with Speech Recognition Abilities
ICASSP 2024 - 2024 IEEE International Conference on Acoustics, Speech and Signal Processing (ICASSP)(2023)
摘要
Large language models have proven themselves highly flexible, able to solve a wide range of generative tasks, such as abstractive summarization and open-ended question answering. In this paper we extend the capabilities of LLMs by directly attaching a small audio encoder allowing it to perform speech recognition. By directly prepending a sequence of audial embeddings to the text token embeddings, the LLM can be converted to an automatic speech recognition (ASR) system, and be used in the exact same manner as its textual counterpart. Experiments on Multilingual LibriSpeech (MLS) show that incorporating a conformer encoder into the open sourced LLaMA-7B allows it to outperform monolingual baselines by 18% and perform multilingual speech recognition despite LLaMA being trained overwhelmingly on English text. Furthermore, we perform ablation studies to investigate whether the LLM can be completely frozen during training to maintain its original capabilities, scaling up the audio encoder, and increasing the audio encoder striding to generate fewer embeddings. The results from these studies show that multilingual ASR is possible even when the LLM is frozen or when strides of almost 1 second are used in the audio encoder opening up the possibility for LLMs to operate on long-form audio.
更多查看译文
关键词
large language model,speech recognition,LLaMA
AI 理解论文
溯源树
样例
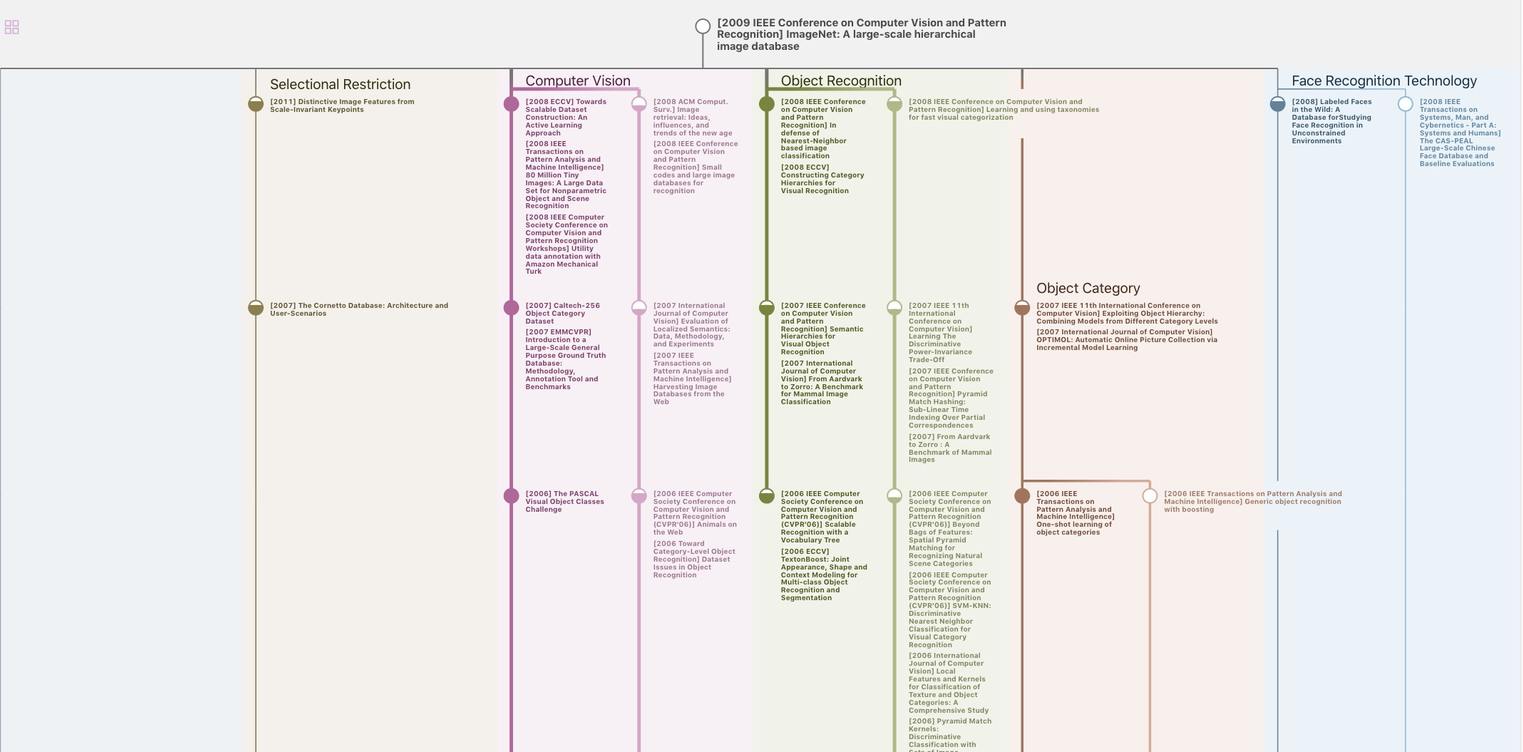
生成溯源树,研究论文发展脉络
Chat Paper
正在生成论文摘要