Temporally Consistent Transformers for Video Generation
ICML 2023(2023)
摘要
To generate accurate videos, algorithms have to understand the spatial and temporal dependencies in the world. Current algorithms enable accurate predictions over short horizons but tend to suffer from temporal inconsistencies. When generated content goes out of view and is later revisited, the model invents different content instead. Despite this severe limitation, no established benchmarks exist for video generation with long temporal dependencies. In this paper, we curate 3 challenging video datasets with long-range dependencies by rendering walks through 3D scenes of procedural mazes, Minecraft worlds, and indoor scans. We perform a comprehensive evaluation of current models and observe their limitations in temporal consistency. Moreover, we introduce the Temporally Consistent Transformer (TECO), a generative model that substantially improves long-term consistency while also reducing sampling time. By compressing its input sequence into fewer embeddings, applying a temporal transformer, and expanding back using a spatial MaskGit, TECO outperforms existing models across many metrics. Videos are available on the website: https://wilson1yan.github.io/teco
更多查看译文
关键词
video generation,consistent transformers
AI 理解论文
溯源树
样例
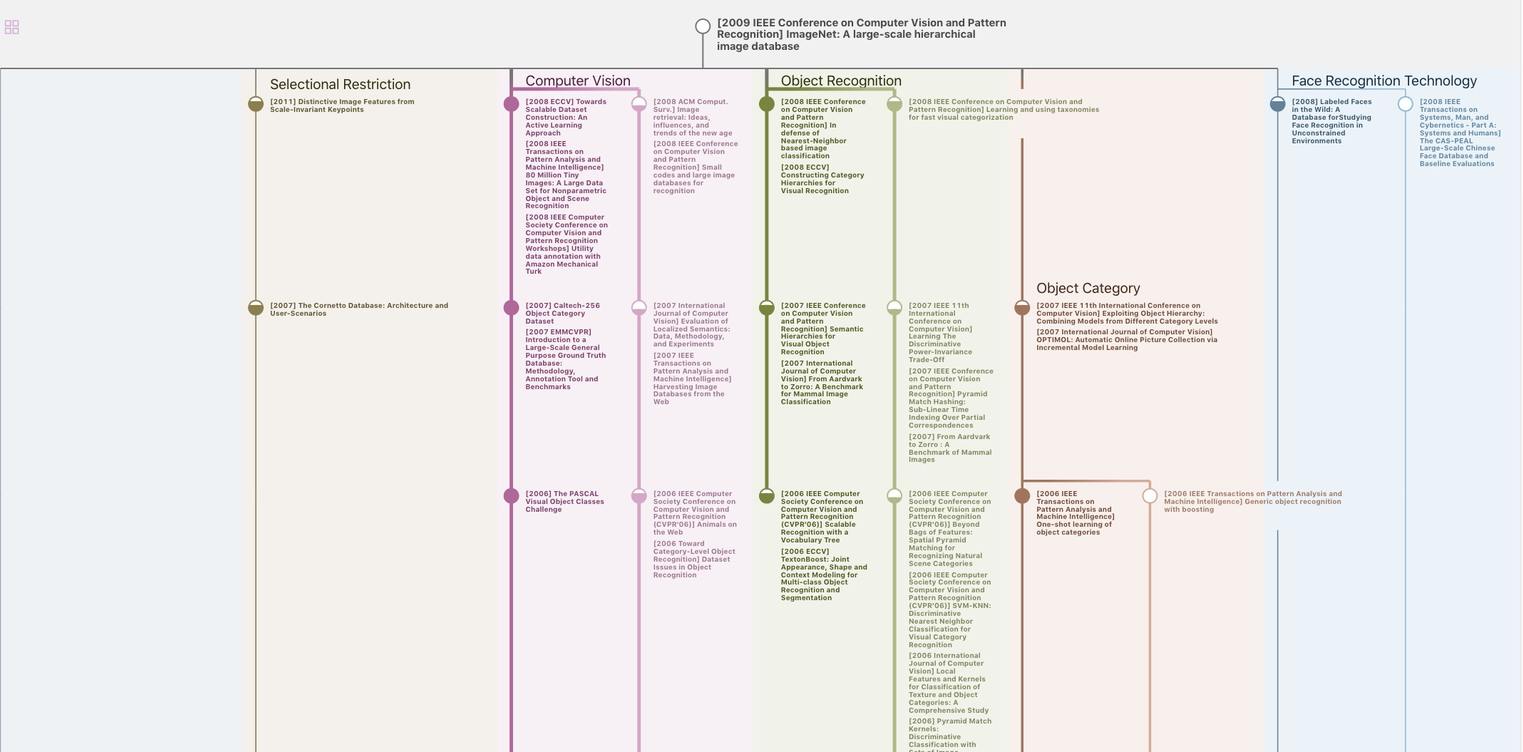
生成溯源树,研究论文发展脉络
Chat Paper
正在生成论文摘要