Policy Gradient in Robust MDPs with Global Convergence Guarantee
ICML 2023(2023)
摘要
Robust Markov decision processes (RMDPs) provide a promising framework for computing reliable policies in the face of model errors. Many successful reinforcement learning algorithms build on variations of policy-gradient methods, but adapting these methods to RMDPs has been challenging. As a result, the applicability of RMDPs to large, practical domains remains limited. This paper proposes a new Double-Loop Robust Policy Gradient (DRPG), the first generic policy gradient method for RMDPs. In contrast with prior robust policy gradient algorithms, DRPG monotonically reduces approximation errors to guarantee convergence to a globally optimal policy in tabular RMDPs. We introduce a novel parametric transition kernel and solve the inner loop robust policy via a gradient-based method. Finally, our numerical results demonstrate the utility of our new algorithm and confirm its global convergence properties.
更多查看译文
关键词
robust mdps,gradient,policy
AI 理解论文
溯源树
样例
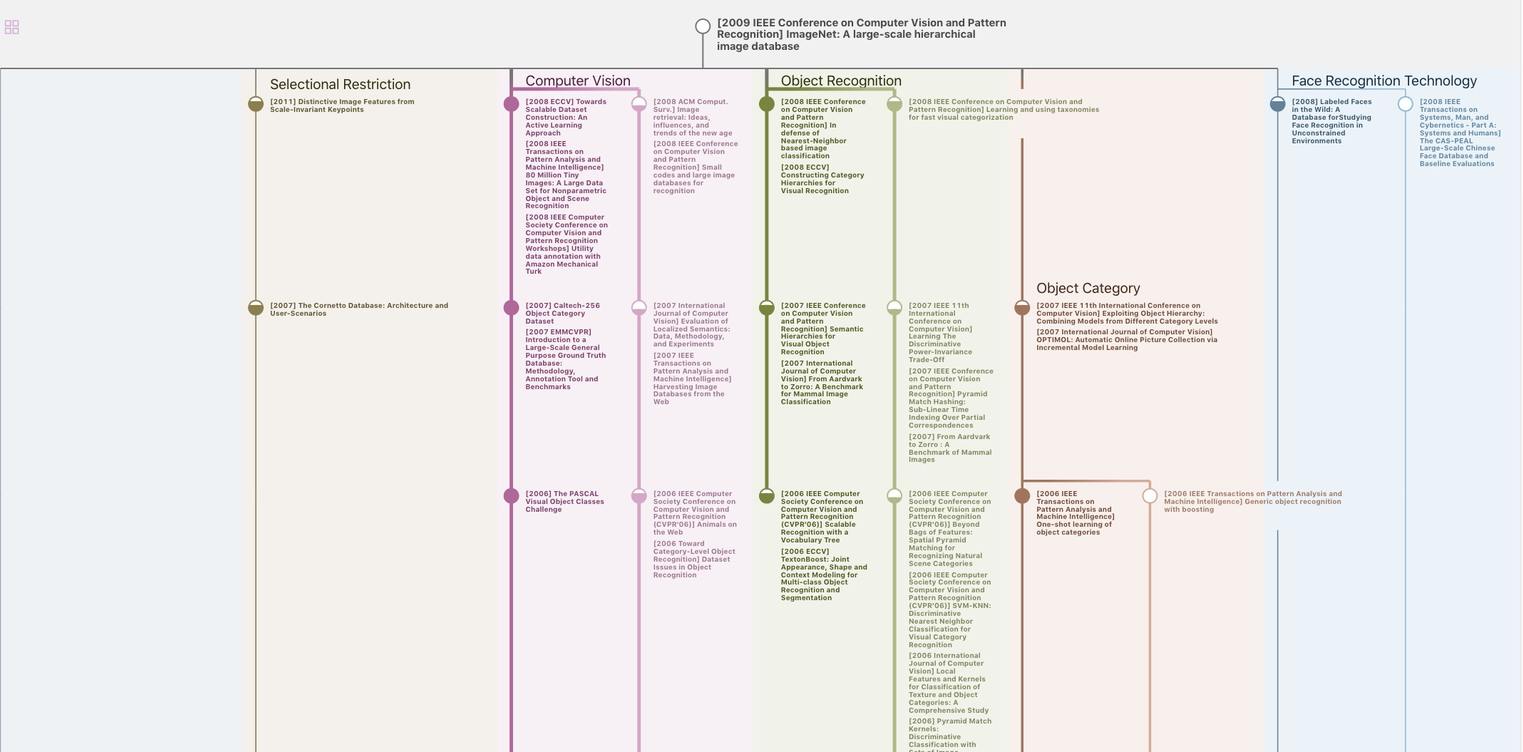
生成溯源树,研究论文发展脉络
Chat Paper
正在生成论文摘要