Byzantine-Robust Learning on Heterogeneous Data via Gradient Splitting
ICML 2023(2023)
摘要
Federated learning has exhibited vulnerabilities to Byzantine attacks, where the Byzantine attackers can send arbitrary gradients to a central server to destroy the convergence and performance of the global model. A wealth of robust AGgregation Rules (AGRs) have been proposed to defend against Byzantine attacks. However, Byzantine clients can still circumvent robust AGRs when data is non-Identically and Independently Distributed (non-IID). In this paper, we first reveal the root causes of performance degradation of current robust AGRs in non-IID settings: the curse of dimensionality and gradient heterogeneity. In order to address this issue, we propose GAS, a GrAdient Splitting approach that can successfully adapt existing robust AGRs to non-IID settings. We also provide a detailed convergence analysis when the existing robust AGRs are combined with GAS. Experiments on various real-world datasets verify the efficacy of our proposed GAS. The implementation code is provided in https://github.com/YuchenLiu-a/byzantine-gas.
更多查看译文
关键词
gradient splitting,heterogeneous data,learning,byzantine-robust
AI 理解论文
溯源树
样例
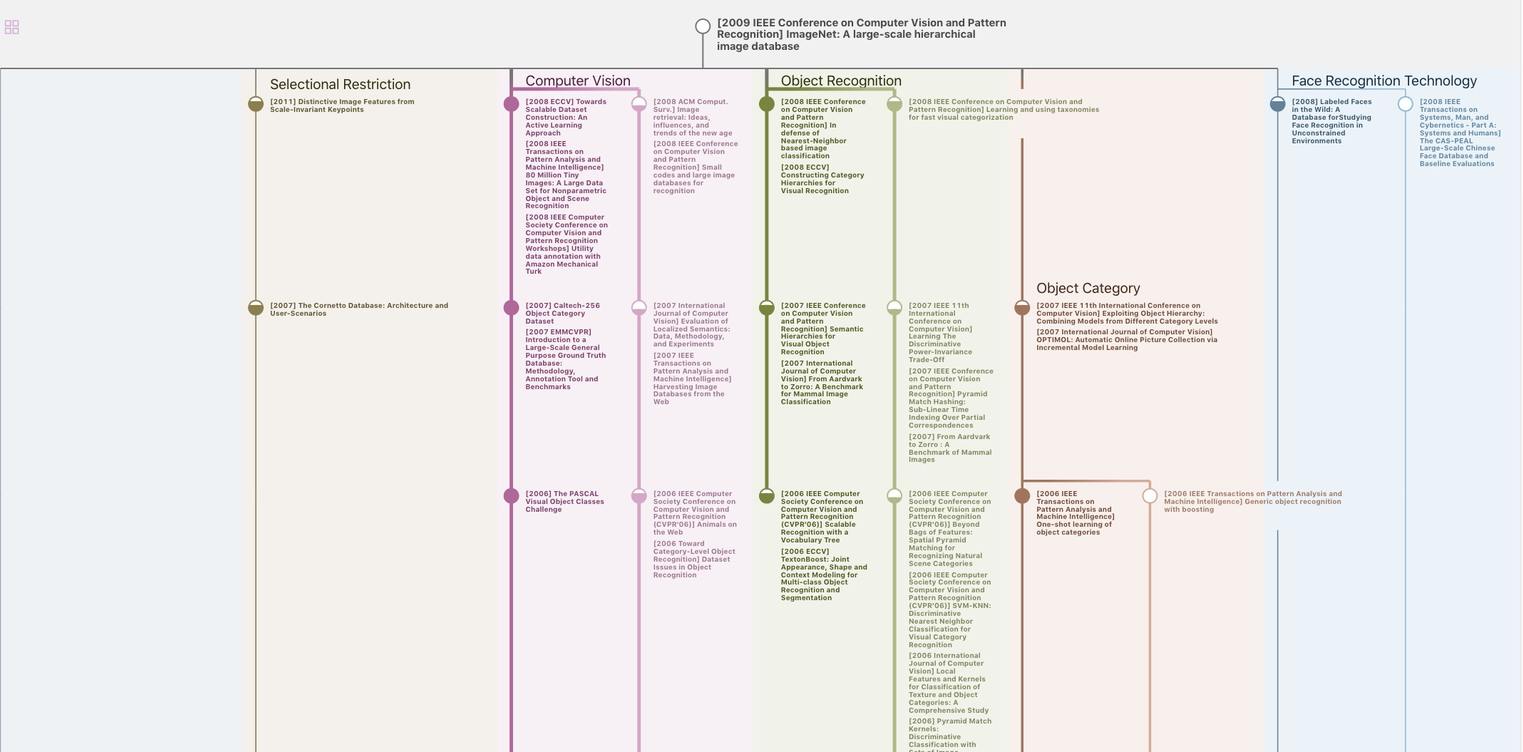
生成溯源树,研究论文发展脉络
Chat Paper
正在生成论文摘要