NeuralSlice: Neural 3D Triangle Mesh Reconstruction via Slicing 4D Tetrahedral Meshes
ICML 2023(2023)
摘要
Learning-based high-fidelity reconstruction of 3D shapes with varying topology is a fundamental problem in computer vision and computer graphics. Recent advances in learning 3D shapes using explicit and implicit representations have achieved impressive results in 3D modeling. However, the template-based explicit representation is limited by fixed topology, and the implicit representation, although flexible with arbitrary topology, requires a large number of sampled points to regress the surface, which is computationally expensive. In this work, we propose a novel 3D shape representation named NeuralSlice, which represents a 3D shape as the intersection of a 4D tetrahedral mesh and a 4D hyperplane. A novel network is designed to incorporate the proposed representation flexibly, which learns a deformable 4D template and a parameter for slicing 4D hyperplane to reconstruct the 3D object. To learn the local deformation of the 4D template, we further propose a spatial-aware network to locate the 4D points within the 3D feature volume of input shape via positional encoding, which leverages the local geometrical feature to guide the 4D deformation. By addressing the 3D problem in a higher 4D space, our method supports flexible topology changes while being highly efficient. Our method is guaranteed to produce manifold meshes. NeuralSlice outperforms the state-of-the-art explicit-based approaches in terms of reconstruction quality. Compared with implicit approaches, by avoiding point sampling, our method is 10 times faster than the implicit approaches, and better preserves thin structures. NeuralSlice has the capability of representing various shapes and topologies using a single 4D tetrahedral mesh. The corresponding code can be found on GitHub at https://github.com/IGLICT/NEURALSLICE
更多查看译文
AI 理解论文
溯源树
样例
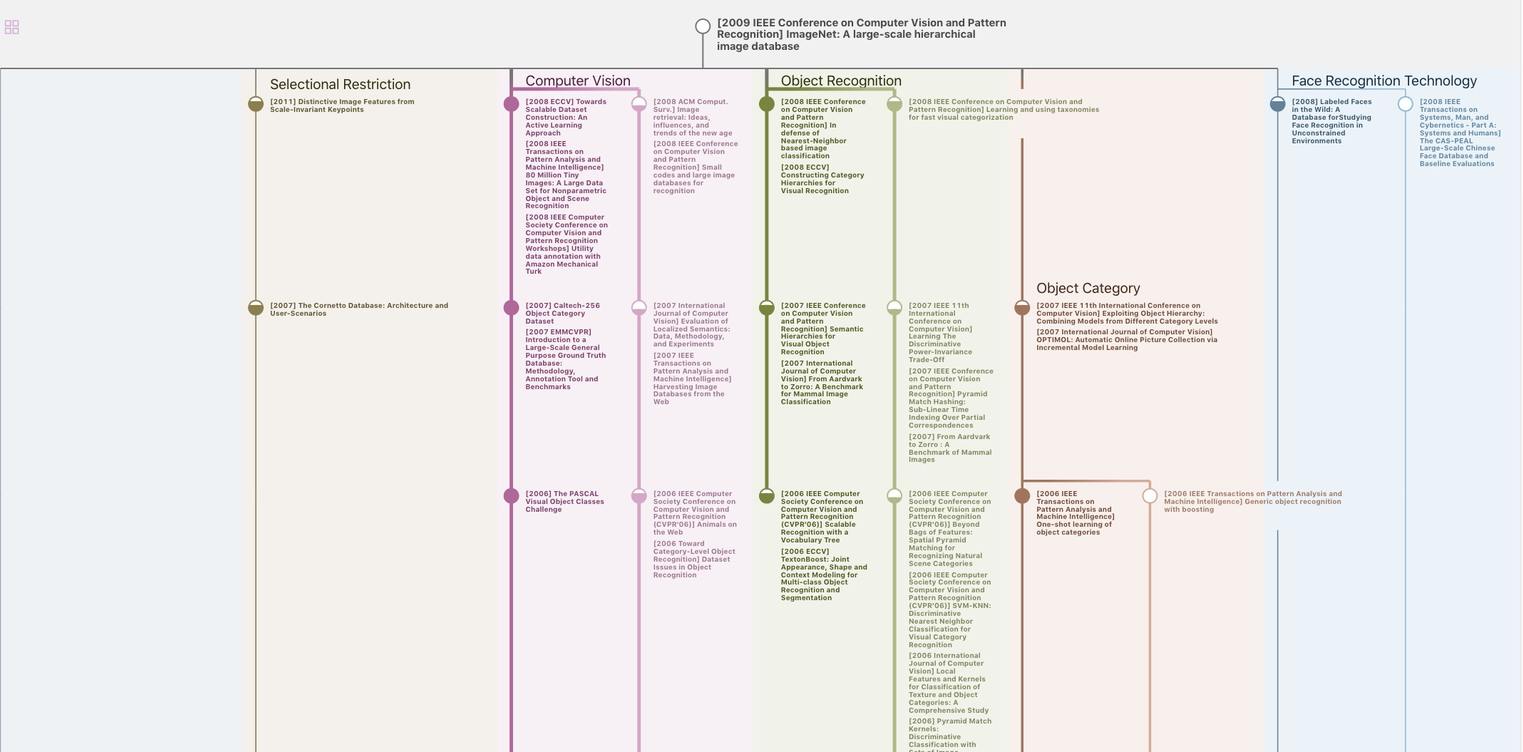
生成溯源树,研究论文发展脉络
Chat Paper
正在生成论文摘要