Strategic Classification with Unknown User Manipulations
ICML 2023(2023)
摘要
In many human-centric applications for Machine Learning instances will adapt to a classifier after its deployment. The field of strategic classification deals with this issue by aiming for a classifier that balances the trade-off between correctness and robustness to manipulation. This task is made harder if the underlying manipulation structure (i.e. the set of manipulations available at every instance) is unknown to the learner. We propose a novel batch-learning setting in which we use unlabeled data from previous rounds to estimate the manipulation structure. We show that in this batch-learning setting it is possible to learn a close-to-optimal classifier in terms of the strategic loss even without knowing the feasible manipulations beforehand. In line with recent advances in the strategic classification literature, we do not assume a best-response from agents but only require that observed manipulations are feasible.
更多查看译文
AI 理解论文
溯源树
样例
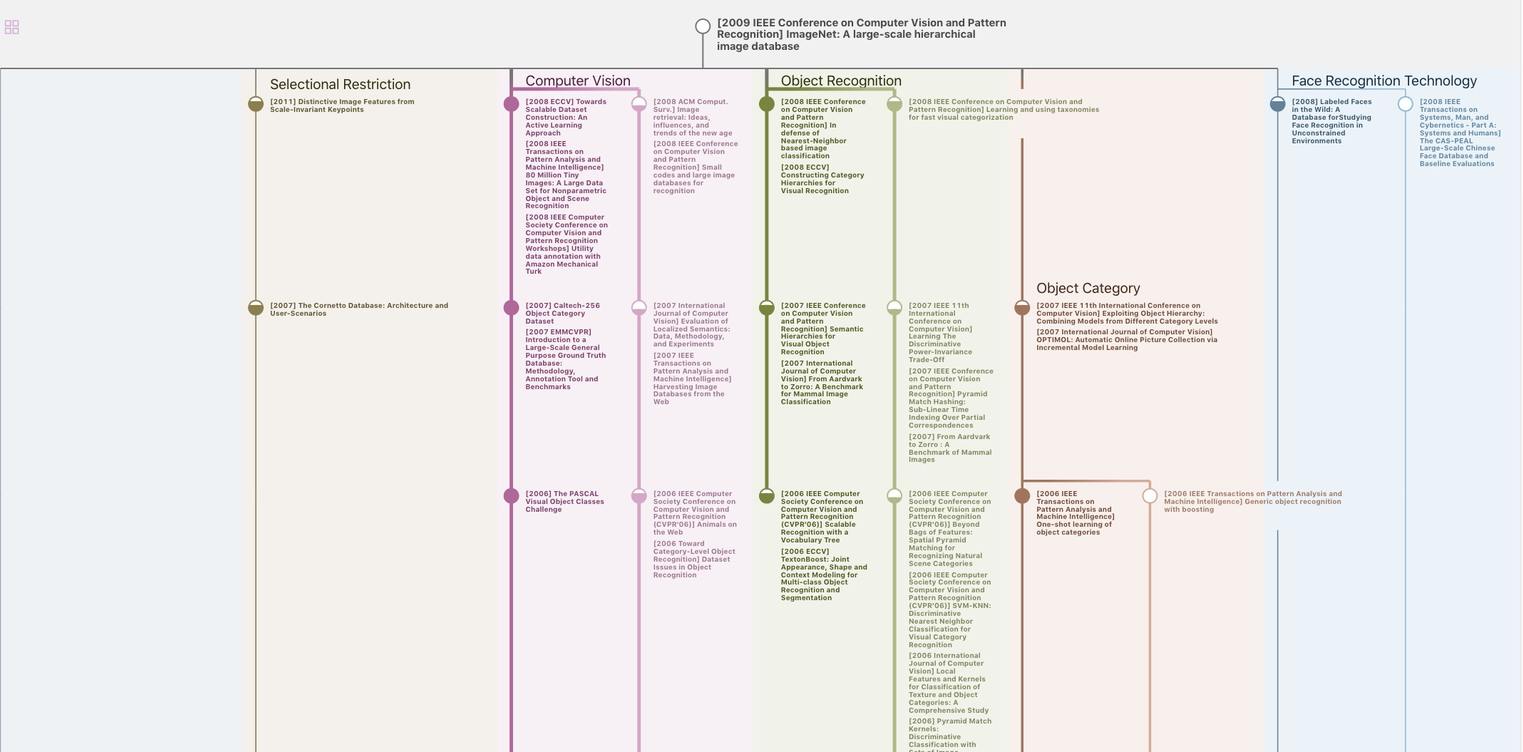
生成溯源树,研究论文发展脉络
Chat Paper
正在生成论文摘要