BEATs: Audio Pre-Training with Acoustic Tokenizers
ICML 2023(2023)
摘要
We introduce a self-supervised learning (SSL) framework BEATs for general audio representation pre-training, where we optimize an acoustic tokenizer and an audio SSL model by iterations. Unlike the previous audio SSL models that employ reconstruction loss for pre-training, our audio SSL model is trained with the discrete label prediction task, where the labels are generated by a semantic-rich acoustic tokenizer. We propose an iterative pipeline to jointly optimize the tokenizer and the pre-trained model, aiming to abstract high-level semantics and discard the redundant details for audio. The experimental results demonstrate our acoustic tokenizers can generate discrete labels with rich audio semantics and our audio SSL models achieve state-of-the-art (SOTA) results across various audio classification benchmarks, even outperforming previous models that use more training data and model parameters significantly. Specifically, we set a new SOTA mAP 50.6% on AudioSet-2M without using any external data, and 98.1% accuracy on ESC-50. The code and pre-trained models are available at https://aka.ms/beats.
更多查看译文
关键词
acoustic,pre-training
AI 理解论文
溯源树
样例
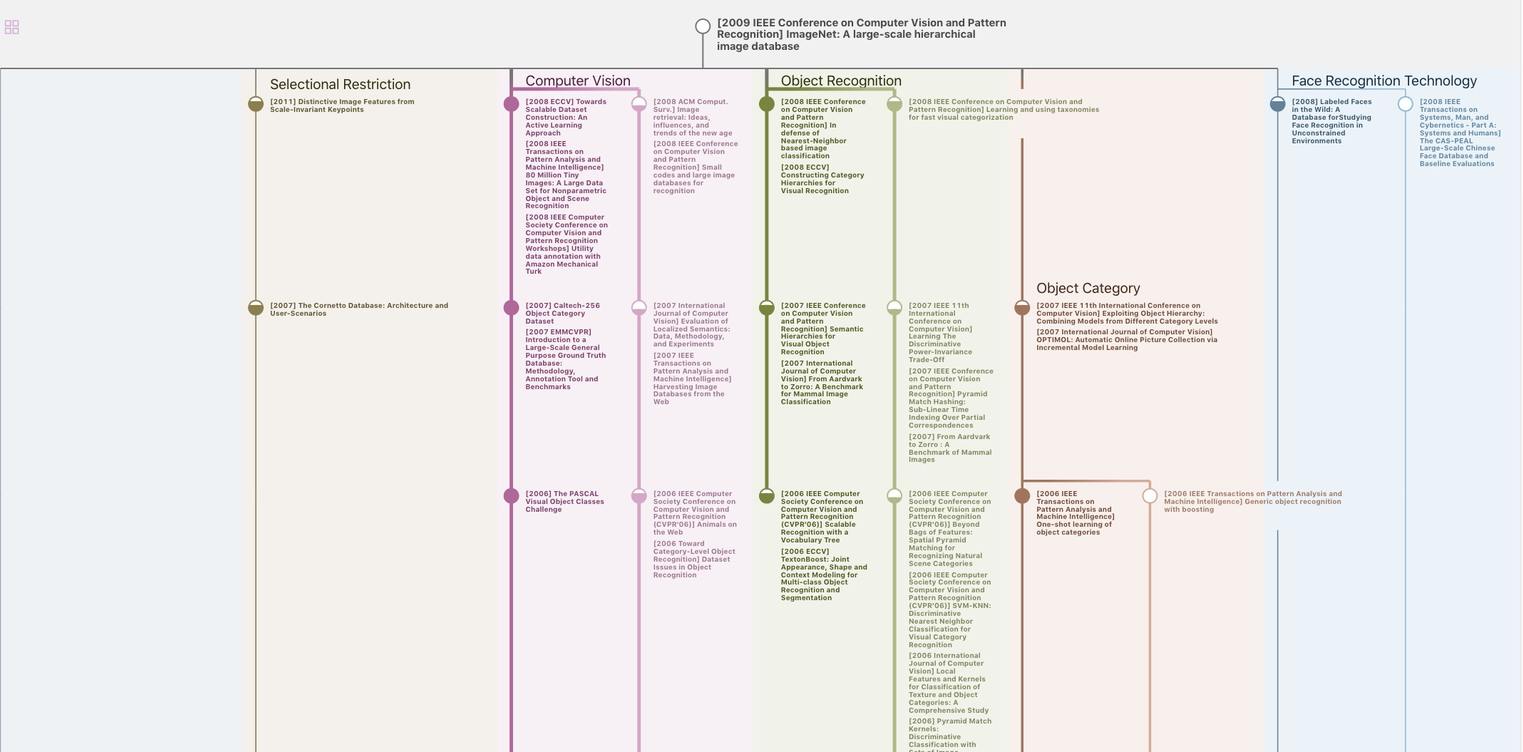
生成溯源树,研究论文发展脉络
Chat Paper
正在生成论文摘要